Brand vs. Performance marketing has been a growing battle online since digital advertising grew to be the dominant share of ad spend. Our belief at Recast is that it’s not Brand versus Performance, but that it’s more of a Brand-Performance spectrum, with some channels and campaigns having a longer term effect, and others getting quicker results in the short term. We believe short term should be predictive of the long term, so an important part of the job is looking for short term signals that indicate long term impact on brand performance.
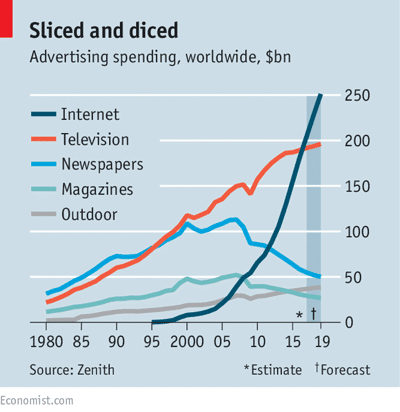
Indeed even the categorization of digital channels as short term Performance marketing, and traditional media channels as Brand marketing, is an anachronism. People watch YouTube on their TV, listen to Radio on their iPhones, and read Newspapers on their iPads. What makes more sense as a categorization now is whether the objective of the campaign is short term, medium, or long term sales. For example a digital channel like Google Ads might be short term, but so might Newspaper classified ads. Display advertising on a premium website likely behaves similarly to premium placements in a magazine, in terms of being more memorable than a Google search, but not as memorable as a TV ad.
The difficulty comes when we try to measure the long-term effect of campaigns. We believe that at least some brand campaigns have an effect over and above the immediate performance we see over the few weeks after they run. The issue is that we can’t just take this on faith: we need to know how much of an impact they are likely to have, and over what time frame the payback period would be. Furthermore, we need some sort of forward-looking model for estimating what the impact of a specific TV is going to be in the future, based on the performance we see now.
This is by no means a solved problem, but in a recent Marketing Mix Modeling summit hosted by Meta, one proposed solution was presented by GfK & Nepa in partnership with Meta, in a session entitled “Measuring long term effects with MMM”. In this post I’ll cover the issues around brand performance measurement and why it has historically been a huge problem. Then I’ll introduce the highlights from the GfK, Nepa, and Meta session, explaining what they did and why it shows promise. Finally I’ll walk through a tutorial to demonstrate how you can use this method in your own MMMs.
Performance Measurement
Measurement of short term channels like Google Search Ads and Newspaper Classifieds is relatively straightforward: you can mostly rely on digital tracking or promo codes. Measuring the incremental contribution to sales of medium-term channels like Meta Ads and Radio is more difficult due to a delayed effect on sales: more visual and emotive creative tends to be more memorable, and therefore only measuring impact within a 1 day window can mean missing most of the benefit. However the modern marketer does have tools for dealing with this problem, for example post-checkout “How did you hear about us?” surveys, Marketing Mix Models (MMMs) with Adstocks (a transformation accounting for the lagged effect of advertising), and conversion lift studies to calibrate the MMMs.
For many smaller brands the correct amount to spend on brand advertising is zero: if you don’t have the large distribution networks of Coca-Cola, or if you don’t have an undifferentiated product like Dishwasher soap that’s bought on a regular basis, you might not need brand advertising at all. Reaching customers who aren’t yet ready to buy is very expensive, and you could go out of business before they eventually remember to buy from you. What’s more, until you establish economies of scale and the ability to maintain quality, growing your market share too aggressively can actually just make you go bankrupt quicker! Before embarking on an exercise to measure the potential impact of brand advertising, first ask yourself if it’s really needed for your company stage.
Brand Measurement
However no consensus has yet been reached on how to measure the long term effects on brand from any marketing channels. When you talk to traditional brand marketers about how they measure success, it’s clear that many don’t. As crazy as it might sound to performance marketers who have to justify every penny, many organizations simply set aside a portion of their revenue for brand marketing.
Many brand-oriented marketing organizations use “brand awareness” surveys as their main KIPs. The way this works is every month or quarter a survey would be conducted which asks “Which of these brands have you heard of?”, and if a higher percentage selects your brand, you get your bonus. There’s no attempt to connect this KPI to sales, and anyway the average CMO tenure is only 3.3 years, so they won’t be around to find out. Measuring the impact of brand investments remains one of the top challenges brand marketers have to deal with.
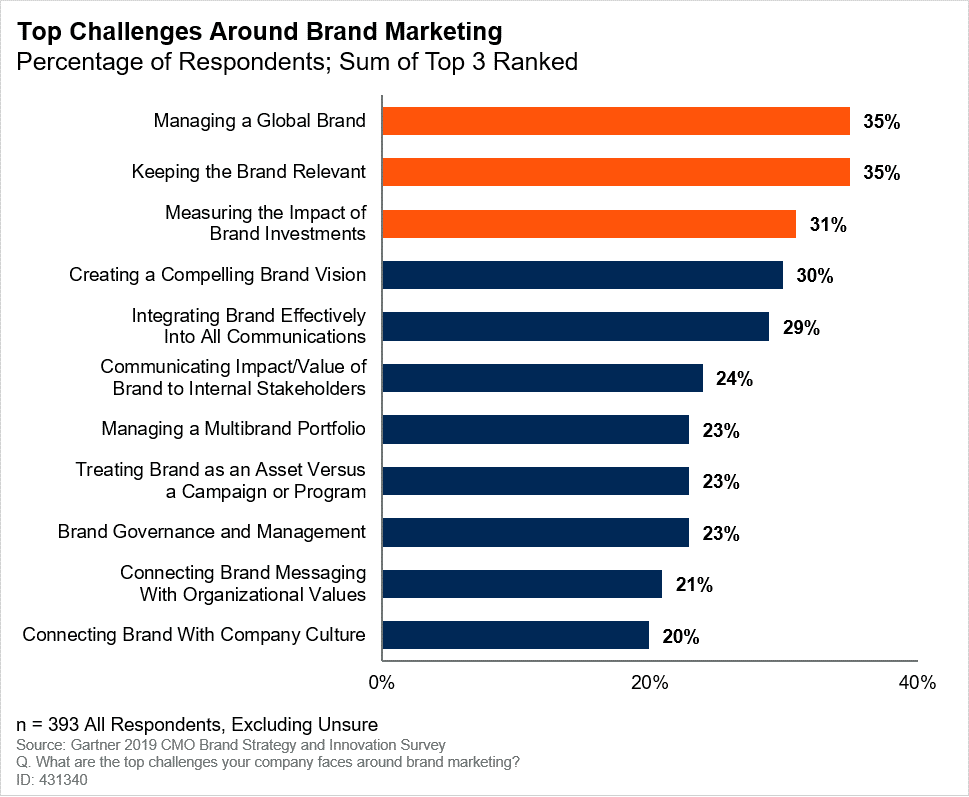
Everybody holds up their hands at this status quo, partly because the accounting department sees marketing as a ‘fluffy’ discipline anyway. This tenuous situation, and growing upwards pressure from the rise of performance marketing, means sometimes you’ll get traditional marketers angrily quoting the work of Binet & Field at you, as if you are questioning some holy religion. They’ll proclaim that the ratio of Brand advertising to activation (what they call performance marketing) should be 60:40, as if it was some sort of universal law (it’s not).
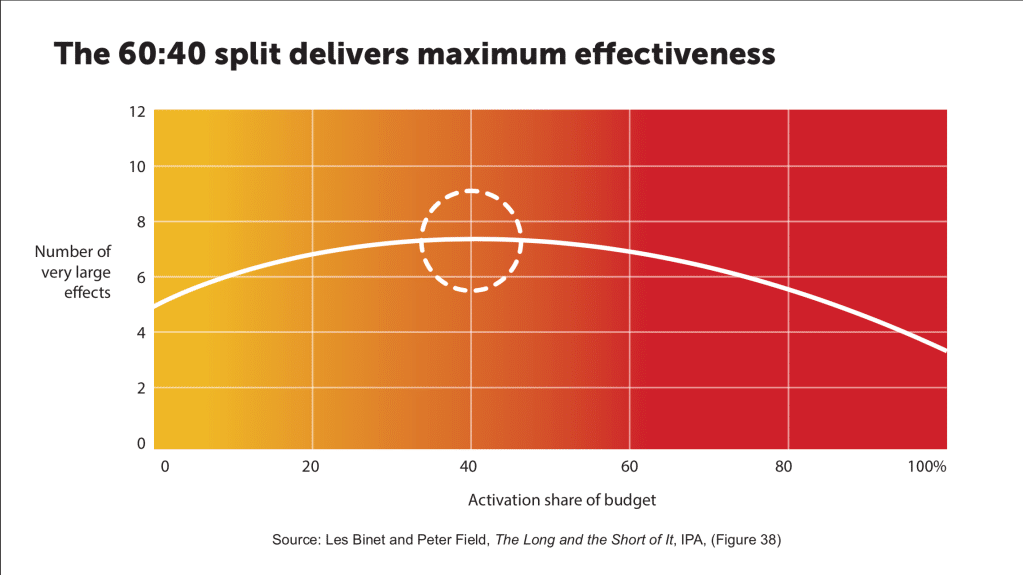
To be fair to brand marketers when you have exhausted your in-market segment, the customers you’re fighting for are the ones who won’t be in market for a long time, so just focusing on making good creative is probably the right focus for the average brand marketer. Afterall, if you get a really big creative win, it’ll be extremely obvious in the data, which is why I always advise to take bigger bets rather than lose sight of what matters trying to discover some more accurate measurement technique.
Adstocks in MMM
While this is not necessarily reflective of how Recast approaches long-term effects (the actual model specification can be found in the technical model specification), the standard solution to accounting for the lagged effect of advertising is using ‘Adstock’ transformations in your Marketing Mix Model.
With this method each channel has a different half life, that you have to find during the modeling process. In our experience the adstock method can identify signals out to about 3 months, but generally not longer than that. So for example a TV campaign might have a long Adstock of 0.9, where 90% of the effect from week 1 spills over into week 2, and so on, but this still drops off over the course of several weeks. Or you might look at Google Ads which might have almost no Adstock, because all the sales it was responsible for occurred in the same week.
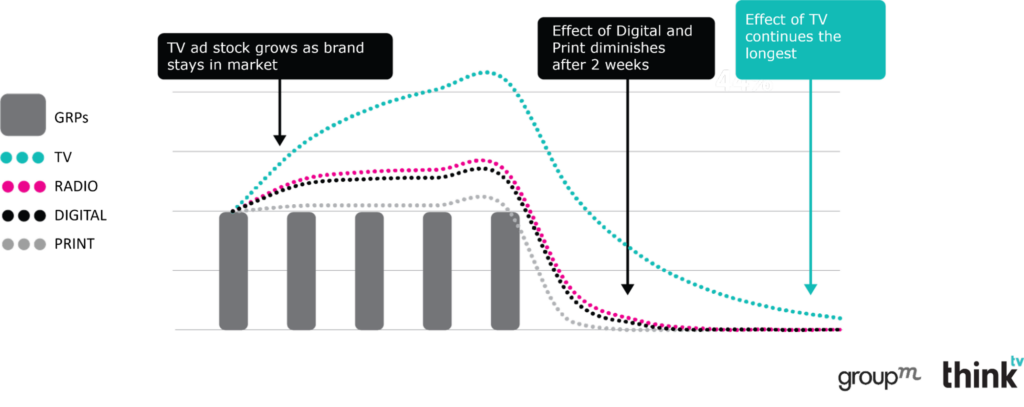
However well this works for short and medium term effects, this causes a problem in my experience for long term effects. When you assume a sufficiently long Adstock, it can become correlated with baseline (AKA “organic”) sales, i.e. it steals credit for sales you would have gotten anyway with no media investment. In private I’ve heard from multiple brands concerned about this, but who are unable to speak publicly about it because of the internal politics around budget allocation. If your Marketing Mix Model is commissioned by the brand team, and the long Adstock assumption in your model causes your Brand TV campaign to look like it’s performing well, it’s unlikely that anyone can challenge it. As Upton Sinclair said: “It is difficult to get a man to understand something when his salary depends upon his not understanding it.”
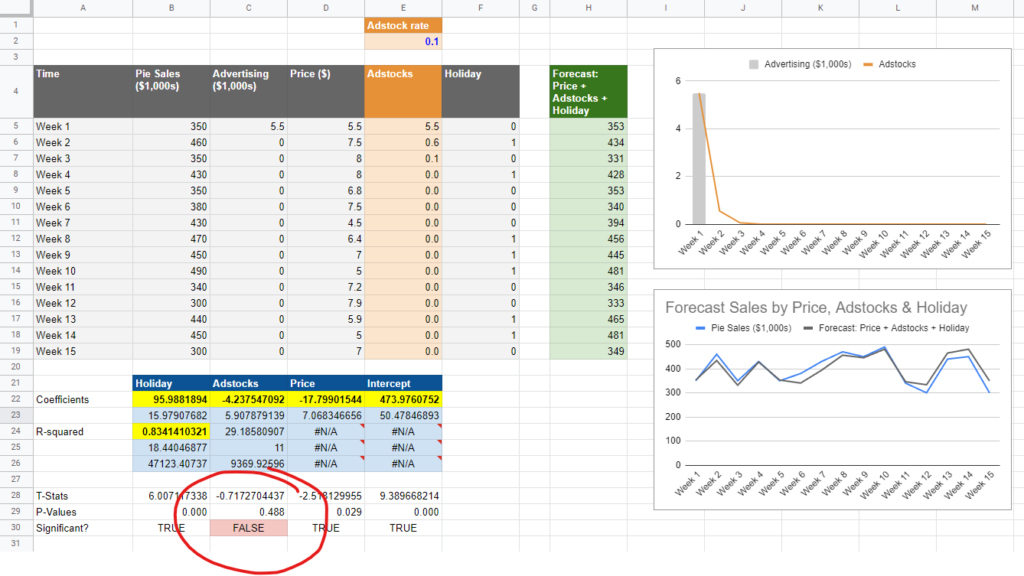
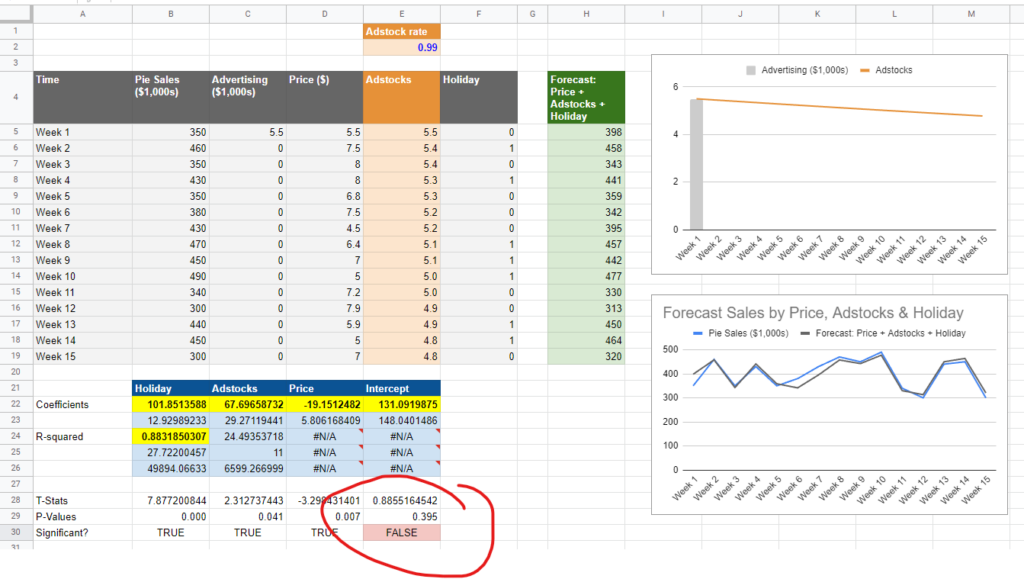
What’s going on in the above screenshots is that we took the exact same model, and changed the Adstock rate from 0.1 to 0.99, i.e. from 10% carryover to 99%. As you can see the higher Adstock makes the chart in the top right go almost flat: spend from one period continues on linearly. The result is that the adstock variable starts to correlate with the constant (which is perfectly linear, as every cell is equal to 1). When this happens, the adstocks ‘push out’ the constant term (called the ‘Intercept’ in the screenshot), which becomes statistically insignificant. The adstock variable essentially steals some of the credit from baseline (AKA Organic) sales, getting more credit than it’s worth. This can be a really big deal! If you overestimate the impact of your brand marketing, you’ll assign too much budget to it, wasting your investment.
Measuring Long Term Effects with MMM
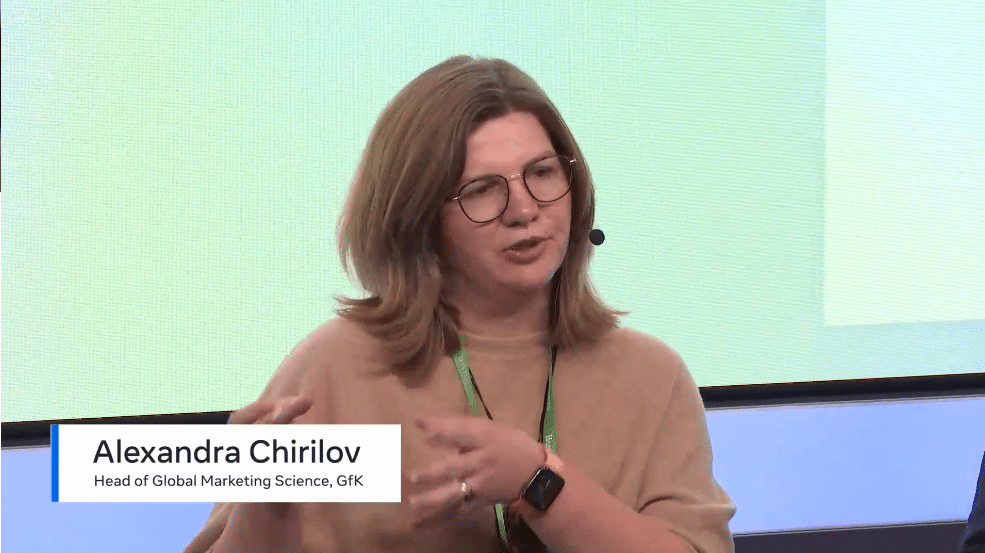
Now let’s discuss what was covered in the session by GfK, Nepa, and Meta (again, this is not Recast’s methodology, but it’s good to get context). The good news is that it’s a relatively easy, elegant, and accessible technique. First the method requires brand tracking survey data that continuously measures brand equity / strength / awareness. This would be the aforementioned survey that asks something like “Which of these brands have you heard of?”, from a portion of the population over time, so you can plot trends.
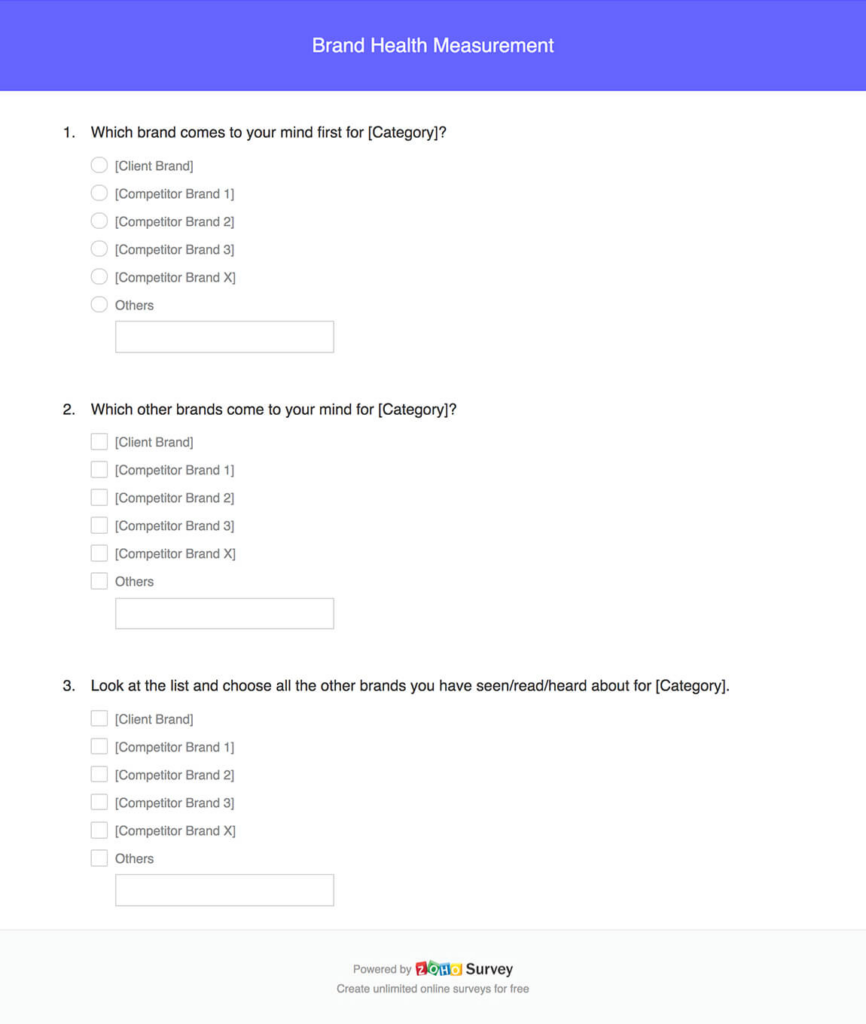
They did mention that you could also use Google search term volume as a decent proxy, which is also echoed in the share of search work done by James Hankins, though it depends on your category and if the keywords are polluted. For example if Recast was doing share of search for Marketing Mix Modeling market, we’d have to tease out people specifically looking for Meta’s Robyn open source library, including those searching under the old Facebook name, separate from those looking for the social network, company news, or the Swedish singer.
Their method is fairly straightforward: they first measure how marketing drives brand awareness, then second how brand drives sales. So they’re describing an embedded or multilevel model, where one model’s output serves as the input for the base sales model. Brand is measured as brand awareness measured by continuous brand tracking surveys, or one of the other brand salience metrics. That way you have a model for how much the typical brand campaign contributes to the top of the funnel, brand awareness, and then how important that level is for its contribution to baseline sales.
A word of caution: as Kalle from Meta warned in the session, when using brand tracking data in MMM it can be very sensitive to sample bias and noise. No matter how accurately you conduct your survey, you’ll still end up with a margin of error. The ‘true’ value of brand awareness might be plus or minus a few points, which means even small swings up or down in your estimates can seriously affect the results of your model downstream. We also still have the same problem we had before regarding the long term effect of a channel being too closely correlated with baseline sales: that didn’t go away. There are noise reduction techniques to clean up the data, and it’s possible to aggregate multiple brand KPIs (awareness, salience, consideration) to reduce variance, though in the session they didn’t give examples of what they used in the study.
Now for some surprising findings from the study. One is that “Short term is not a predictor of the long term” as Alexandra from GfK says. She’s seen campaigns that had no impact in the short term but great impact over the long term, and vice versa. If this is true it would be a big deal! Most assume there must be a short term effect for there to be long term value, which makes sense and has been supported by the data so far. If this is true, then it may not be possible for us to tell in the short term how much impact a brand campaign will have in the long term: there’s no short term indicator that we’re on the right track to pick up on. However due to the magnitude of the implications further testing and validation on this finding is required.
Another interesting insight from the study was that DLMs – Dynamic Linear Models, which we know as Time-varying coefficients – are important because the impact of channel performance changes over the long term. It makes it far more intuitive and plausible than simply regressing long term brand survey data alone, and it explicitly includes the ability to change in the model. DLMs / Time-varying coefficients are a must when we have volatility like COVID, recessions, wars, etc. The assumption that one single coefficient explains channel performance across that 3 year time period (or longer) doesn’t hold anymore.
Another useful question to ask with this type of analysis is “How long is long term?” as Alexandra from GfK put it. She found that some brand campaigns only had an effect of 6 months, which is shorter than she expected. Though she found that many brand campaigns only ran for around 20 days, which is likely too short to have a lasting impact. However it’s also important to consider that this might be correlation not causation, for example maybe poor ads get turned off quicker, or less important campaigns run for less time. It’s also important to account for ad fatigue, as running an ad for too long might hurt performance too.
One final point was that brand doesn’t just impact volume of sales, it also impacts price. “A lot of the long term effect is through price elasticity” says Alexandra from GfK. Essentially the effect of a brand is partially in making customers willing to pay more for the product, and that is part of the long term impact. This is something traditional marketers, with the 4Ps drilled into them, tend to get right more than performance marketers. Ability to raise prices without decreasing sales is an indicator of a strong business, and that’s what investment in brand advertising can give you.
Brand MMM Tutorial
Although the code and data haven’t been released from the session, now that we know the general method has potential, we can try implementing it ourselves. This should be viewed as an advanced technique, because you’re more likely to get it wrong than get it right. The effect of brand is often strongly correlated with other variables in your marketing mix model, so it’s highly likely that you’ll end up with implausible or inaccurate results. This is a particular danger if you’re using standard linear regression rather than something more flexible like Bayesian MMM, which can help you avoid some of the danger by setting realistic priors for each variable.
The exercise for this section comes from a tutorial on Vexpower, entitled “What’s the long term effect on brand?”, which teaches this embedded model technique for incorporating brand spend into your Marketing Mix Model. We won’t run through the entire tutorial here, as it’s relatively technical, but hopefully you’ll gain some intuition for how this technique works, and decide if it’s worth experimenting with.
Generating the Data
We don’t actually know what the ‘truth’ is about performance when building an MMM, because if we knew we wouldn’t have to estimate it with our models. However one technique that can be used to evaluate modeling techniques is to simulate the data used in the model. That way you know what the real truth is, and see if your model picked up on the right answers, as well as where it failed. We previously used this technique – called ‘parameter recovery’ – in our post “How much data do you need for your Marketing Mix Model?”, if you’re interested in learning more about how it works.
First we created normally distributed data with SKLearn’s `make_regression` function, returning the underlying data for our performance marketing spend and its relation to sales, with some statistical noise. We made the assumption that performance would not vary significantly over time, other than moving up and down randomly with noise. The `make_regression` function tells us what the coefficient for this channel is, which we can use later to check the accuracy of our model. In this case we scaled the values (keeping the shape the same) to approximately $210 per day in ad spend and 650 sales per day.
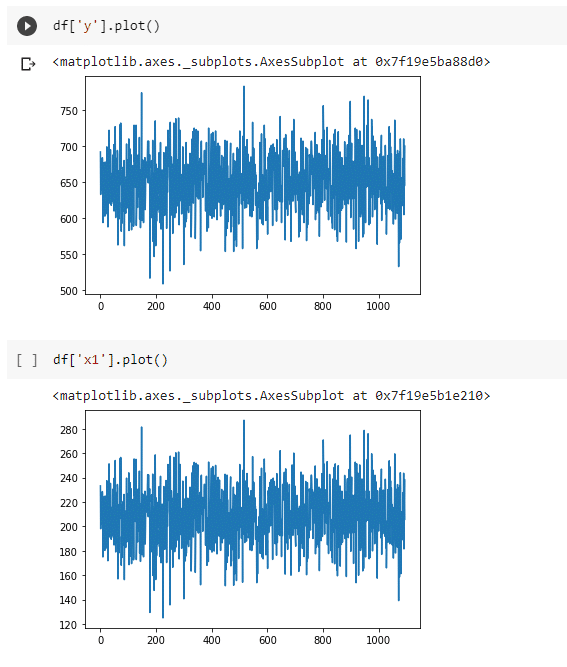
Next we generated the brand campaign spend, which was a sequence of once per year sustained spending for a period of time, with an added adstock (lagged effect) and power (saturation) transformation, so that the impact on brand awareness was felt for a long time, but didn’t accumulate too aggressively during high spending periods. This was multiplied by a general upwards linear trend to get a figure that shows increasing cumulative brand awareness, followed by periods of exponential decline when brand campaigns were off, each time reaching a new plateau.
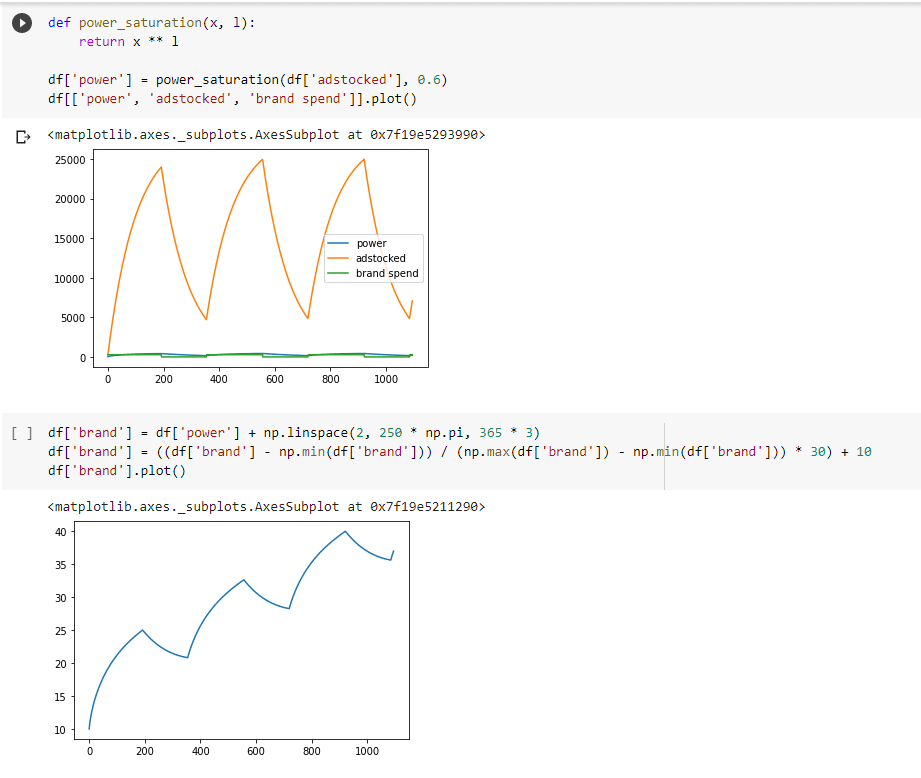
Note that this increase in brand awareness over a short period would be a rare success. We used a dramatic increase just to showcase the example, but typically even a very successful startup might go from 15% to 19% brand awareness. If you’re following along, you’ll realize that makes this type of analysis even harder in practice: with a smaller difference year on year in brand awareness, you’re even less likely to be able to tease out the impact in your model, as it’ll be more highly correlated with baseline sales.
Finally I used an objective function to pull all this together into a sales variable, and noted down the coefficients I used for checking our model accuracy later. There were three major assumptions:
- Brand campaigns drive brand awareness
- Brand awareness drives higher sales
- Brand awareness also has a halo effect on performance campaigns
Incorporating these factors explicitly helps us establish semi-realistic short and long term brand effects to tease out in our model. It’s an incomplete analysis because of course there are many other effects of brand to potentially model, but it’s a simple starting point for showcasing the potential for this model to incorporate long term brand effects, which typically is not done in MMM because of the complexity of measurement.
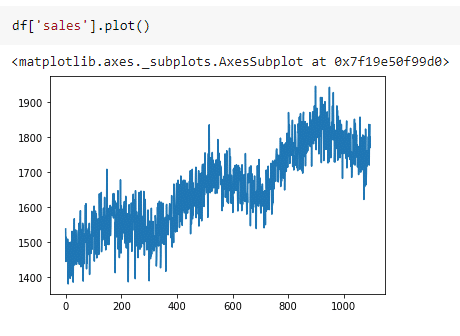
Analysis Findings
First we built a basic model with no embedding, simply a linear regression model using Python’s `statsmodels`. The results that it generated had a good R squared value (which as we know, is worse than useless!), but the channel values are way off. The constant term took up the majority of the sales contribution, leaving both brand awareness and performance marketing undervalued relative to what we know the truth was.
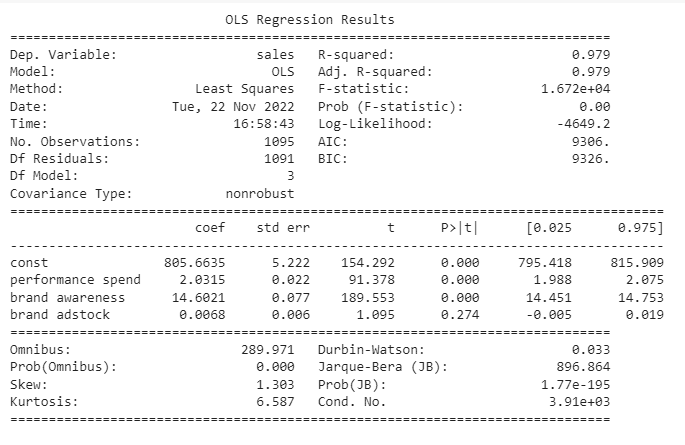
In the tutorial, the next step is then to build an embedded model that predicts brand awareness, not sales. This model takes into account the same drivers, but gives you back an estimated uplift in brand awareness from running branding campaigns. Being able to measure what drives brand awareness will help you forecast it in the future, so you can estimate the probable lifetime impact of running a brand campaign today. This is useful even without incorporating it into your marketing mix model, because it can be used to set targets for branding teams as well as identifying any anomalies above or below trend, for example what the impact might have been from an influencer sharing your product, or a negative PR cycle.
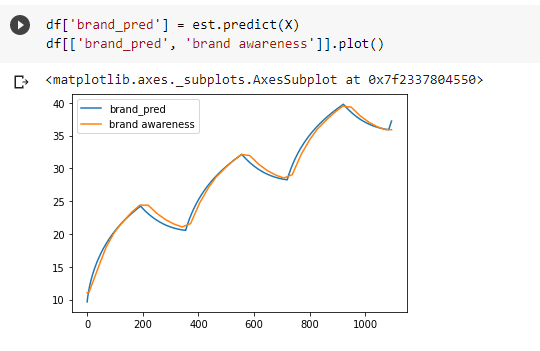
The final model then embeds the brand awareness model inside it, taking the output of brand awareness as an input in trying to predict sales in our base model. The model is much improved with this technique, with the constant taking up less of the sales. However it still struggles with issues: for example it has been estimated that the halo effect of brand on performance (performance x awareness) is negative, when we know it to be positive. Rather than hide this messiness from you like most tutorials, I think it’s important to see just how complicated this type of analysis can be, even for experienced professionals. Tread carefully when attempting to evaluate the impact of long term brand effects: there’s a reason there’s no standard approach!
Simple Model | Embedded Model | Actuals | |
const | 882,202 | 788,232 | 328,500 |
brand awareness | 457,622 | 555,303 | 634,116 |
performance spend | 465,360 | 555,303 | 713,316 |
brand spend | 2,305 | N/A | N/A |
performance x awareness | N/A | -93,865 | 131,557 |
Note this isn’t the only way to replicate the spirit of what Meta, GfK, and Nepa did in their analysis, just the simplest. In production if you were attempting to solve this problem for real I’d recommend something like a Bayesian model which allows multiple hierarchies, sometimes called levels, all estimated as part of the Markov Chain Monte Carlo process. For example, Recast uses a multi-level model to estimate the impact of media channels on brand searches, which is an alternative way to account for the long term effects of brand which accomplishes the same goal we’re trying for here. This was adopted because through significant testing and R&D, we’ve found the modern consumer brands that use Recast benefit most from modeling brand search, and this approach is less likely to make you run into trouble. Bayesian models are more advanced and complicated, so you need to work with a data scientist or vendor that really knows their way around this type of analysis.
Disclaimer: This blog post was written by an external contributor about a speculative method for analyzing long-term effects of changes to brand awareness. The approach laid out in this document is not reflective of how Recast approaches long-term effects. The actual model specification can be found in the technical model specification.