At Recast, we often speak with practitioners who are trying to build their own MMM in-house. While we think there are great reasons to use a tool like Recast instead of building in-house, many people want to try it themselves before committing to working with a partner.
In this post, we’ll step through a checklist of important things to consider when evaluating an MMM vendor or building your own MMM in-house. It will help make sure that you don’t end up with a misleading and worse-than-useless model.
We also compare each section against some of the leading open-source modeling libraries so you can see the benefits and limitations of each one.
Model Features
Marketing is complex. In order to have a good model, you need to make sure that you’re explicitly capturing the most important features of how marketing operates on eventual customers.
Below, we’ll walk through a checklist template you can use to ensure you’re following MMM best practices. You can also use this as part of your procurement process if you’re working with a vendor for MMM.
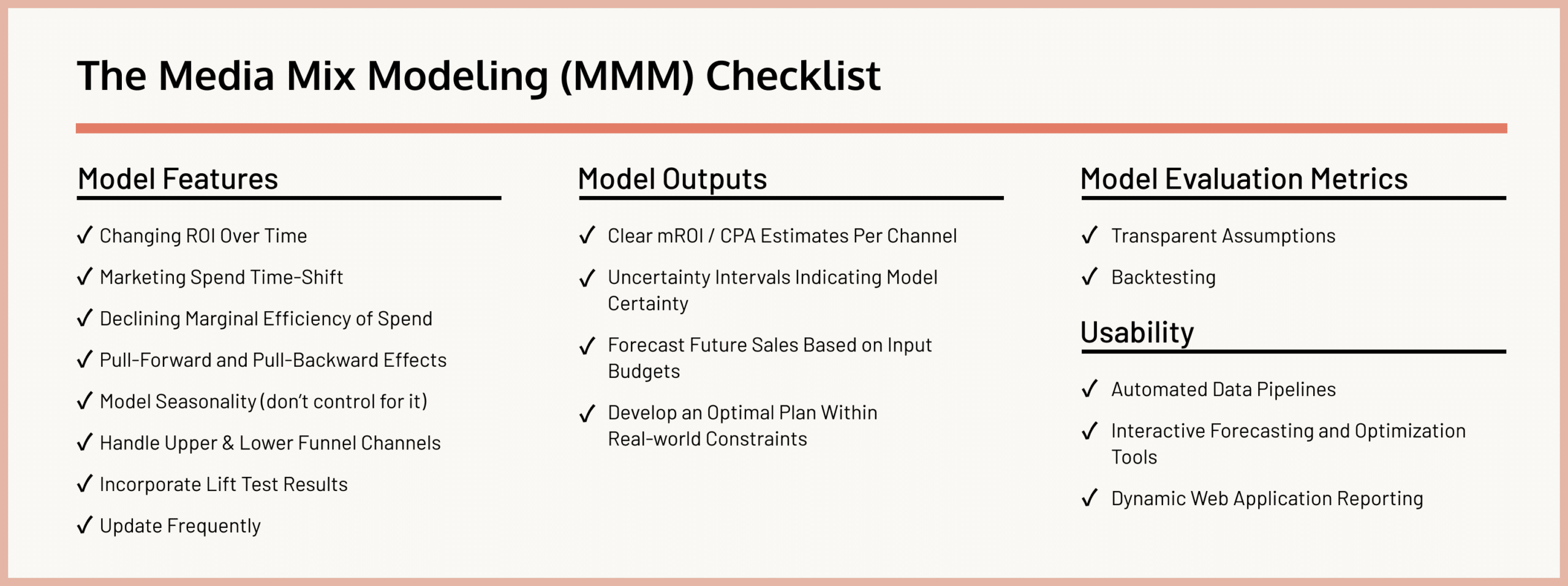
A. Changing ROI over time
This is something every marketer knows, but many modelers fail to appreciate: marketing performance in a given marketing channel changes over time. It’s not uncommon for us to see ROI double (or halve!) in a marketing channel over the course of a month or two. There are many reasons why channel performance can change dramatically:
- The channel changes its algorithm for serving ads (e.g., in response to iOS 14.5)
- You release new creative that performs better than existing creative
- A global pandemic reduces (or boosts!) demand for your product
- A competitor enters the market with a more compelling offer
- A competitor raises prices
- A competitor starts aggressively bidding on your target audience or keywords
- etc.
Failure to allow for marketing performance to change over time can give you faulty results and cause you to make poor decisions when a channel goes from an under- to over-performer (or vice versa).
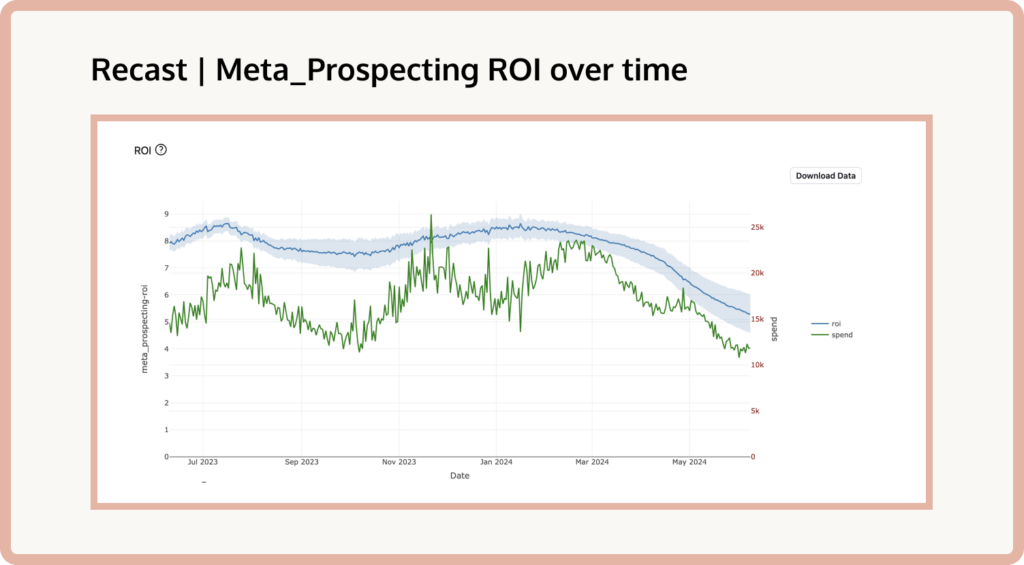
Example: Meta Prospecting performance changing over time in Recast
B. Marketing spend time-shift
If you spend a thousand dollars on a podcast ad today, you will not receive all of the conversions due to that ad today. You probably won’t even receive them all in the next week. Since it takes time for listeners to download a podcast, listen to it, and eventually decide to make a purchase it could take many weeks for your marketing spend to have its full effect.
The amount of time that it takes for your marketing spend to have its full impact will vary (a lot!) by channel, and this can cause tricky modeling problems if your model doesn’t handle this correctly.
Imagine you only spend marketing dollars on TV and search. Your search dollars will probably have all of their effect within a very short time window (say a week), while your TV dollars may take a month or more to have their full effect.
If you run a model looking at spend vs. sales for all of history up until today, you’ll under-credit the impact of TV advertising because your model will not have observed all of the revenue that will be driven by the TV advertising you did yesterday.
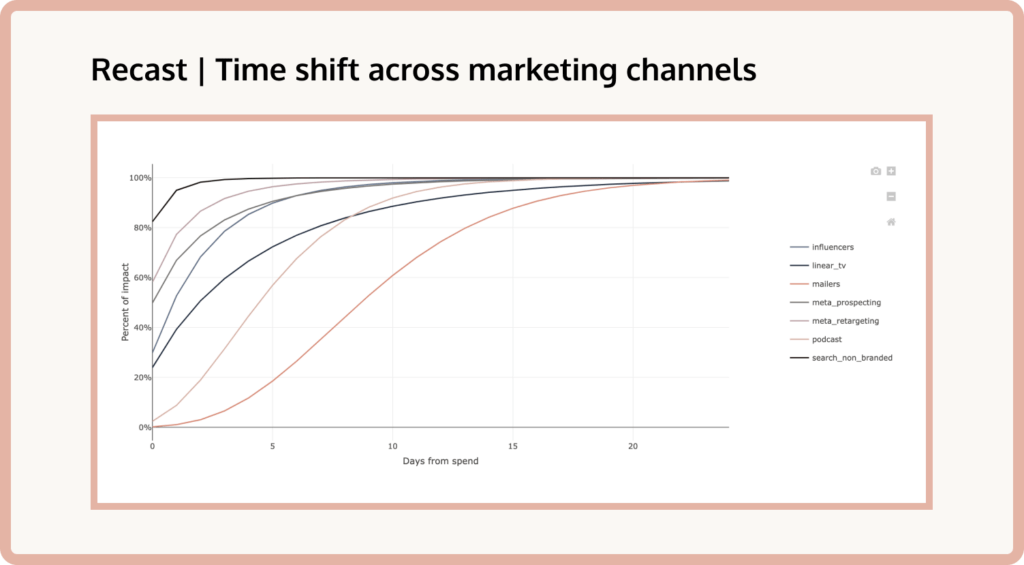
Additionally, it’s impossible to know how long each channel takes to have its full effect – if you’re data scientist is arbitrarily choosing shift effects to apply to marketing dollars (via an “ad stock rate” they pulled out of a hat) then you’re in danger of severely biasing your model.
C. Declining marginal efficiency of spend
Just because you spent $1,000 on Facebook this month and drove a strong ROI doesn’t mean you can spend $1,000,000 on Facebook next month and get the same ROI. In fact, you’ll probably get a much worse return as you scale up your spend. Why? Because there’s declining marginal returns to advertising spend. This happens because Facebook will allocate your first $1,000 to the users that are most likely to convert and buy your product. As you spend more money, Facebook will have to keep expanding its circle of people to advertise to in order to spend those dollars, and by your millionth dollar, you’ll be advertising to people who are much less likely to convert than those targeted with your first thousand dollars.
Different channels will have different amounts of declining marginal effectiveness. Some channels are very effective at small levels of spend, but can’t scale. Other channels may be able to scale but you’ll experience a declining rate of return.
Like with the time-delay effect, the true rates of declining marginal efficiency of spend in a channel are impossible to know, and so you’ll somehow need to model how this impact is affecting the ROIs in your MMM. If you don’t model this correctly, you might end up recommending increasing spend by huge amounts into channels that will not be able to scale, and you’ll waste all of that budget.
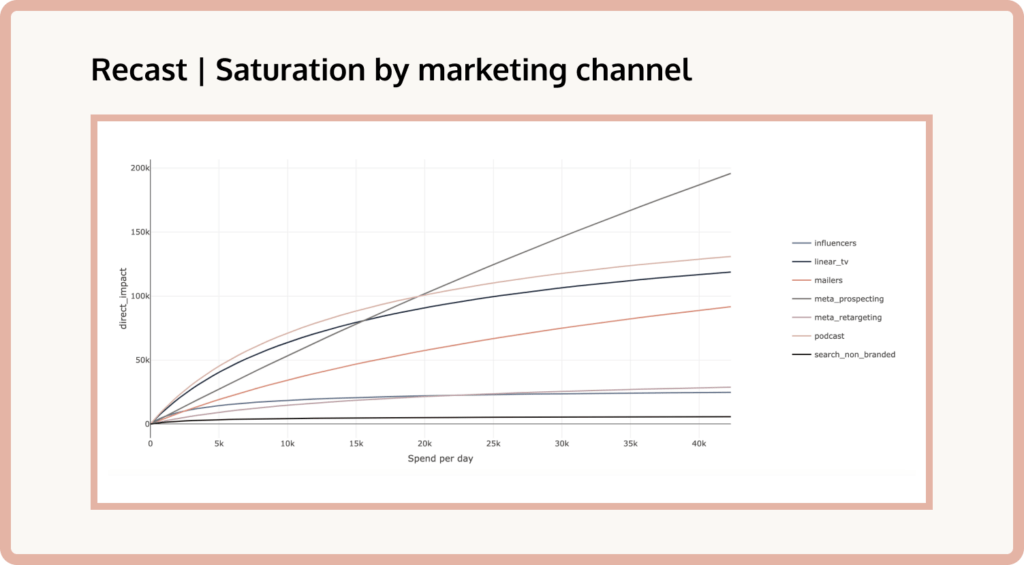
D. Pull-Forward and Pull-Backward effects
Holidays and promotional events are very important for many companies. Handling these events is crucial to correctly estimating the true effectiveness of your marketing dollars.
Imagine this (very common) situation: a big promotional event is coming up, so the marketing team starts increasing spend into top-of-funnel channels in order to put customers into the marketing funnel who can then be converted by the sale. Many customers and potential customers, in anticipation of the sale (if, for example, you do the sale every year) will delay purchases that they were going to make to wait for the sale. And likewise, other customers might “pull forward” a purchase they were going to make in the future in order to take advantage of the sale.
In this case, you need to make sure that you’re correctly accounting for that pull-forward and pull-backwards effect and how that interacts with the marketing spend leading up to the sale. If you simply include a “dummy variable” on the date of the sale, you’ll grossly mischaracterize how promotional events and marketing spend interact.
E. Model seasonality (don’t control for it)
The relationship between marketing and seasonality is devilishly tricky to handle correctly. The issue is that marketers (wisely) spend more marketing dollars when customers are most likely to purchase their products: advertise hot wings before the super bowl, sunscreen in the summer and sweaters in the winter.
However, if you simply “control for” seasonality in an additive model, your MMM is going to tell you that your marketing spend is least effective during those times. That’s because the model will “pull out” the effect of the season as if it were independent of the marketing spend, but really those two things are highly correlated!
This is a common thing that many MMM modelers get wrong, and it leads to nonsensical recommendations to the marketing team (like to only advertise their products when no one wants to purchase them!).
F. Handle upper and lower funnel channels correctly
Many traditional MMM models have implicit assumptions in them that the independent variables are not correlated (for example, anything in the family of linear regressions). Unfortunately, this assumption does not hold at all for marketing spend. Marketing spend tends to be highly correlated over time as brands launch “campaigns” where they’ll increase spend across a subset of channels all at the same time, which in turn impact other variables in the model like brand searches or organic variables. Additionally, many ad campaigns are run during periods of seasonally high demand, which makes this effect even harder to tease out. It’s also problematic to include channels like email, affiliates, and customer referral in the model because activity in those channels is actually caused by activity in other marketing channels.
For example branded search is a channel that is actually caused by other marketing channels. We expect that when brands spend more money on TV advertising they will drive more searches for their brand name and will end up paying the “Google tax” for all of those additional clicks. This relationship can wreak havoc on less sophisticated models since branded search spend will be highly correlated with other top-of-funnel marketing spend and the model will be inclined to over-credit branded search effectiveness. It’s critical to model these relationships explicitly in order to make accurate forecasts and correctly identify which channels are driving incremental impact.
Failing to account for this correlation will lead to severely biased estimates in your model (you’ll end up giving all of the credit to one of the channels that were used in the campaign, or to variables downstream from advertising like brand term searches, email, or affiliates).
G. Incorporate results of lift tests
It’s critical to be able to anchor your model to reality and other gold-standard sources of information about channel performance. If you run a lift-test for your TV program in January, you want to make sure that you can include the results of that test in your model so that 1) the TV estimates are as precise as possible and 2) all of the other channels’ effectiveness can be estimated conditional on the results of the lift test.
H. Update frequently
In order to make the results of the MMM actionable you can’t just update the model once every 3 months. Performance marketers are making budget allocation decisions on a day-by-day and week-by-week basis, so you need to make sure that they have the most up-to-date estimates of channel performance possible. If you’re relying on MMM results 6 months out of date, then you could be wasting hundreds of thousands or millions of dollars.
Model Outputs
I. Clear marginal ROI / CPA estimates per channel
Marketers care about marginal ROI and CPA because that tells them where they can invest their “next dollar” most effectively. The primary output of your MMM should be a clear communication of the current incremental ROI and CPA of the marketing channels your business is active in so that marketers can make effective budget allocation decisions.
Sometimes modelers get so caught up in all of the bells and whistles of their model that they forget that at the end of the day the job of the model is to produce insights that are actionable for marketers.
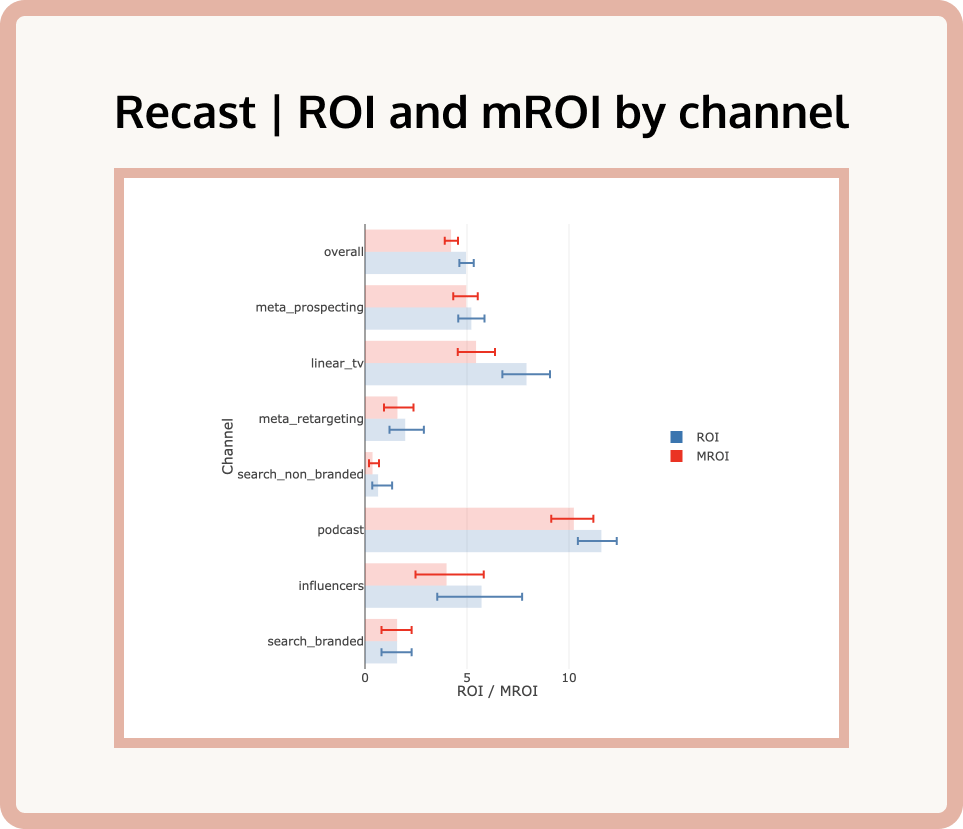
J. Uncertainty intervals indicating model certainty
Correctly communicating uncertainty is critical to a good MMM. For every estimate that the model produces, the point estimate should be accompanied by a confidence or credible interval expressing the amount of uncertainty in the estimate. In general (though not always!), channels with more spend will have more certainty associated with their ROI estimates, and channels with less spend will have less certainty.
It’s important to communicate this uncertainty to marketers so that they can make effective decisions about where to invest their dollars (and how big of a risk they might be taking). We think a Bayesian framework is really useful for MMM because it naturally generates these intervals for all of the parameters in the model.
K. Forecast future sales based on input budgets
While predictive accuracy isn’t a sufficient condition for a good MMM, it is a necessary one. So, a good MMM should be able to predict how much revenue or customer acquisitions will be produced by a given marketing budget into the future. This is really useful for budgeting and planning processes (the finance team will love it!) and can help marketers adjust if they’re not on pace to hit their targets.
These forecasts of course should come with credible intervals expressing the uncertainty in the forecast based on the inputs!
L. Develop an optimal plan within real-world constraints
And finally, the model should be able to produce optimized budgets subject to realistic complex constraints. Marketers often have constraints like “we want to spend as much as we can up to a blended CPA of $73 but we’ve already locked in TV spend for the quarter at $1.5m”.
A good MMM should be able to produce a realistic optimal budget based on those constraints that marketers can use for planning.
Model Evaluation Metrics
M. Transparent assumptions
MMMs should be glass boxes, not black boxes. Because these types of models are complex, many vendors or platforms will try to hide all of the complexity within a black box and say “just trust us, we’re experts”.
This is problematic because the complexity allows them to put their thumb on the scale or even simply make mistakes that generate results that are just plain wrong.
To combat this, it’s important to validate that all of the assumptions the model is making are transparent and reviewable. Every model makes assumptions, from model structure to hyper-parameters for the model. You want to be able to review these hyper-parameters! (Note that hyper-parameters are known as “priors” in a Bayesian framework).
The best modeling frameworks provide extensive documentation on the modeling assumptions, modeling process, and hyper-parameter settings so that you can review and check all of the assumptions.
N. Backtesting
The next check you should make when evaluating an MMM is its out-of-sample predictive fit. That is, you want to check that the model does a good job of predicting sales or customer acquisition even on data that the model hasn’t seen before. If you’re evaluating an MMM vendor, you absolutely must ask for this.
The best modeling platforms have out-of-sample predictive checks built into the modeling process so you can constantly monitor this.
Often, modelers will rely on in-sample fit statistics when checking their model. This is problematic because MMMs are subject to “over-fitting” where the model is too fine-tuned to the data that it’s seeing that it actually can’t predict on new data at all. This is very bad! R-squared in the case of MMMs is a meaningless metric. Any modeler that tries to convince you that their model is good because it has a high R-squared does not know what they’re doing and should be avoided!
We hope this checklist is helpful as you evaluate new MMM projects, and if you’d like to learn a little more about Recast, we’d love to talk.