The team at Meta did another Marketing Mix Modeling summit last week, and given the popularity of the last one we covered, we thought we’d do it again.
This summit covered a lot of familiar themes – e.g. the loss of tracking from iOS14 – but with a particular focus on the mobile gaming industry. That made this uniquely interesting and more tactical, because nobody got hit by the loss of tracking more than gaming apps, and they’re also known for their advanced data-driven approaches to marketing. The overriding theme was again how MMM combined with Lift and Geo tests can combine with attribution to fix the $10b hole in Meta’s balance sheet and make our ads work again.
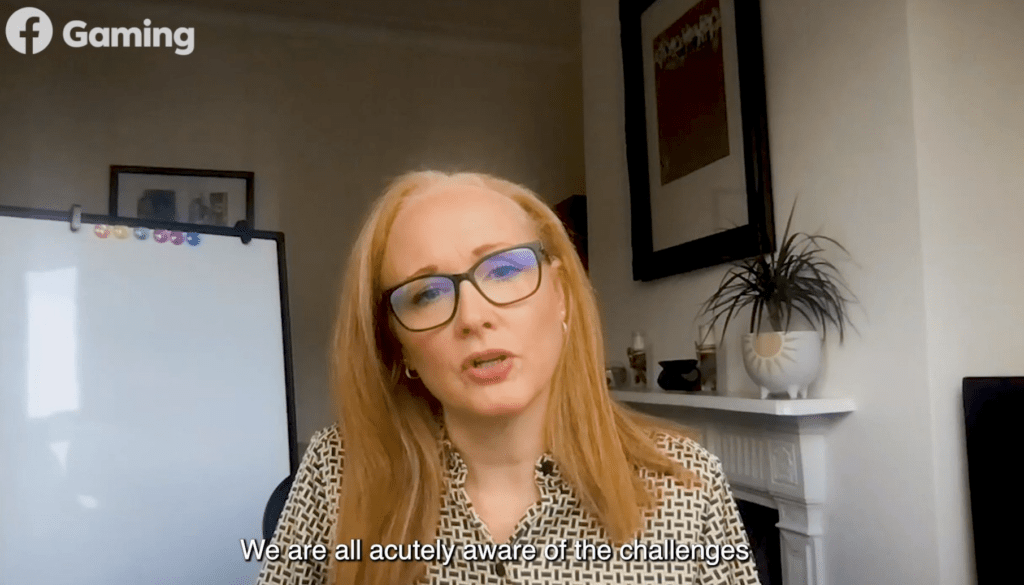
The agenda covered incrementality, their MMM partner program, and how to operationalize MMM within your organization. They mostly gave the floor to partners such as Analytic Edge, Singular, and MetricWorks, as well as hosting a panel discussion by AppsFlyer, Adjust, Pecan who are incubating Meta’s open source project Robyn in their products. All of the panels were interesting, and while they have shared the videos on the event page, I thought it’d be more convenient to be able to browse through a few highlights.
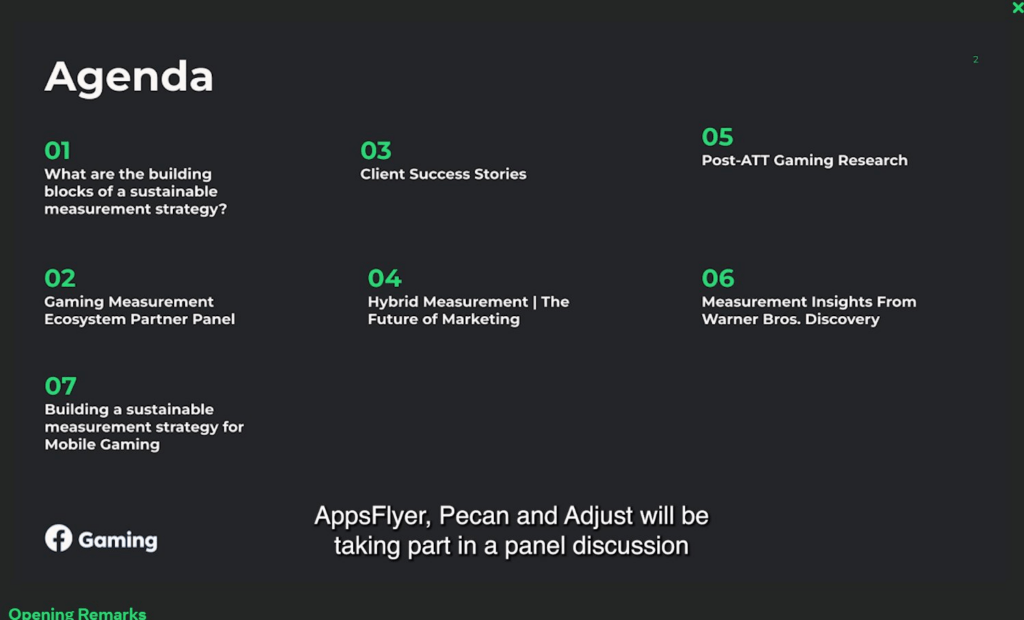
How Existing Gaming Partners Complement Their Current Offerings With MMM
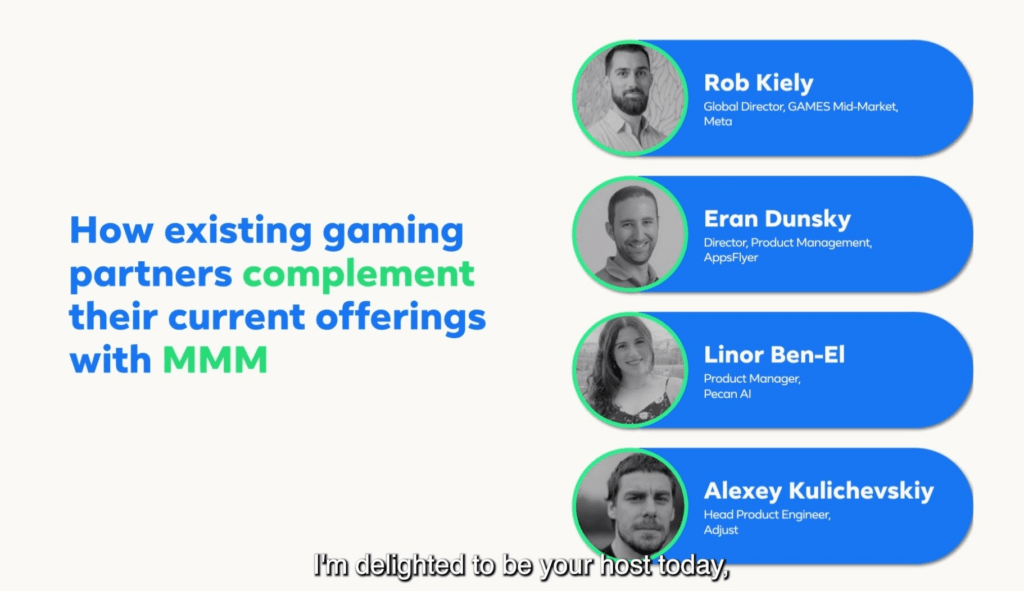
First session was from Meta’s MMM tech partners. More or less the same companies as featured in their annual summit, but different representatives. What the host opened with is that we’ve lost a lot of tracking data with the user privacy backlash, and people are trying out MMM to solve it, but it’s expensive and takes too much time.
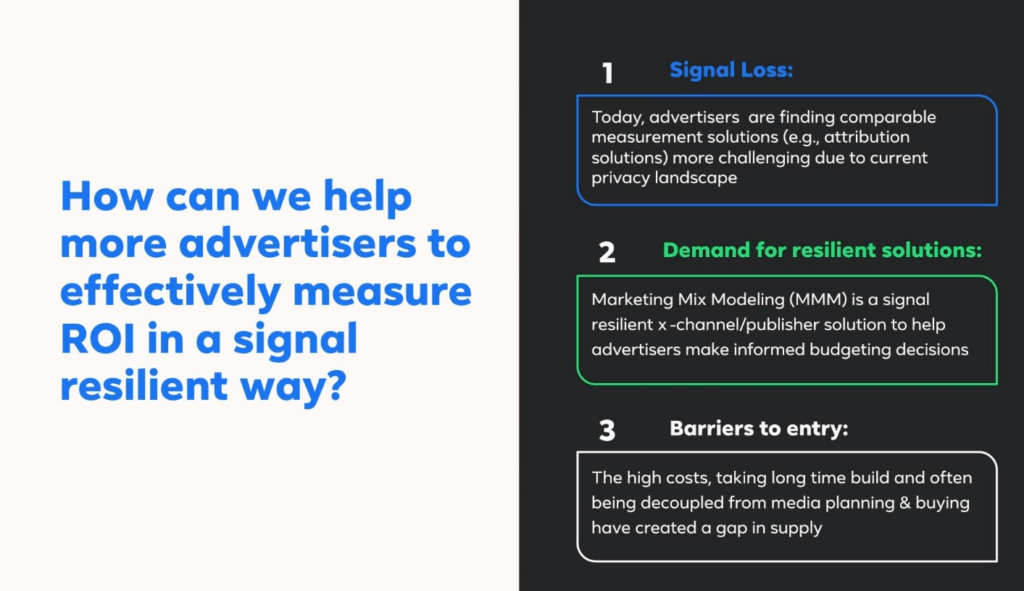
One of the most interesting pieces of data from the whole event: 44% of advertisers reported shifting to MMM as the number 1 solution for loss of tracking data from iOS14. Now we’re not sure how many advertisers that represents, or what size / sector, but it’s a remarkable statistic. Also interesting to see advertisers rely more on branding and creative testing as the other solution out of tracking hell.
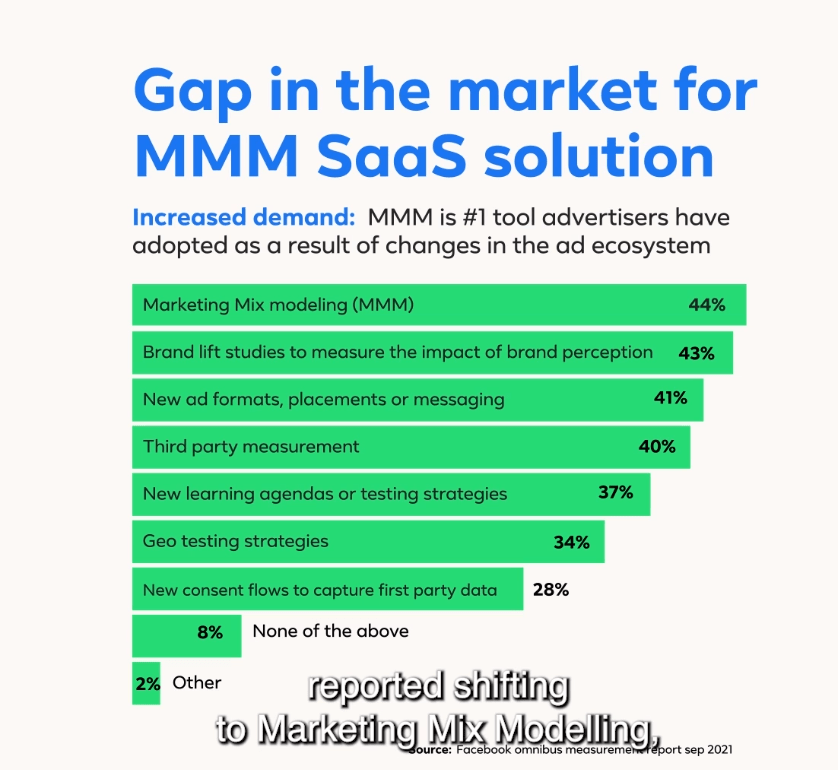
There were no big surprises in the best practices offered, at least for those who have been paying attention. Basically in 2022 your MMM needs to be:
1/ cheaper
2/ done automatically not annually
3/ adaptive to changing performance
4/ calibrated by experiments
5/ actionable by non-technical teams
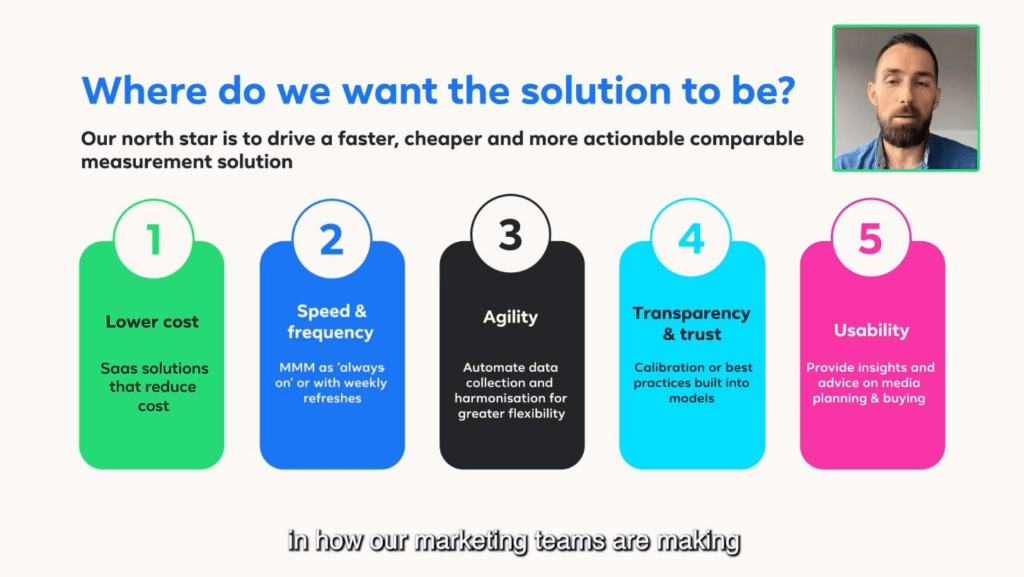
This is what the companies in their incubator had to agree to. Much more relaxed than previous iterations (I’ve heard horror stories of past Meta programs).
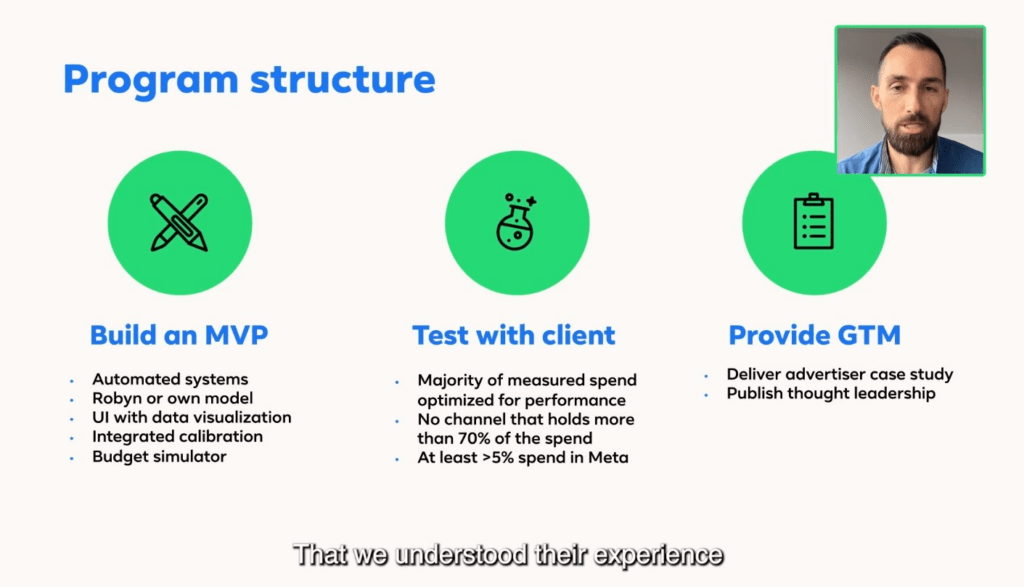
Great point from Linor from Pecan: “Putting privacy issues aside, attribution alone was never perfect”. It’s important context that iOS14 certainly woke us up, but attribution was already broken! The case for MMM SaaS according to Alexey from Adjust is that not every company has the internal expertise to successfully deploy an MMM, as it’s relatively sophisticated.
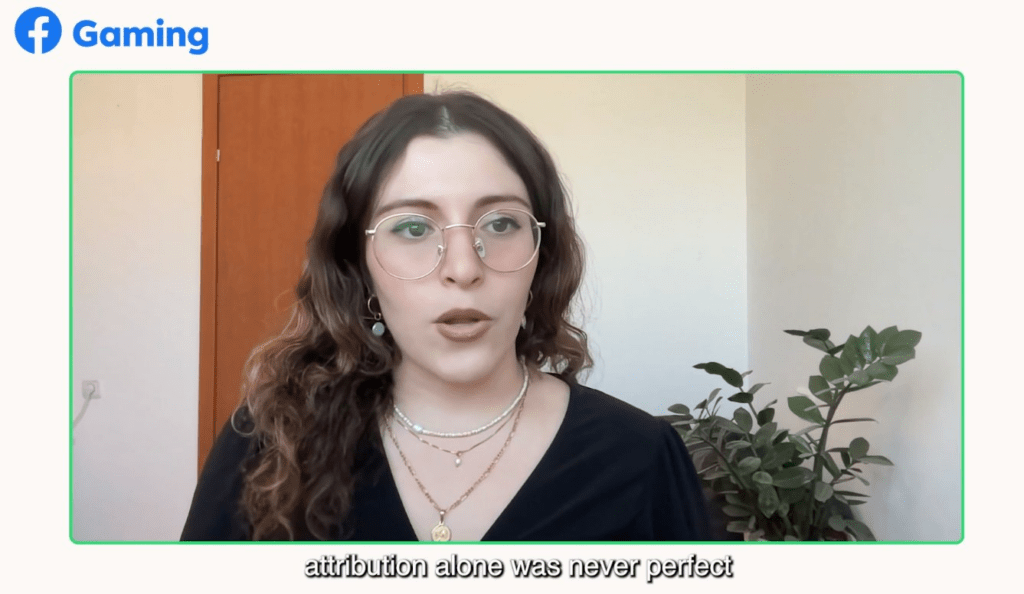
MMM is a model, and therefore can reinforce existing hypotheses that you have, but it can also help you formulate new hypotheses, as Eran from AppsFlyer puts it. The key is trying to remove human bias from the inputs, but use the outputs to formulate new ideas to test.
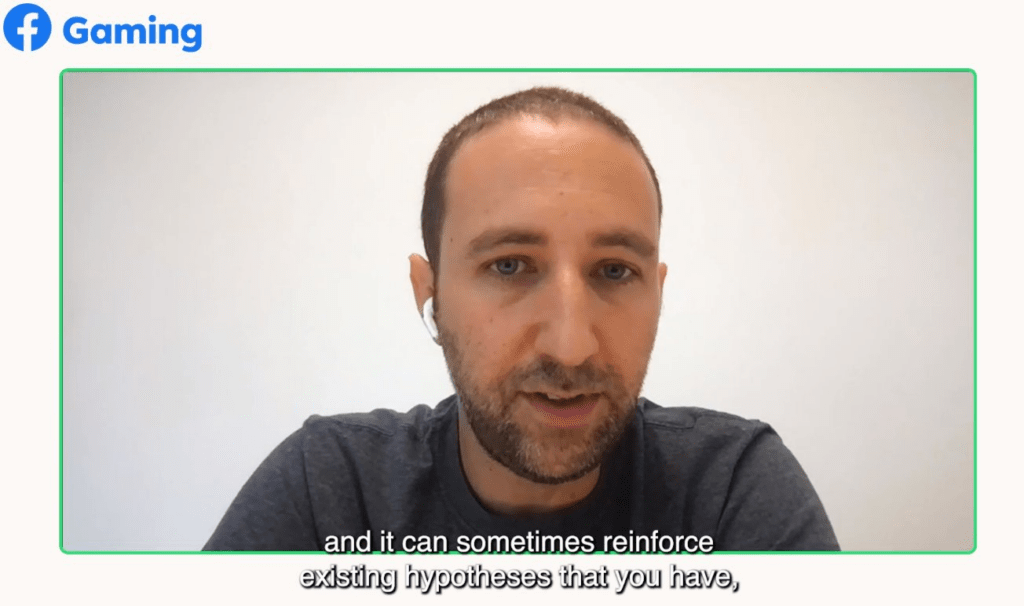
Alexey from Branch says that modeling is a process: it’s not enough to train a model and show nice diagrams. The model value only increases when you test, learn, and iterate on it. This is what I’m seeing with my own work: it’s the aftercare that’s important, after the model is ‘done’.
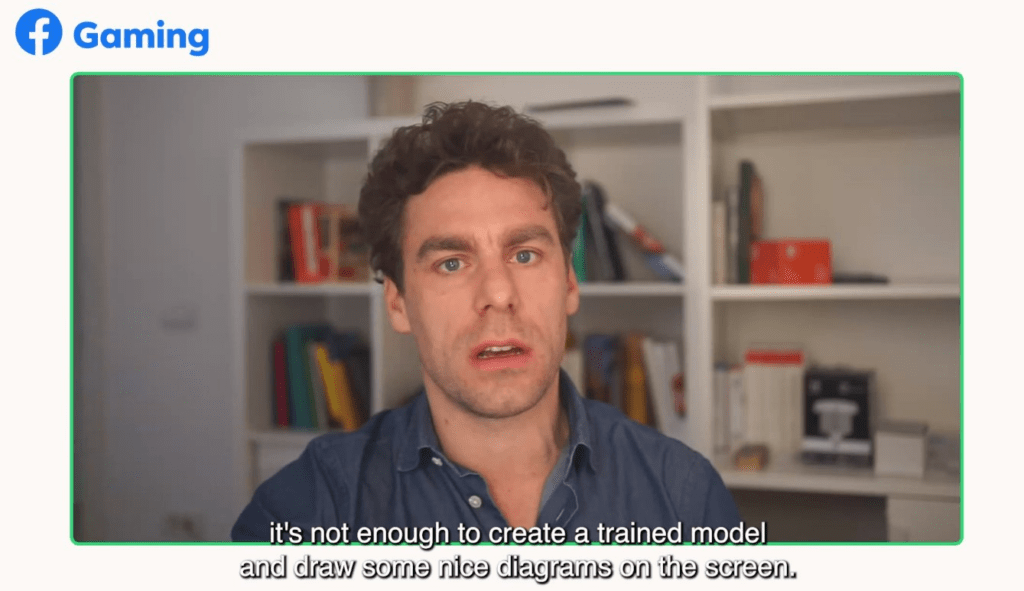
“The key to a successful project is to make a model at the right level of granularity” – Linor from Pecan. That’s true but in my experience it’s very hard to get reliable granularity out of MMM… it’s rare that anyone shares practical tips for getting that granularity, which shows me it’s still not a solved problem. Simulation is the key to actionability in MMM: what would happen if I switched $1m from one channel to another? That’s the actual goal of MMM past all the statistics.
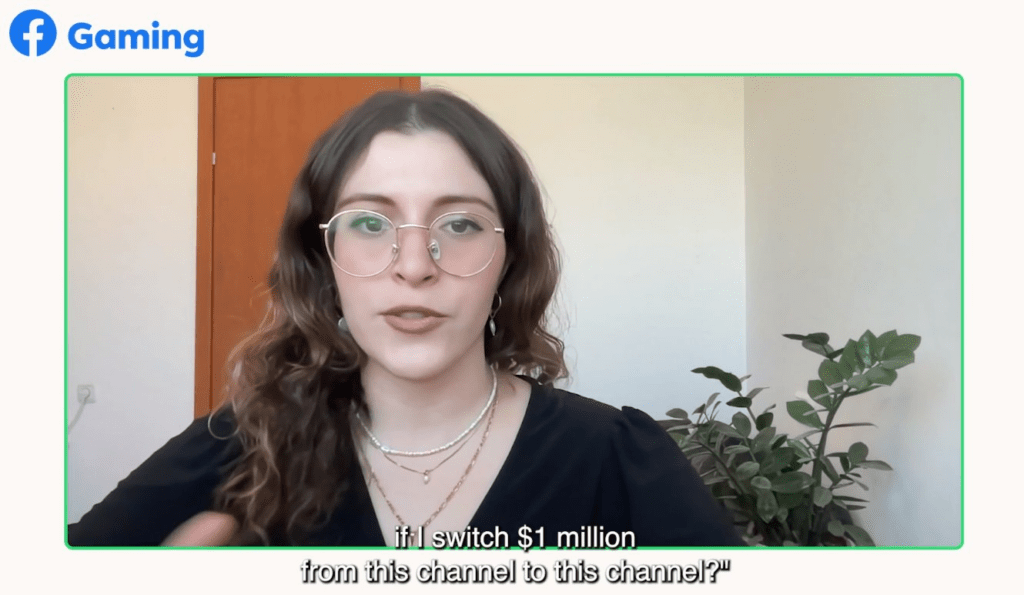
Now some case studies: Branch had a big win from MMM as one of their clients was spending 11% of their budget, “a huge amount”, and MMM had shown it to have zero incrementality. The client validated the result. That’s a huge amount of money saved. Note that sometimes a ‘zero’ result is suspicious and indicates the model has done a bad job. Always test unusual findings.
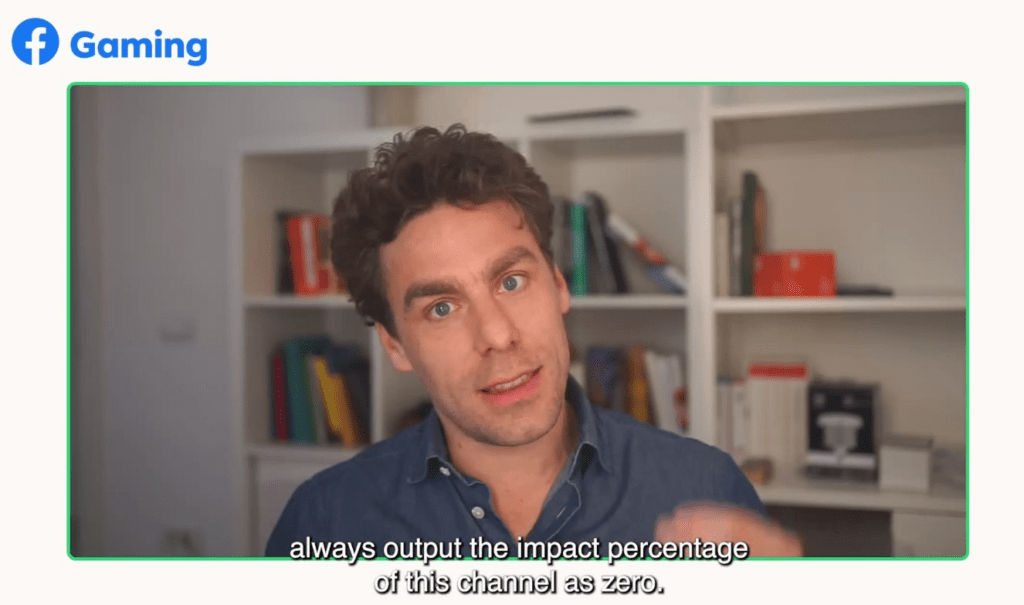
Linor from Pecan tells the opposite story: MMM told a client that some of his channels had a huge opportunity for more budget, which is something normal attribution can’t tell you. She also used MMM to identify a channel that was defrauding one of her clients! This is a story with a happy ending: they got the money back. 🙂
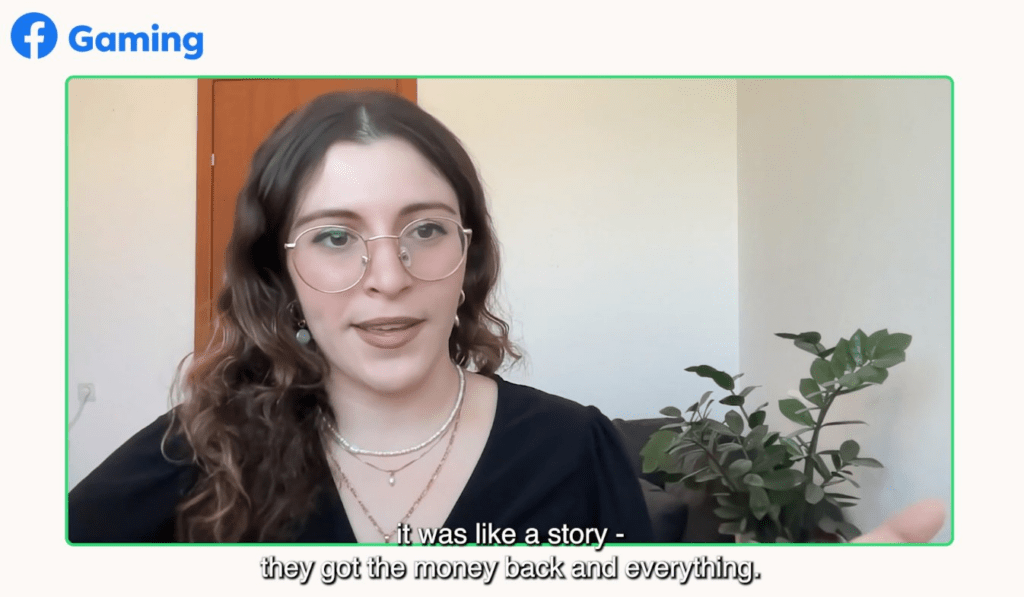
There was a key operational insight from Eran of AppsFlyer: for one client they had unexplained bi-weekly spikes in revenue that created noise in the model. The client confirmed these were in-app promotions, which when controlled for, improved model accuracy. This is an important and difficult part of the modeling process, but if you do it right, you can get much better results.
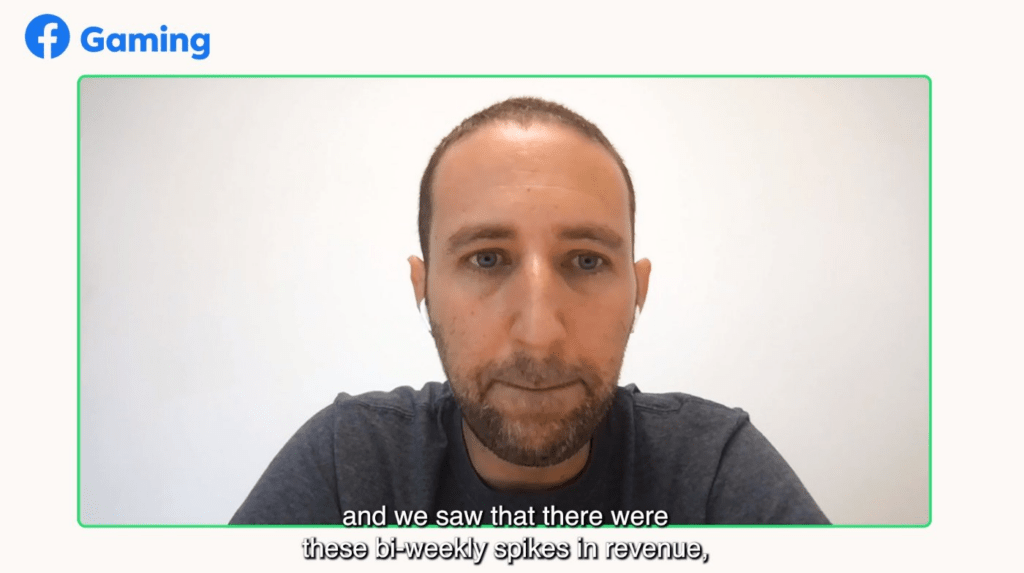
Demand for better measurement solutions isn’t the blocker for new MMM solutions that’s all the teams want! It’s the (justified) skepticism of savvy data-driven marketing teams as to whether a model is giving accurate results that can be relied upon. They need to know the model is going to be useful before they bet millions on it.
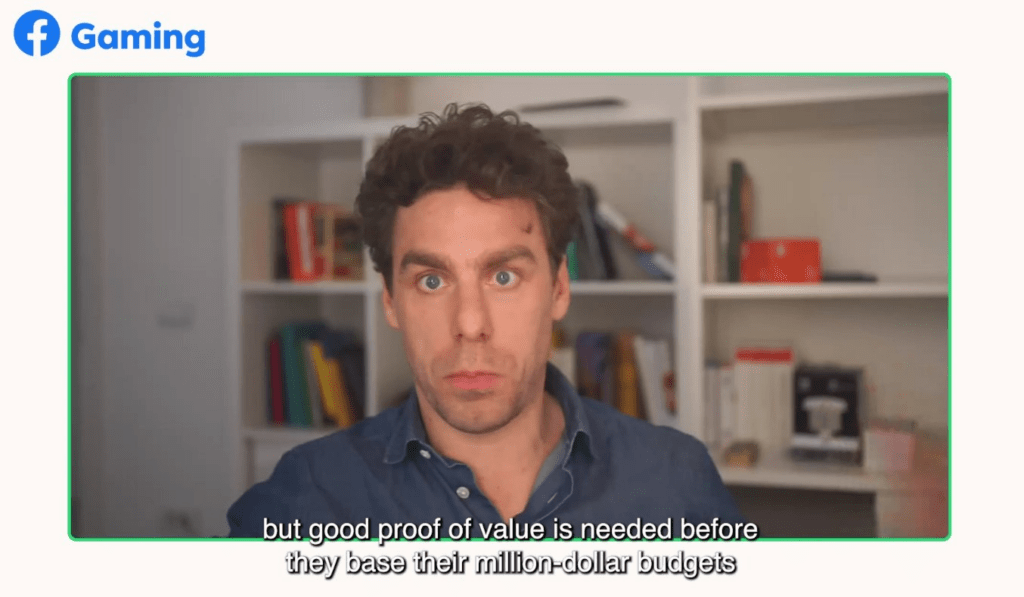
Linor from Pecan is cautioning that even though Apple was the first to take a swing at user tracking, they won’t be the last. You need to get on board the MMM train now in order to benefit from it in the future.
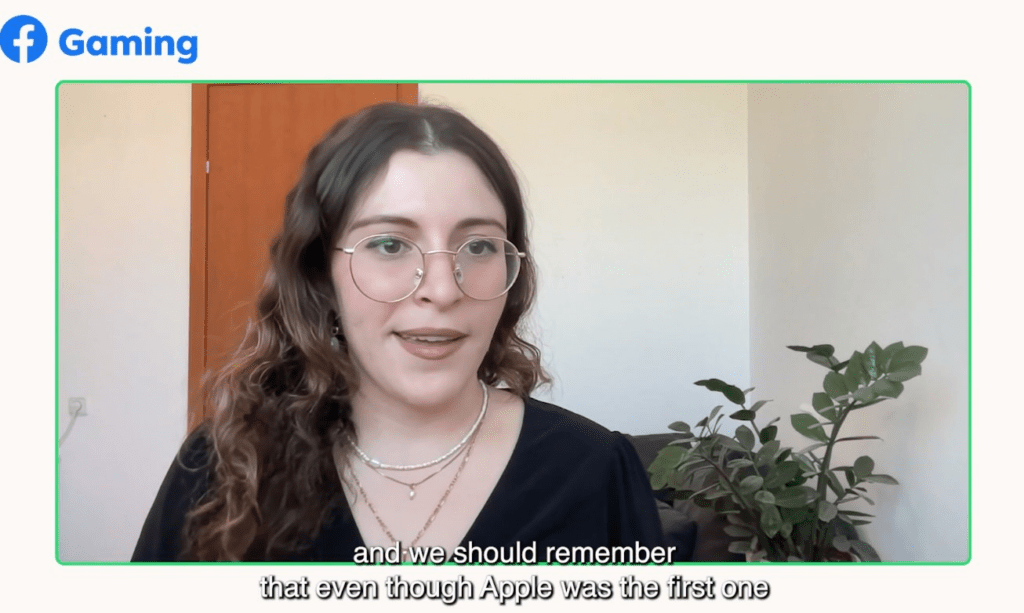
Why Hybrid Measurement Is The Future Of Marketing
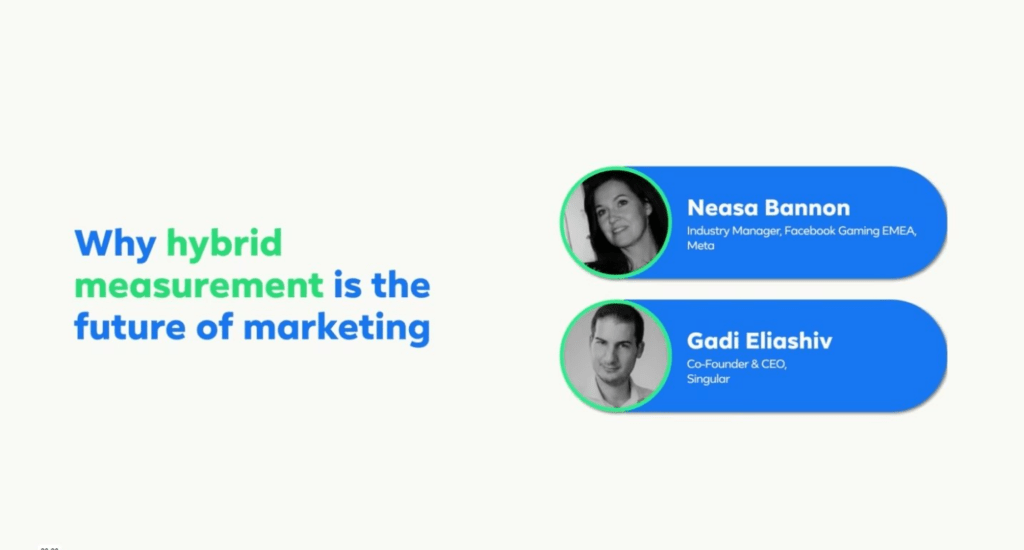
The next session is Neasa from Meta and Gadi from Singular. Hybrid attribution is most certainly the future of marketing in my opinion: you need to triangulate from multiple angles to find the ground truth now, you can’t rely on only one method.
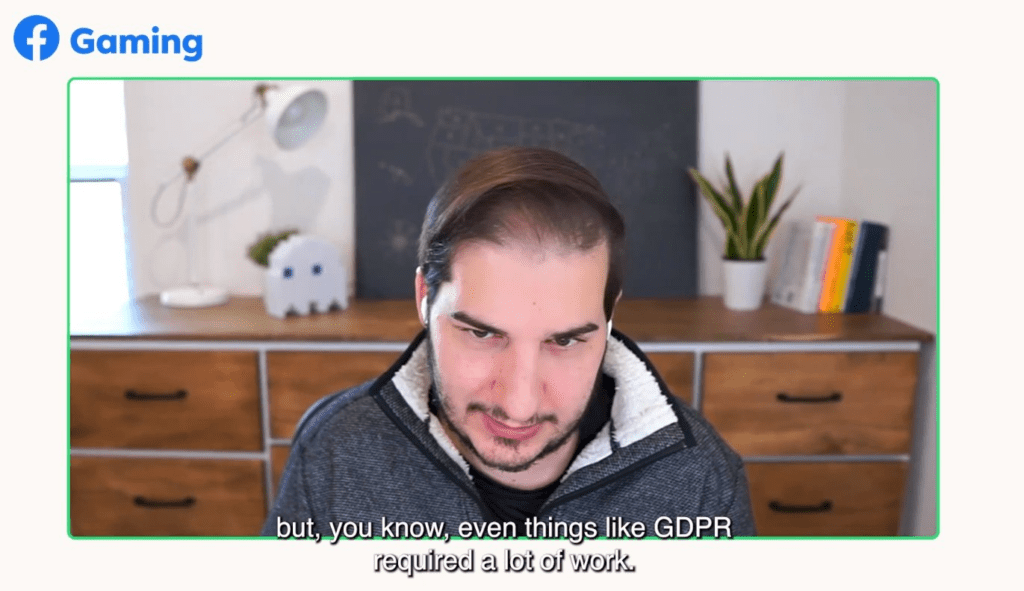
This point is usually papered over by those who aren’t operators, but most privacy issues are real non-events. GPDR required a lot of work, but things kept working after. iOS14 was different as it really changed how everything worked and we had to start again mostly from scratch.
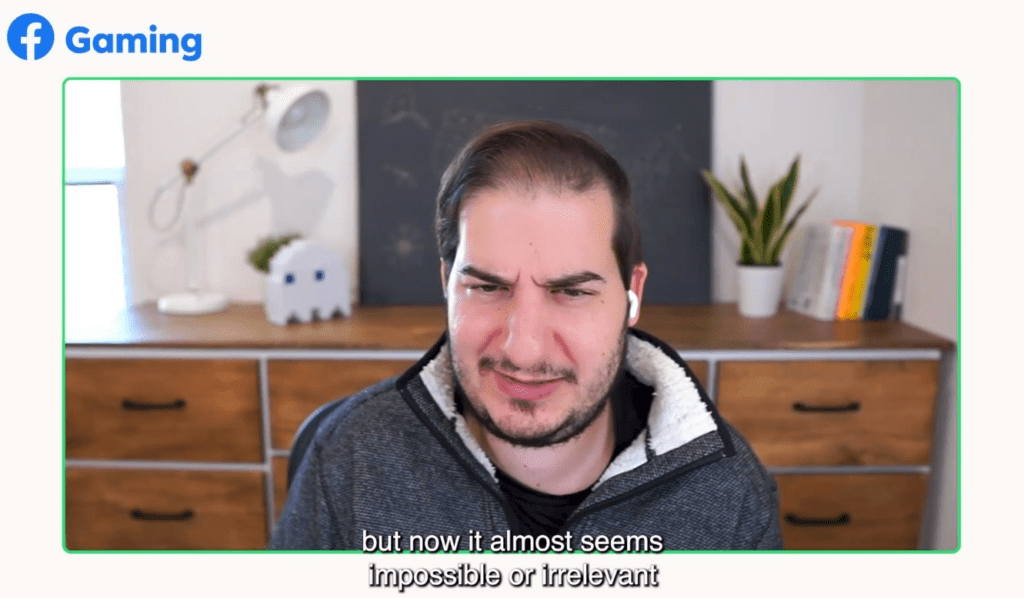
It’s weird to say this, but MTA (Multi-Touch Attribution) seems “impossible or irrelevant” now.
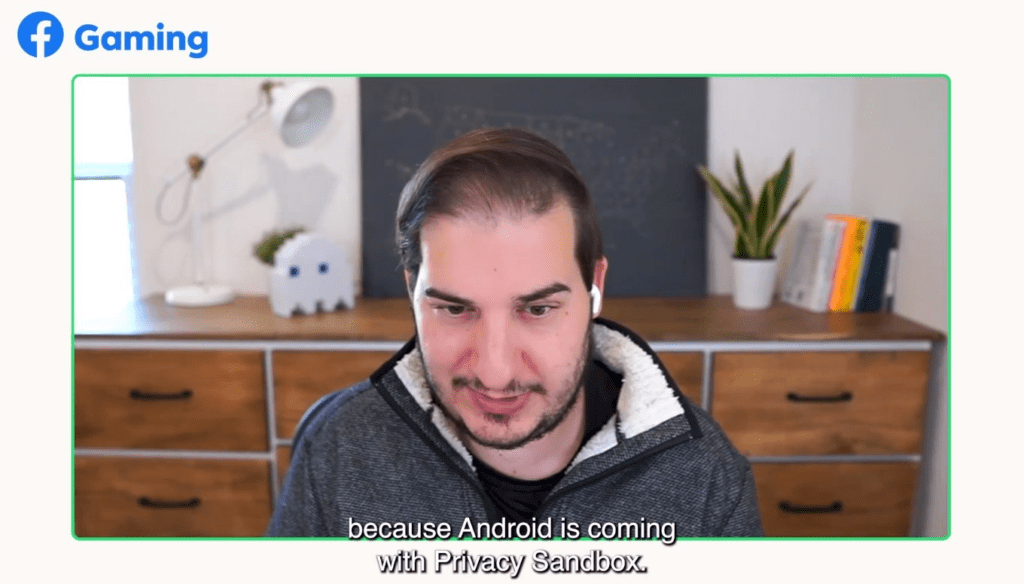
Echoing a point made earlier: nobody can afford to sit by idly and wait, because the same disruptive change that happened with iOS14 is coming to Android with Privacy Sandbox.
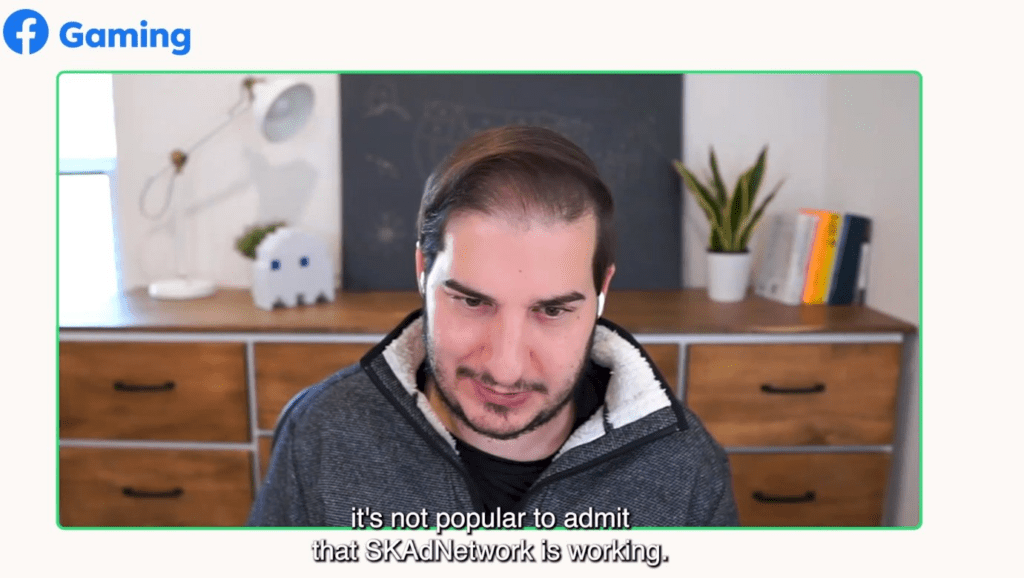
It’s not popular to admit it, but for some companies on the “SKAN train”, Apple’s tracking solution is actually working well enough, after some investment and iteration. It’s just fashionable to dunk on Apple now and blame them for making our lives complicated.
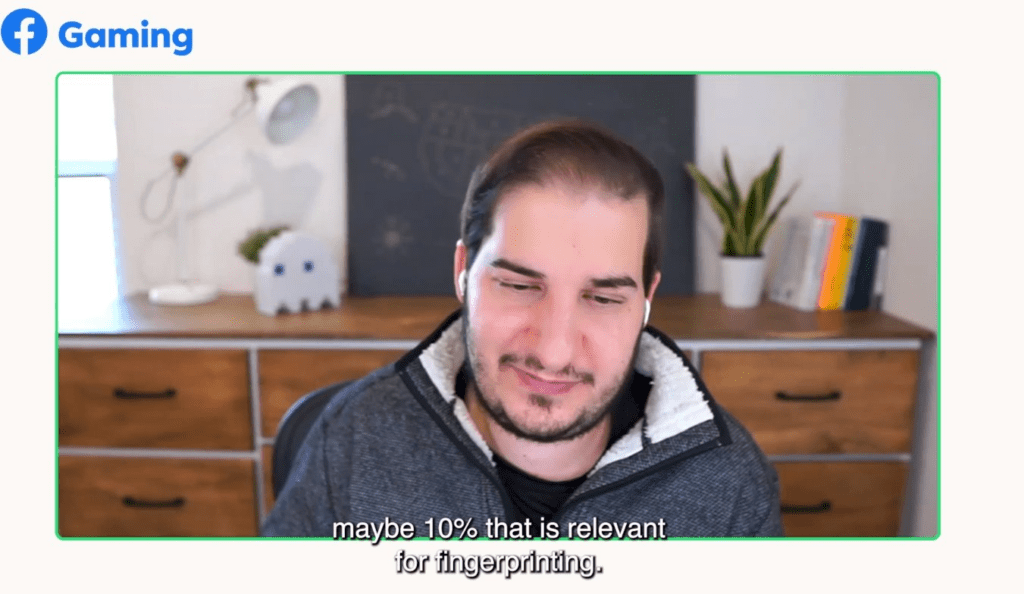
Only around 10% of ad traffic for mobile is even relevant for fingerprinting, because there’s no (documented) way to fingerprint traffic from the main networks like Google and Meta. So it’s essentially irrelevant as an attribution technique, even if it was allowed by Apple.
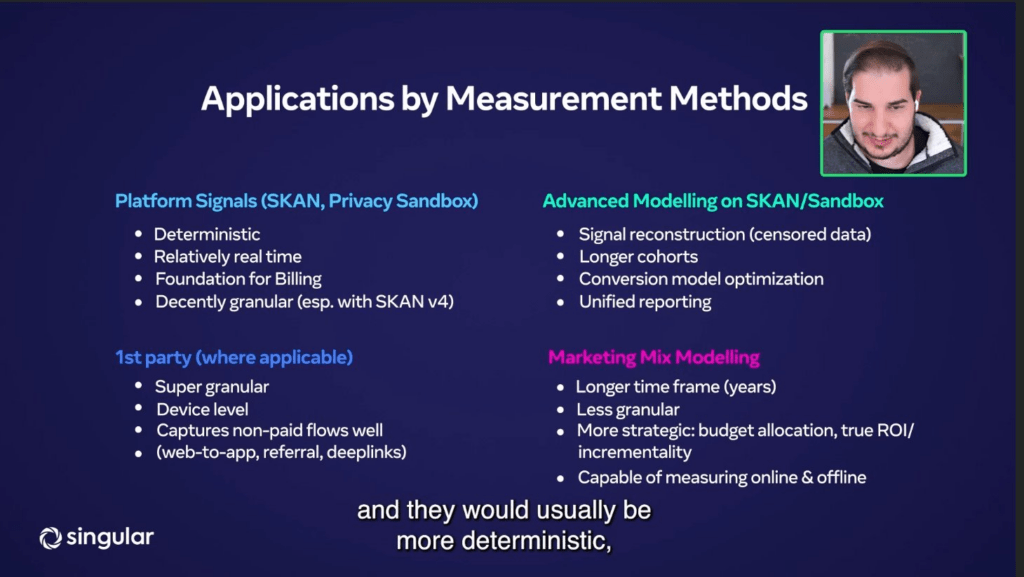
The key insight is that different attribution methods have different use cases. For example SKAN is for real time optimization / granularity, MMM for strategic budget allocation. None of them can do everything alone. Also an interesting admission here that Singular is reconstructing the data that Apple is censoring with SKAN (apparently?). It remains to be seen if this is even possible or what the relative accuracy is, but I imagine it will become less possible over time as Apple won’t like that.
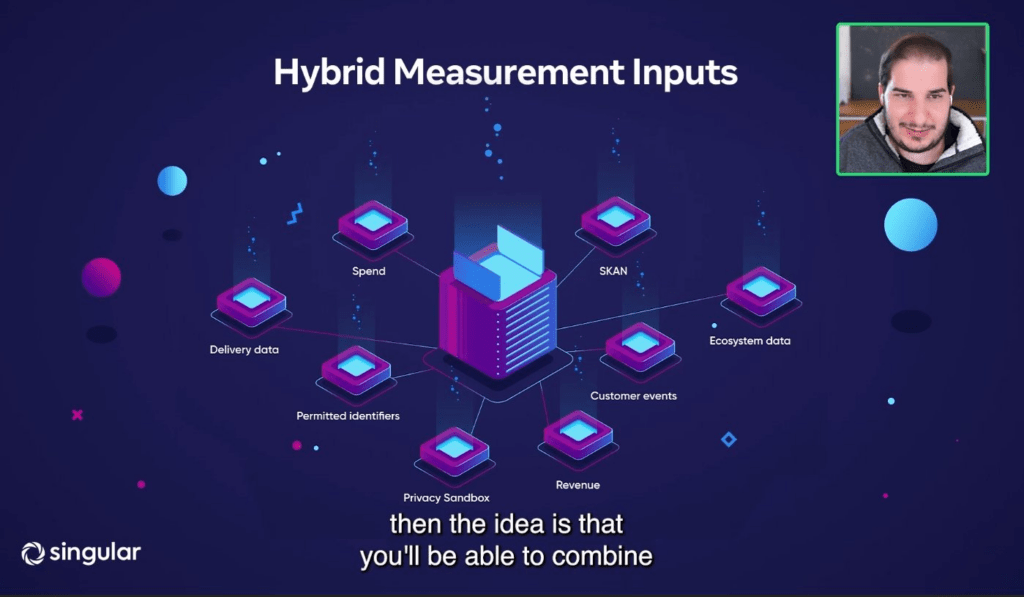
Simply getting all this data joined together in one place is a big challenge, and it’s something Singular can help with.
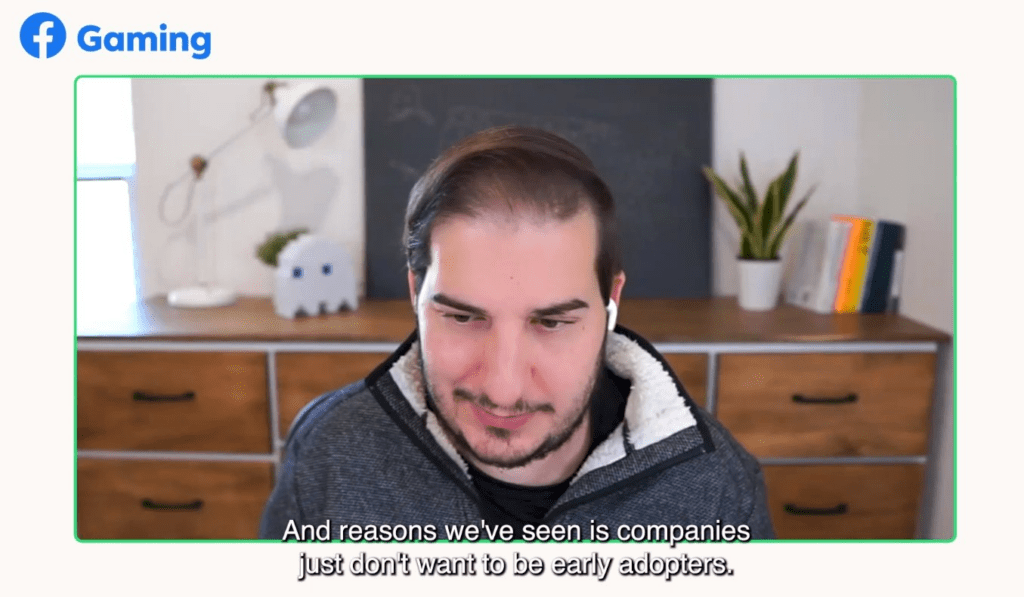
The hesitance around measurement techniques like MMM is because companies don’t want to be early adopters. They want proof, case studies, etc before they’ll commit investment.
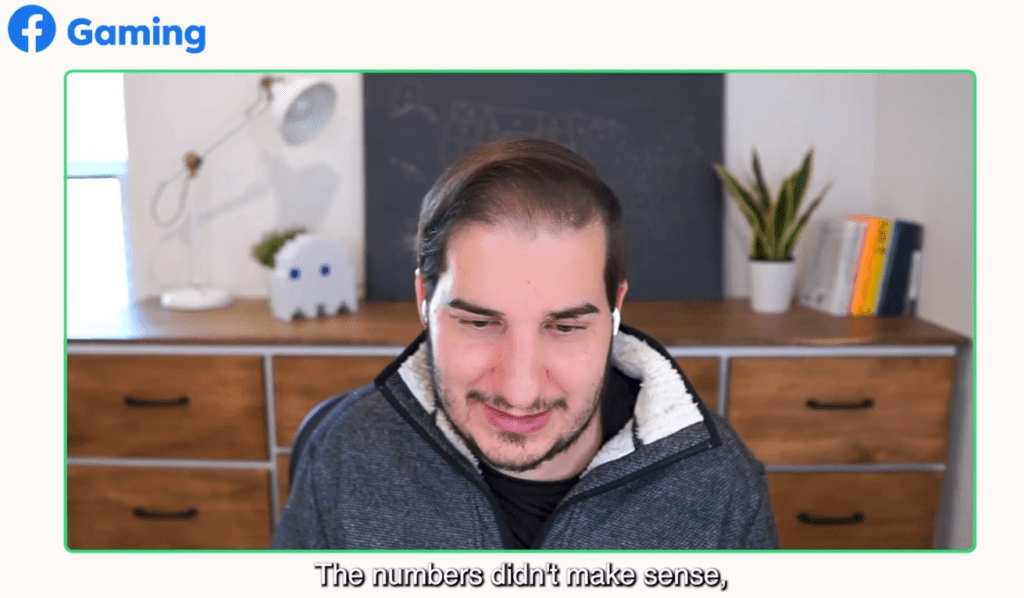
There’s also a cohort of companies that had a past bad experience with MMM, because it was super services-heavy and the numbers didn’t make sense.
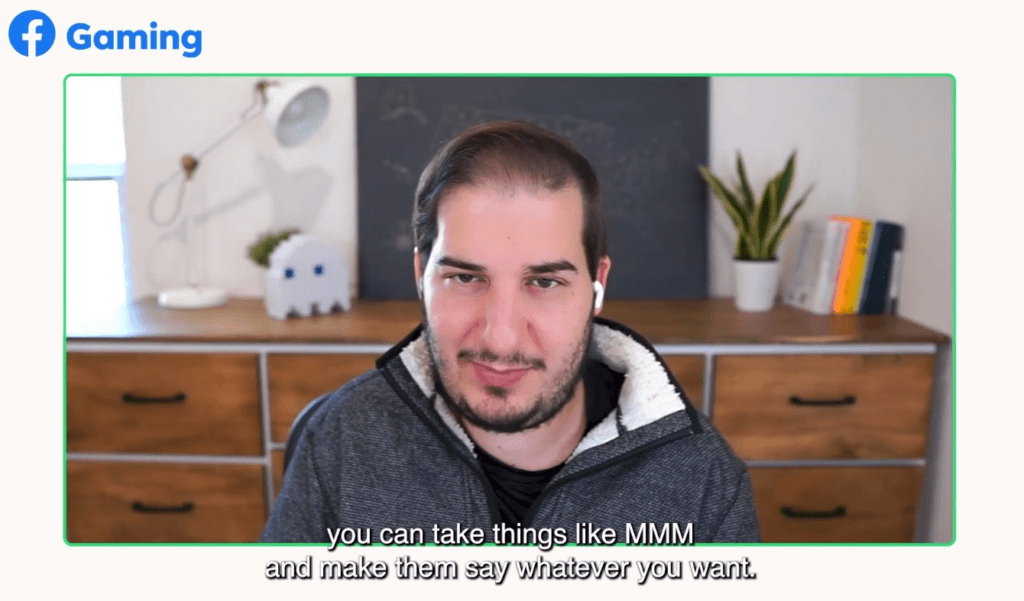
Yup that’s right, you really can take an MMM and make it say whatever you want. I’ve encountered a lot of fraud and politics in my experience in creating or critiquing MMMs. Human bias is the enemy here and you need automation and governance in place to protect yourself.
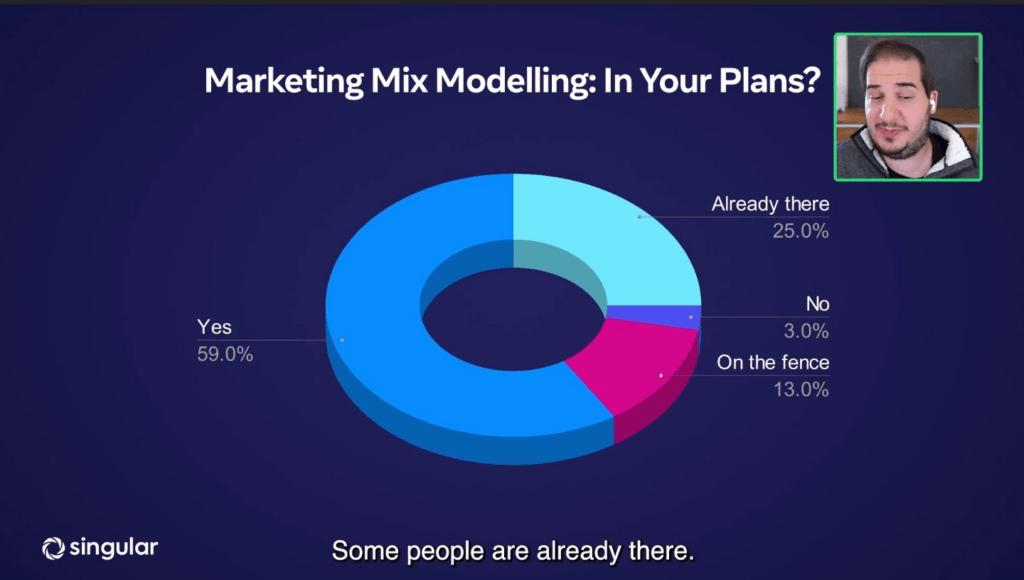
It says a lot that the vast majority of marketers plan to do an MMM, but very few of them have actually implemented it. MMM is very hard to do, and I would bet most of the “Already there” crowd aren’t yet fully in production.
Mobilityware’s Search For Truth And Higher Revenue In The Post-IDFA Storm
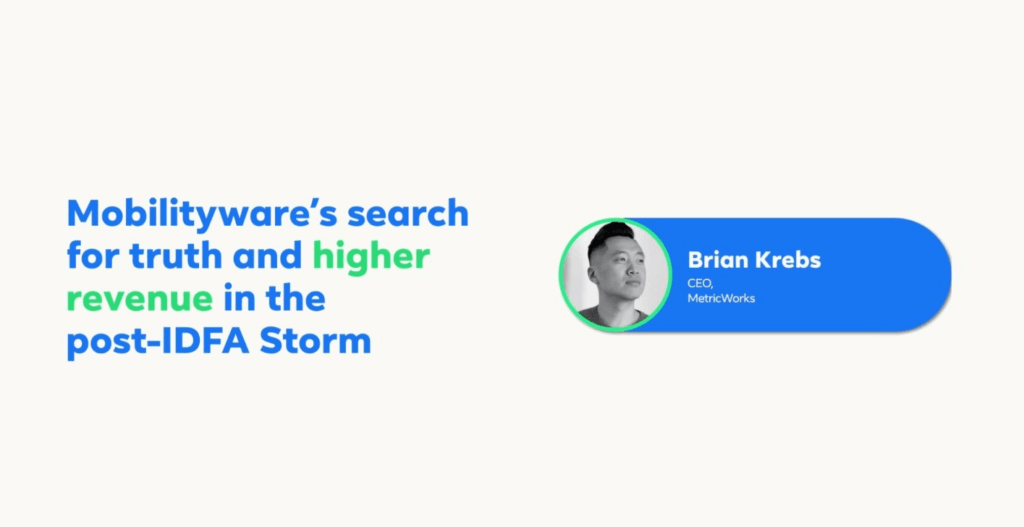
Next up is Bryan from MetricWorks – one of the smartest people I’ve talked to about MMM, so I was looking forward to this.
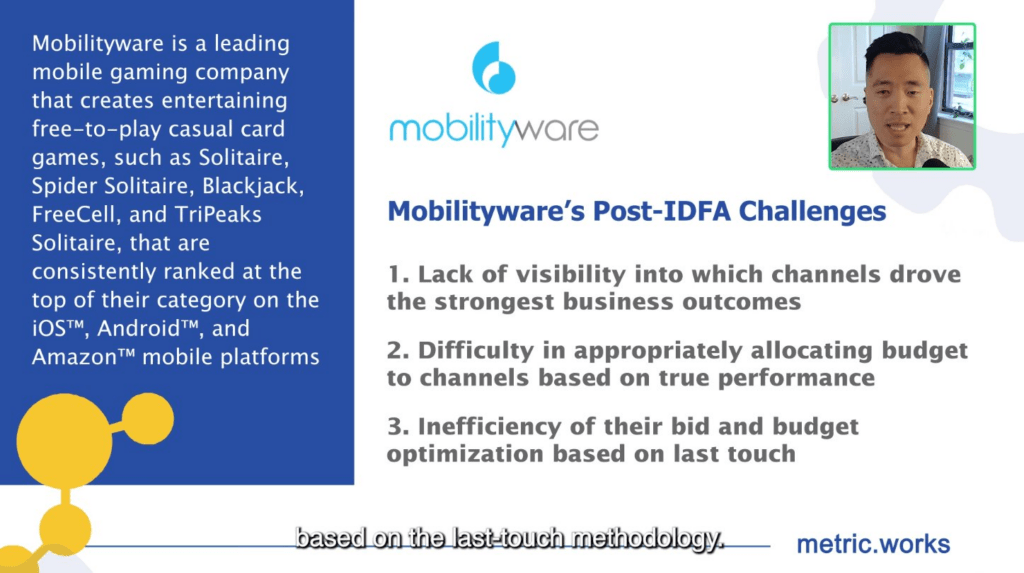
The case study is Mobilityware, a free-to-play games app (think Solitaire, Blackjack, etc).
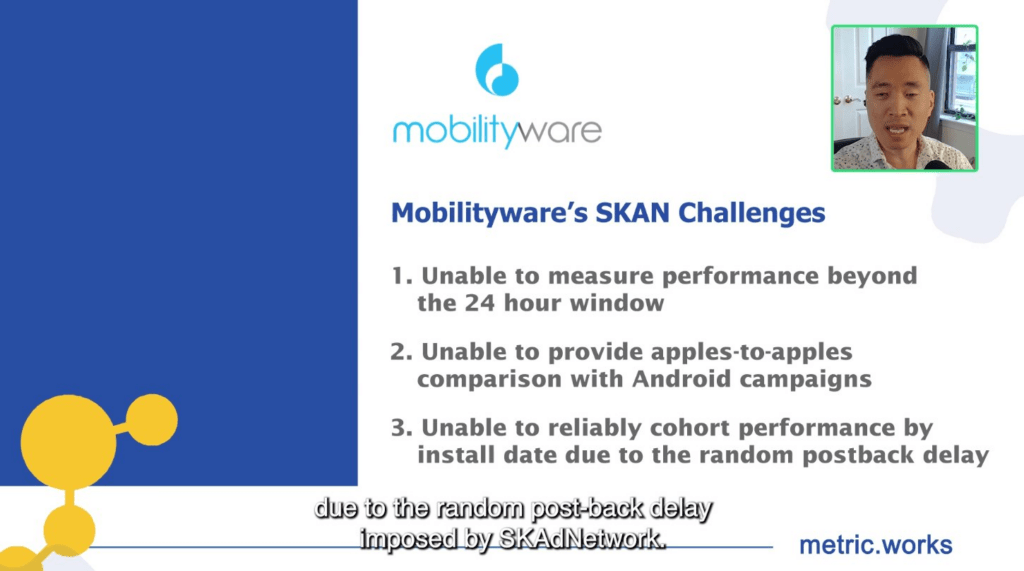
The issue was that SKAN delays postbacks and doesn’t give you visibility after a 24 hour window, so they couldn’t make good optimization decisions or compare across iOS and Android.
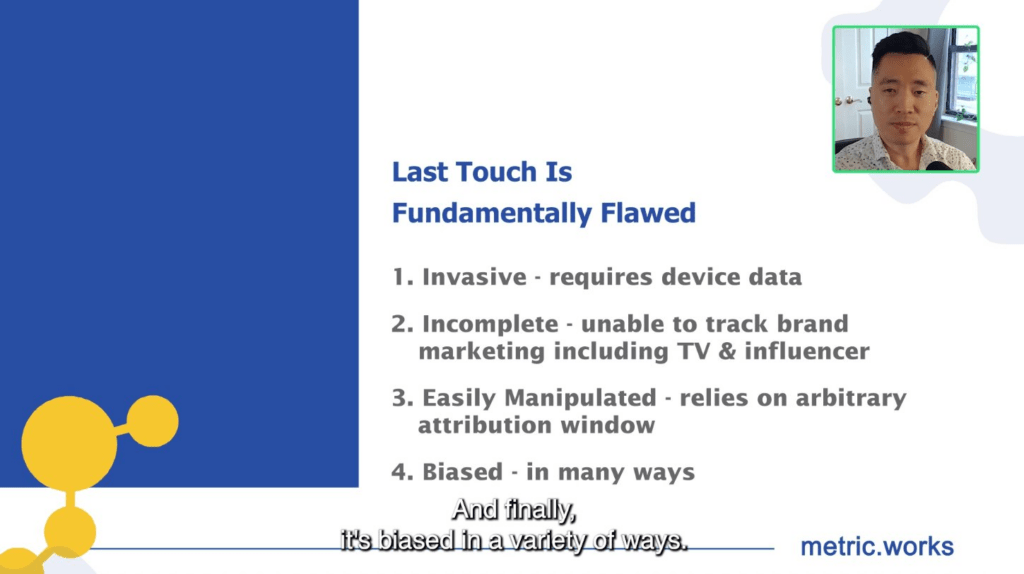
Last touch is fundamentally flawed. Note that most of this was true even before iOS14!
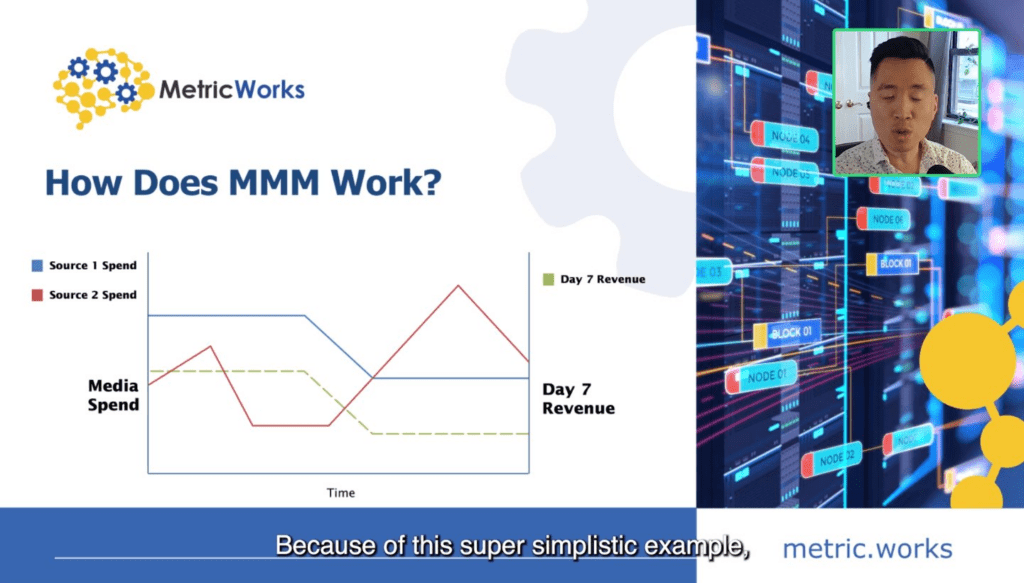
Super simple example of how MMM works. Spend on source one looks highly correlated with Day 7 Revenue, whereas Source 2 does not. The model can tease out those differences and assign relative ROIs to each channel.
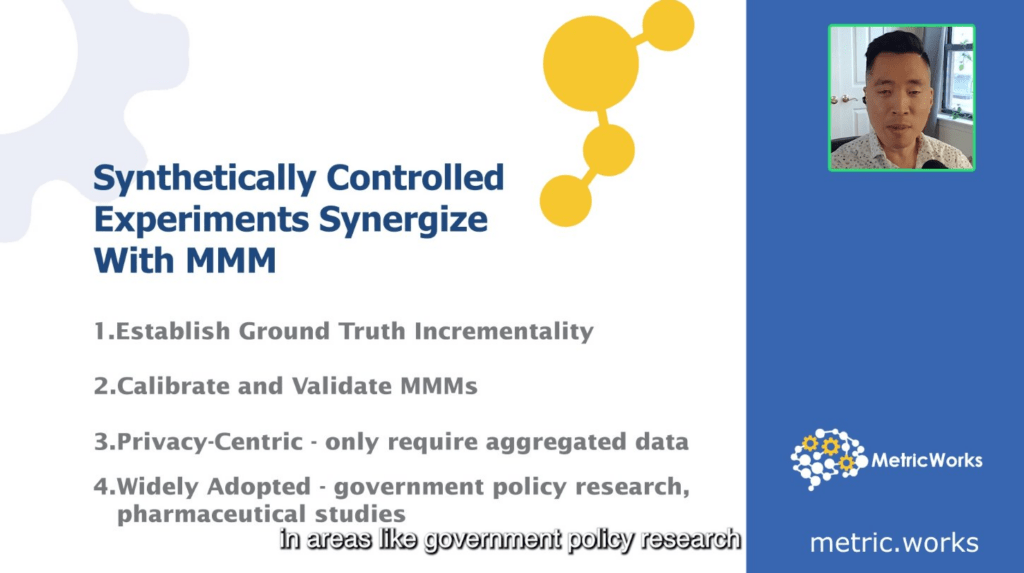
MMM is correlative, so how do you make it causal? Using synthetically controlled experiments (i.e. geo-lift) and using it to calibrate your MMM.
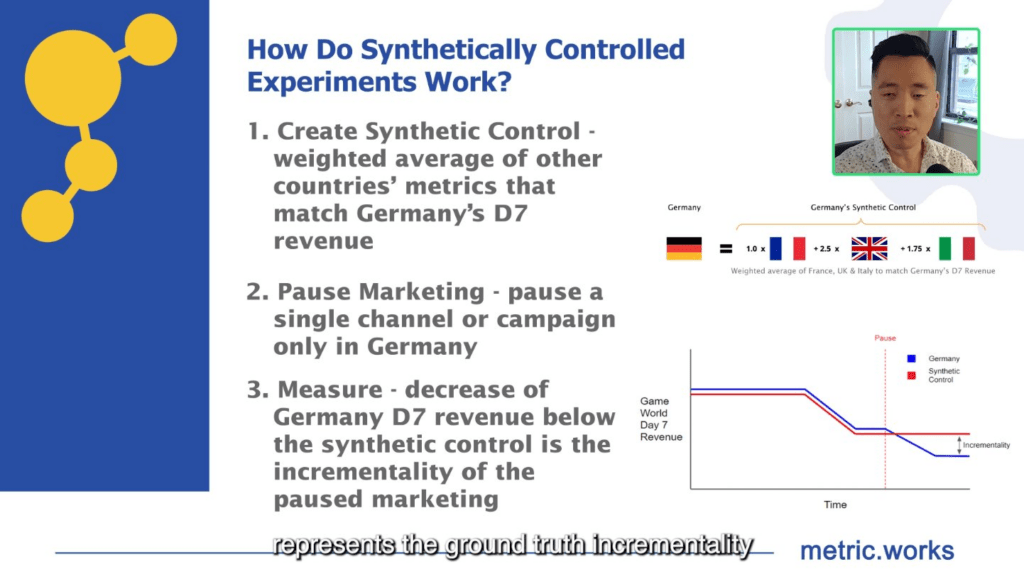
This is a really interesting and nuanced point. Unlike normal Geo-Testing (which suffers from several issues), synthetic controls help you estimate what revenue you would have gotten had you not paused marketing, by using a basket of weights constructed of other geo-regions.
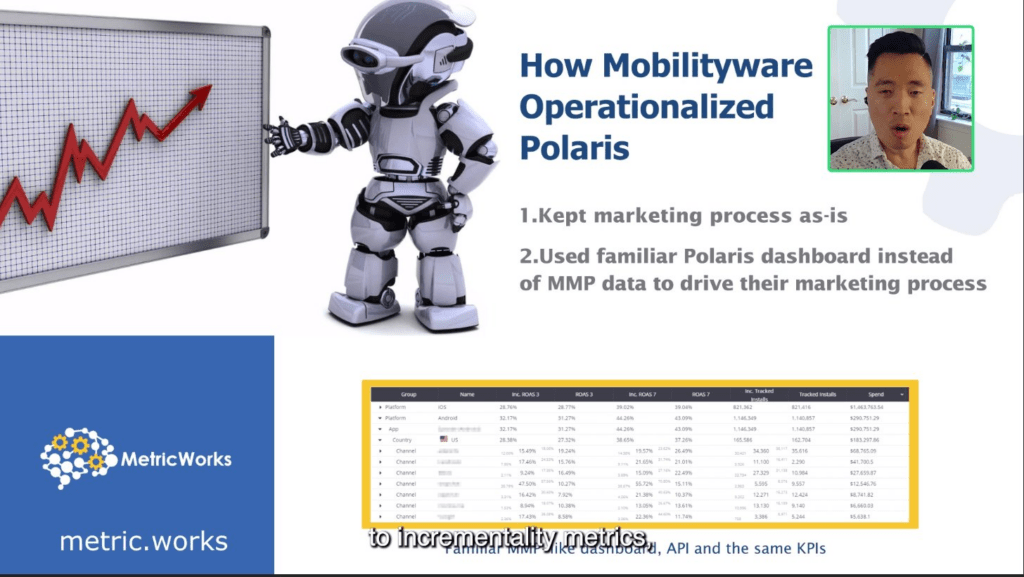
This is something I think MetricWorks have done well. Their dashboard basically looks just like what your team would already be used to within their MMP, i.e. AppsFlyer, Adjust, etc. But they swapped out last click metrics for incrementality adjusted metrics.
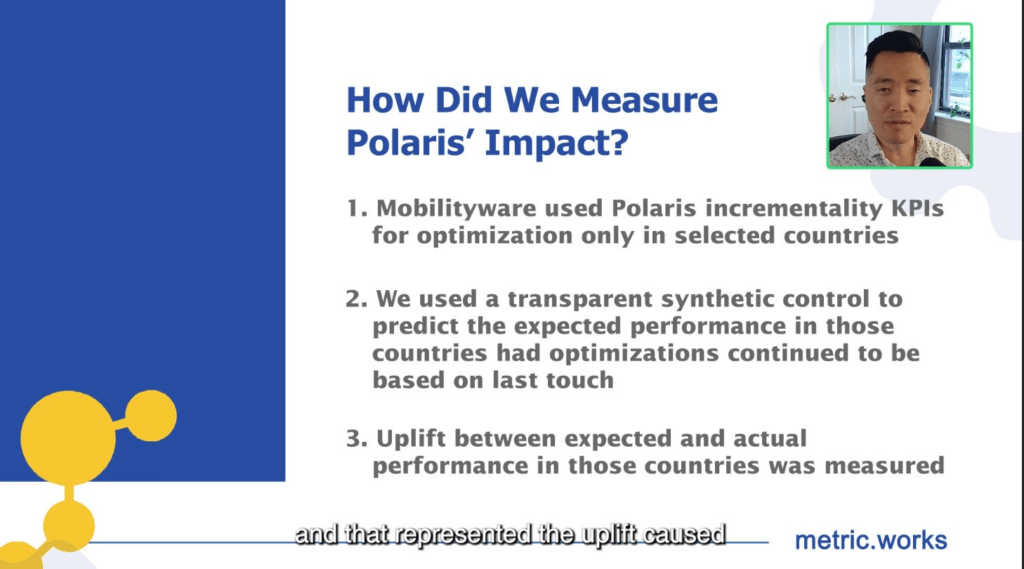
Lol ok so this is funny. “How did we measure the uplift of using our product? We ran a synthetic controlled geo-experiment of course!”. Love this approach: they basically kept using last click in some countries, used Polaris in others, and measured the difference. Measuring the incrementality of measuring incrementality… so meta.
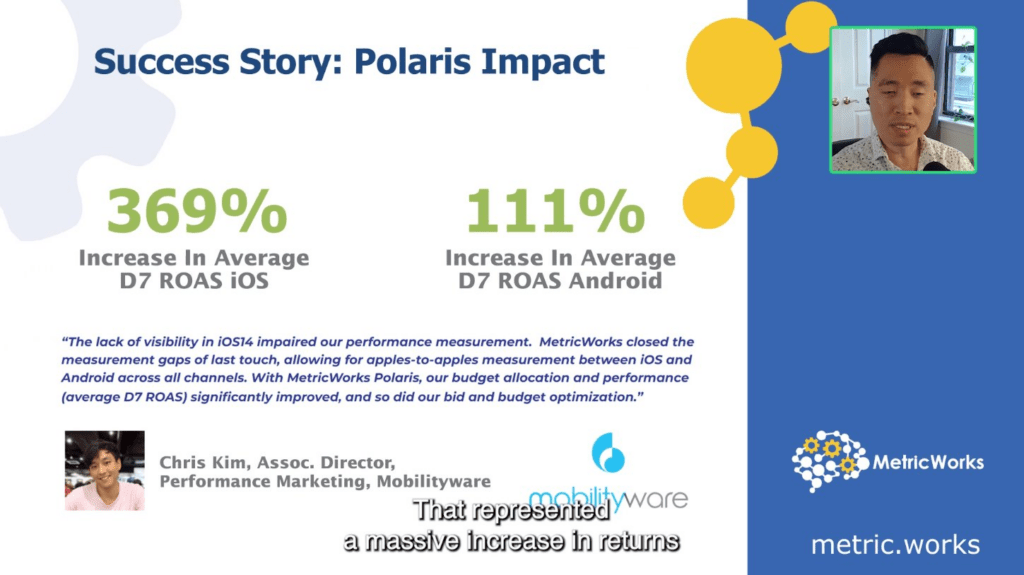
Big headline stat! I’m surprised it was this high even for Android, but incrementality can be a huge win for more complex high spend clients like this.
How App-Based Businesses Can Future-Proof Their Measurement Strategies
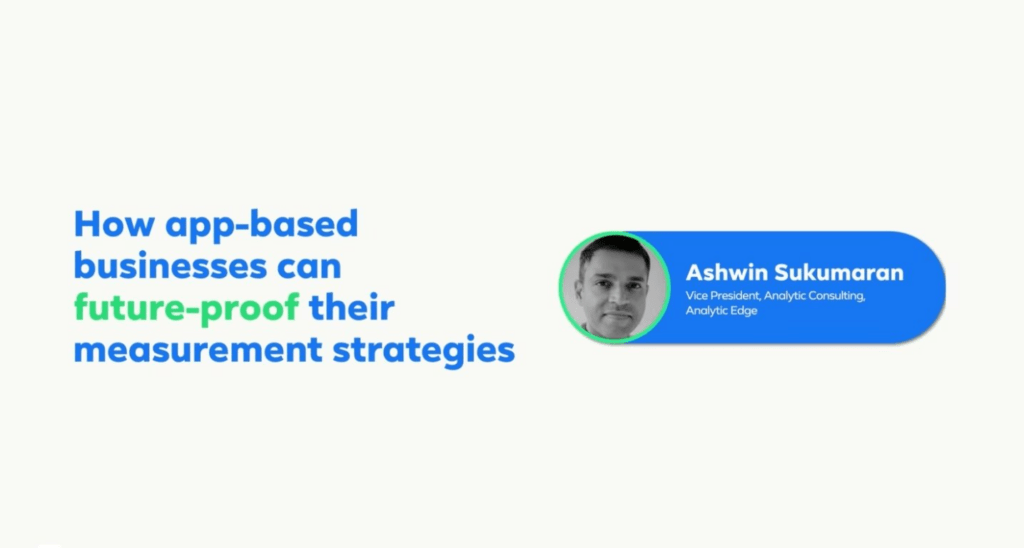
Ok next session. Now we have Analytic Edge who have featured in a few of these webinars, on how to future-proof your measurement strategy.
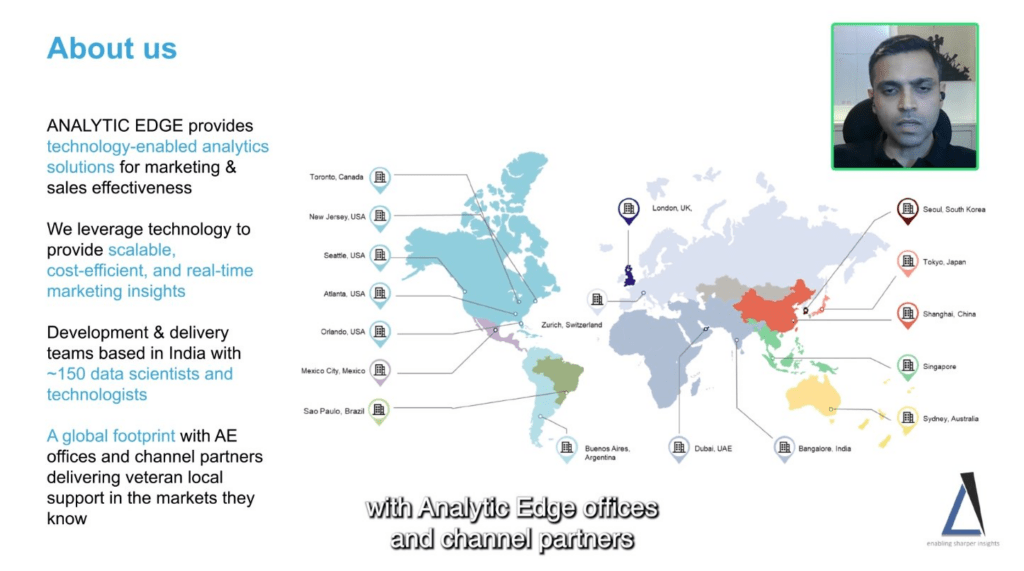
This is like the classic services pitch deck slide. “Look at all the people we have in different places around the world”. It’s impressive to most people but to me it’s an indicator their tech isn’t fully automated.
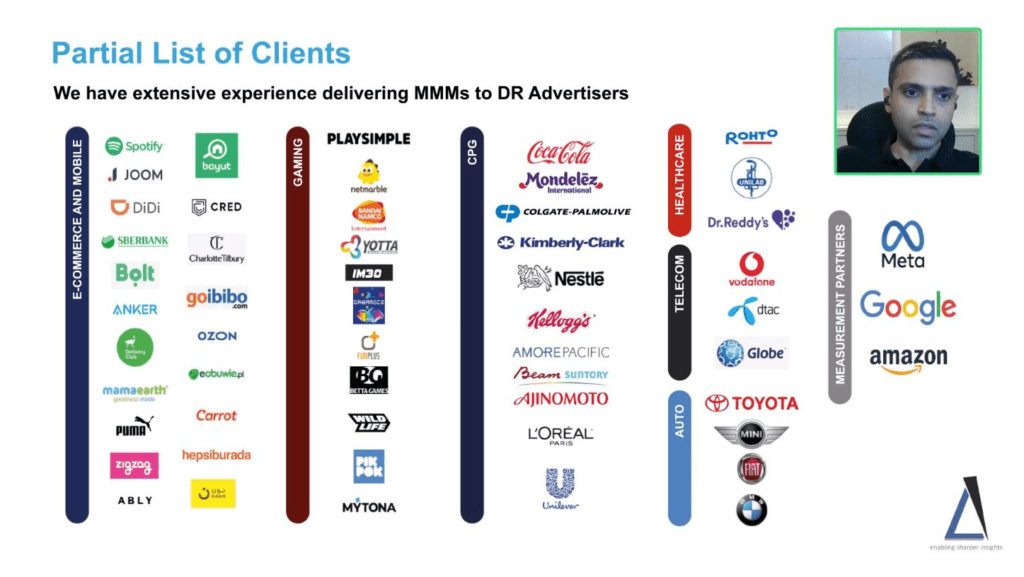
They do have a pretty wild list of clients though. If anyone asks “who does MMM” this serves as a pretty good list of important companies using the technique.
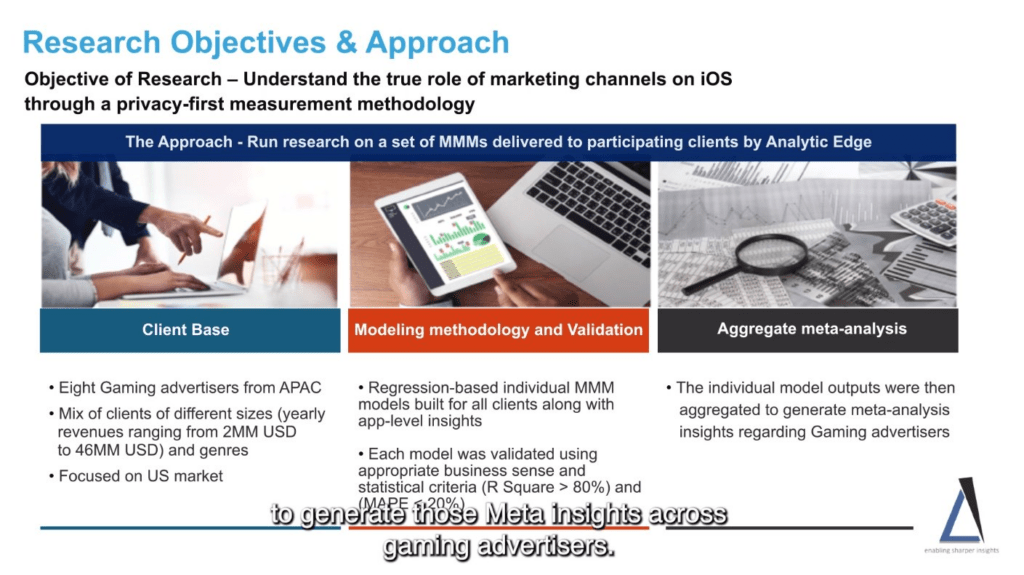
They did a research piece across 8 gaming clients. Interesting to note they went as low as $2m revenue per year (most people think you need to be big to do MMM). Disappointing to see R squared quoted as an accuracy metric in this day and age!
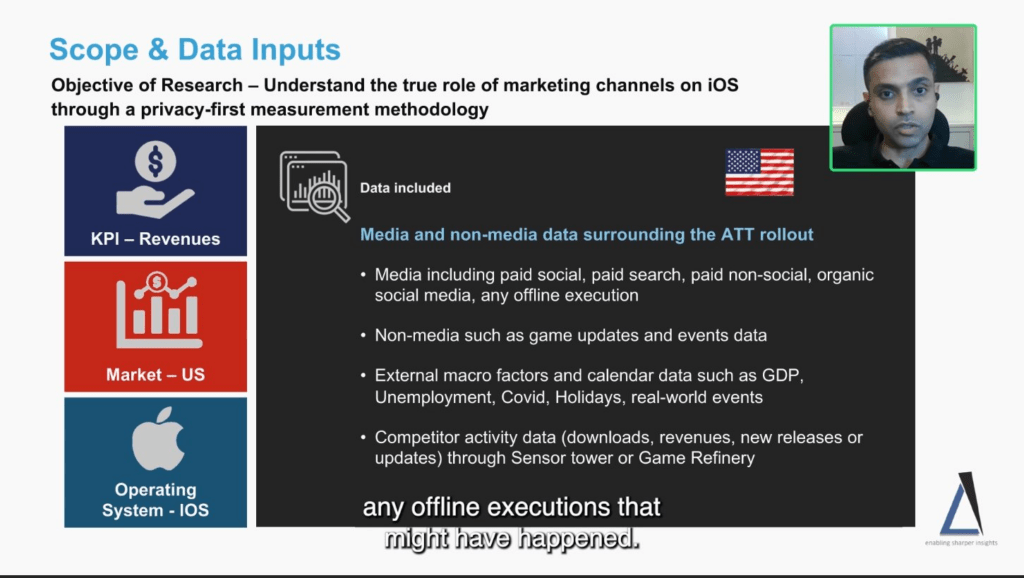
Incorporating competitor activity is a good one: hard to get this data but in mobile there are actually decent providers like Sensor Tower.
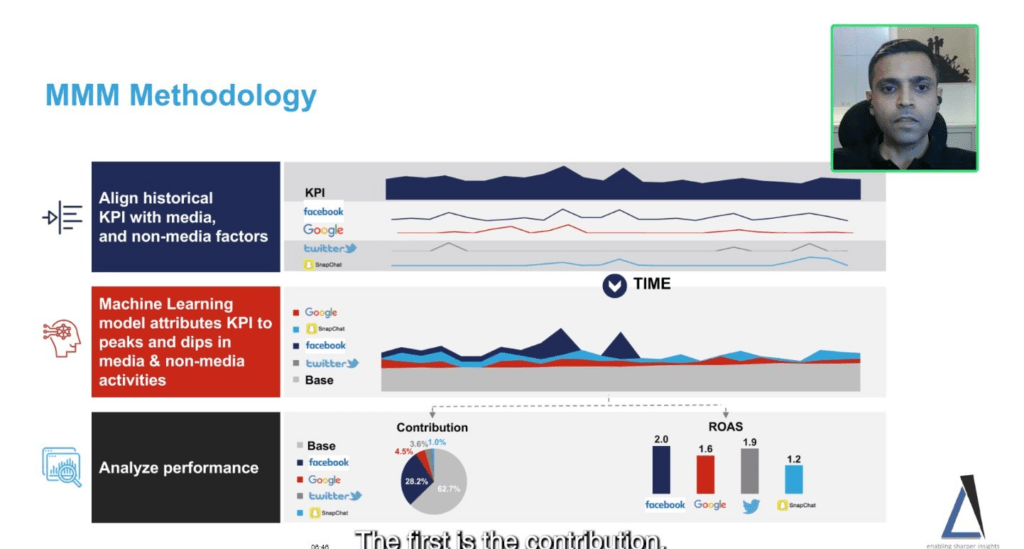
I quite like this as a simple explainer visualization of MMM. First you take a time series line for each channel and sales, then you build a model to use the channel lines to explain the sales line, then you can break it down by contribution.
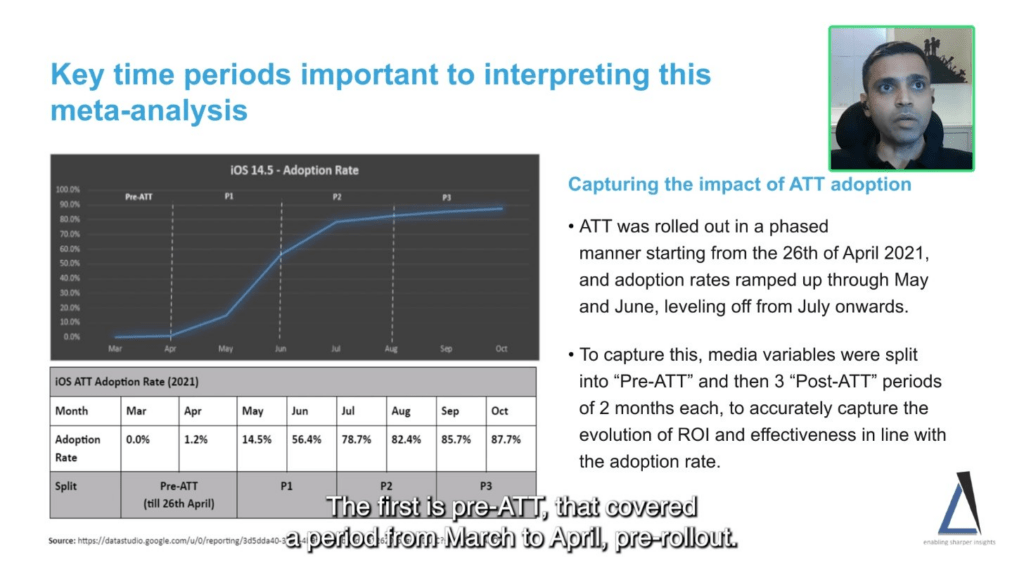
If you’re looking at historical data it’s important to split out the different periods of adoption rates, as iOS14.5 (the one that killed tracking) wasn’t fully adopted all at once.
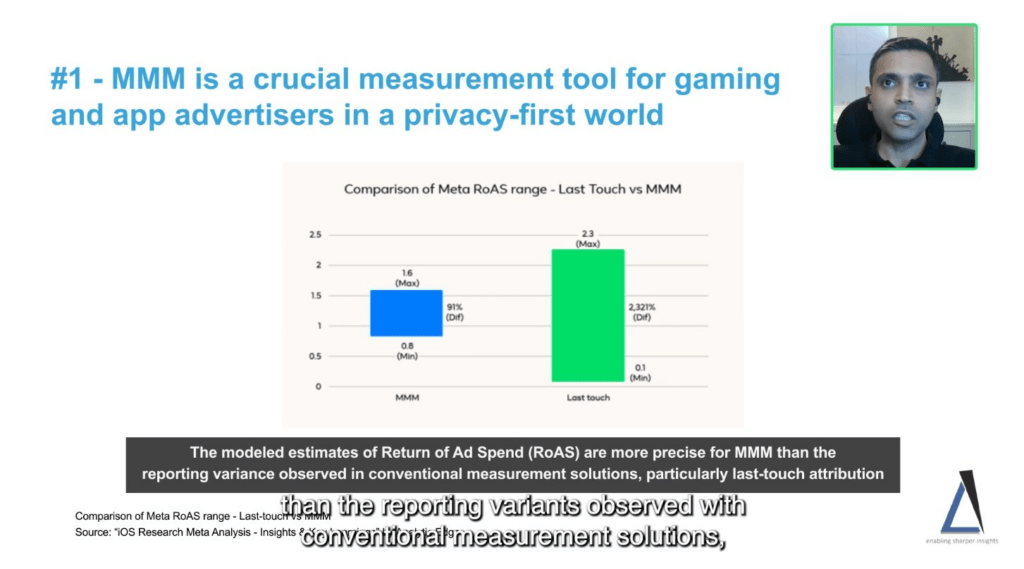
He’s trying to make the point that MMM is more stable in its estimates than last click… but honestly that’s kind of meaningless. We don’t have any proof that MMM is ‘more accurate’ than last click, so stability of ROAS could be good, bad, or neutral.
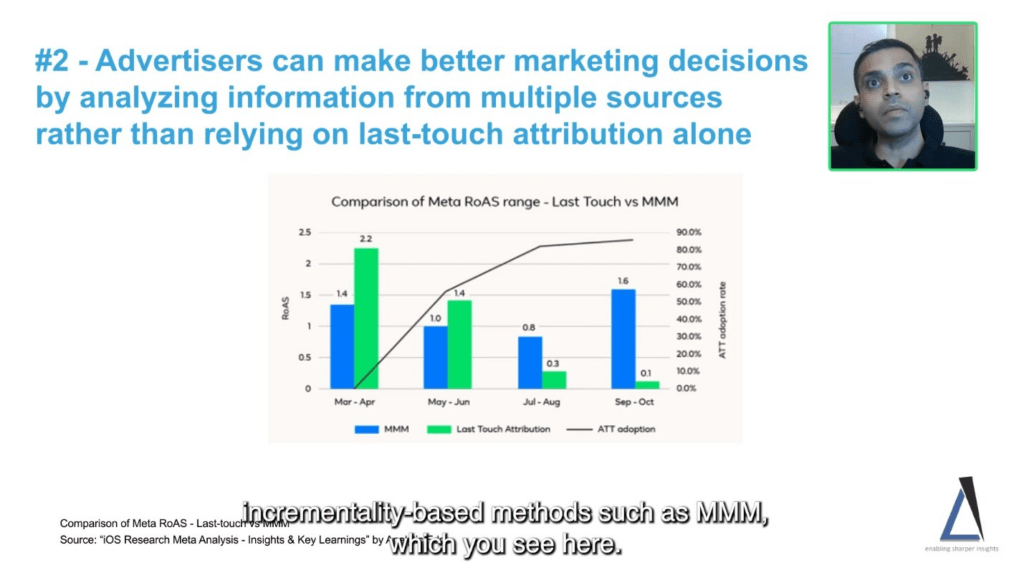
Ok so this is what he was getting at, which is super interesting. Apparently last click is severely misreporting as iOS14.5 gets more adopted, even though ‘true’ (MMM) performance is up. Though this is so drastic I would be suspicious. This is saying for context, that last click reporting shows Meta going from 2.2 ROAS –> 0.1 ROAS. Doesn’t jive with anything I’ve seen.
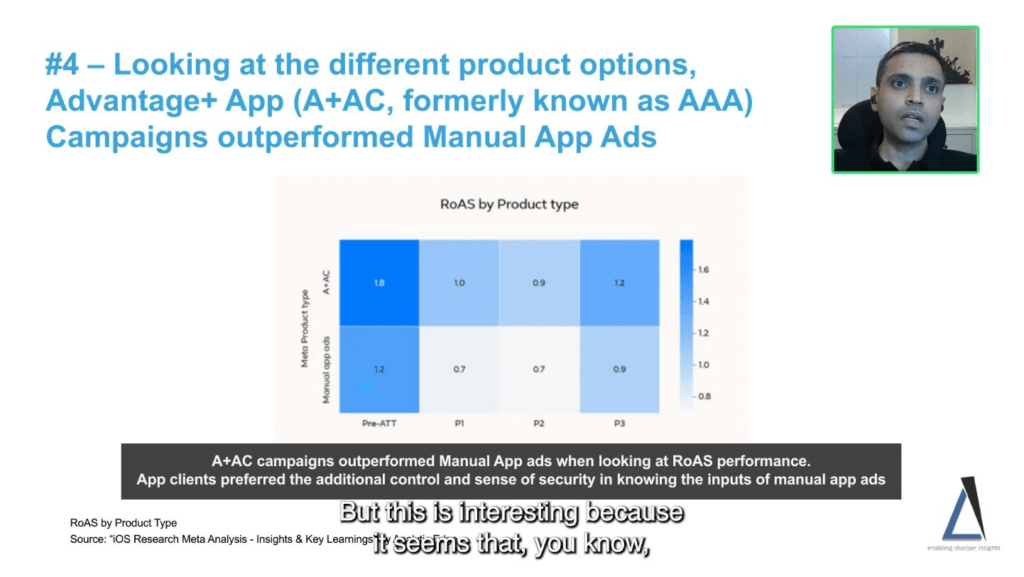
Some evidence that automated ads (formerly AAA, not Advantage+) performed better than manual campaigns even as measured incrementally by MMM.
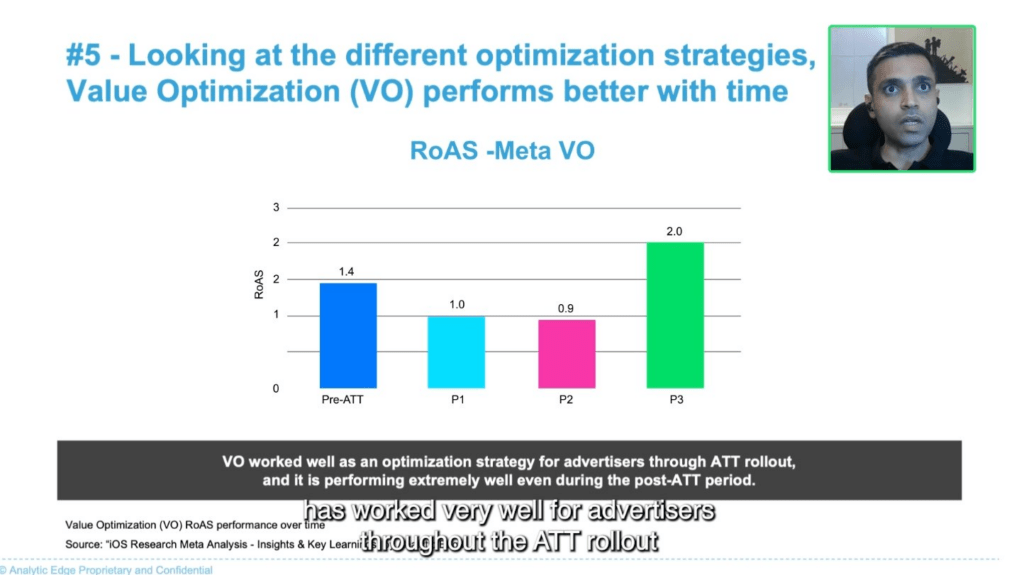
Value optimization seems to perform better over time with iOS14 adoption (no idea why though?).
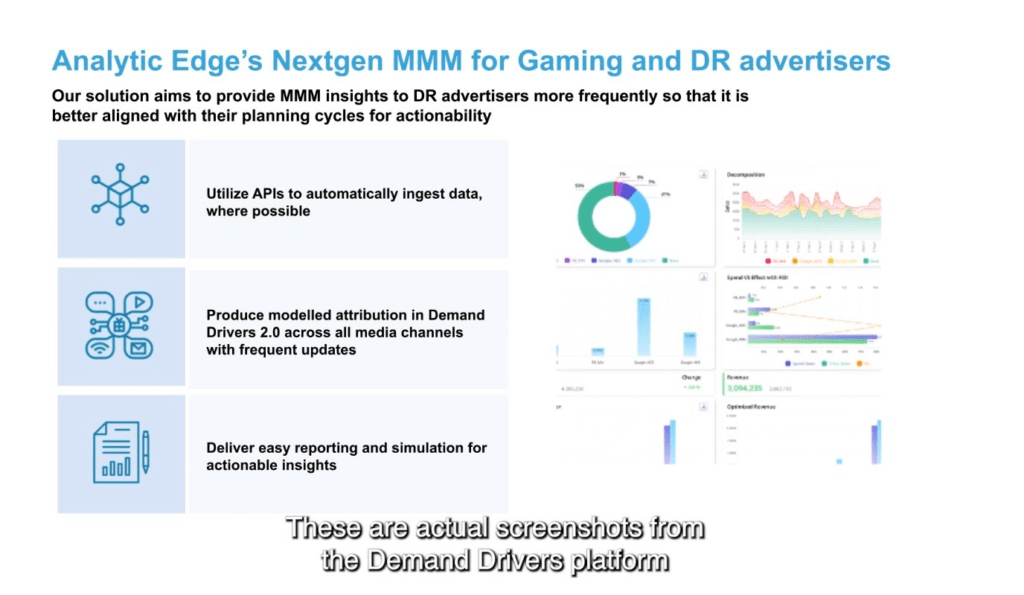
They’ve updated Demand Drivers (their proprietary MMM solution) to be updated more frequently through data pipelines.
The Marketing Mix Modeling Experience: Insights From Gume
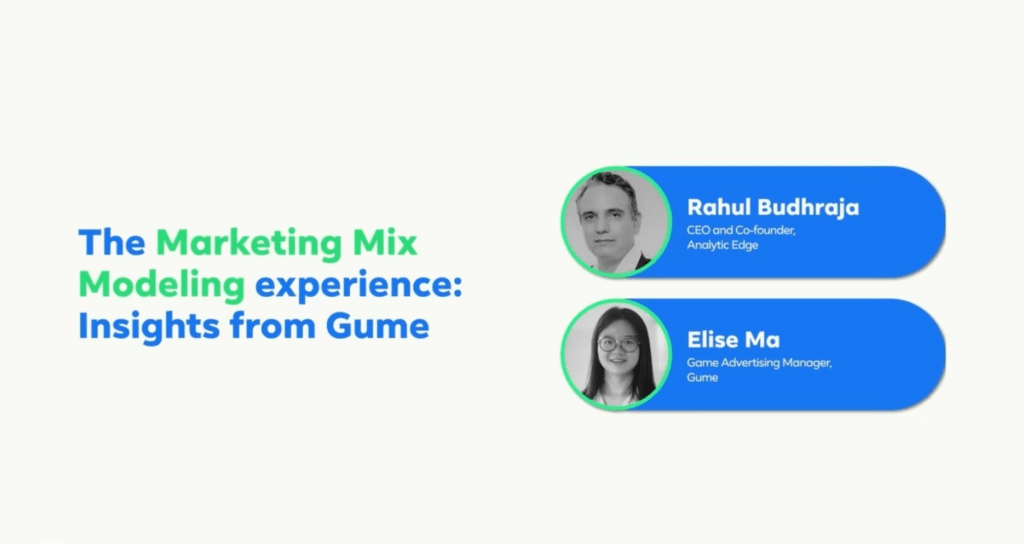
Another Analytic Edge session! Wonder what you have to pay to get this level of exposure 😉
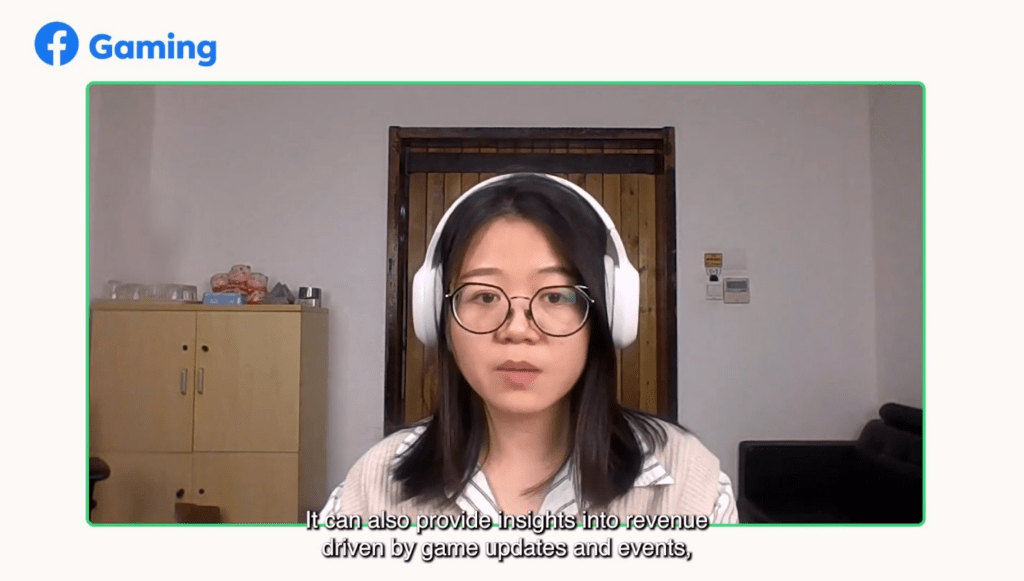
One under-credited benefit of MMM is that you can see the contribution of organic activities like game updates, or macro factors, which you can’t really get with any other attribution method.
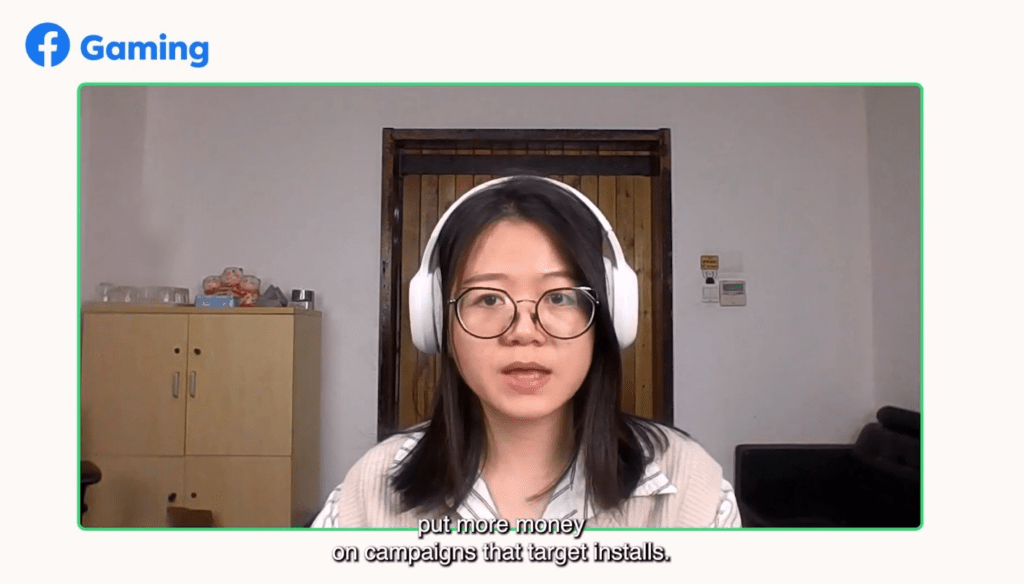
They found with MMM that rather than advertising to existing users, they should invest more at the top of the funnel to get new users (a common finding in the traditional marketing world too: try and reach everyone in your category!).
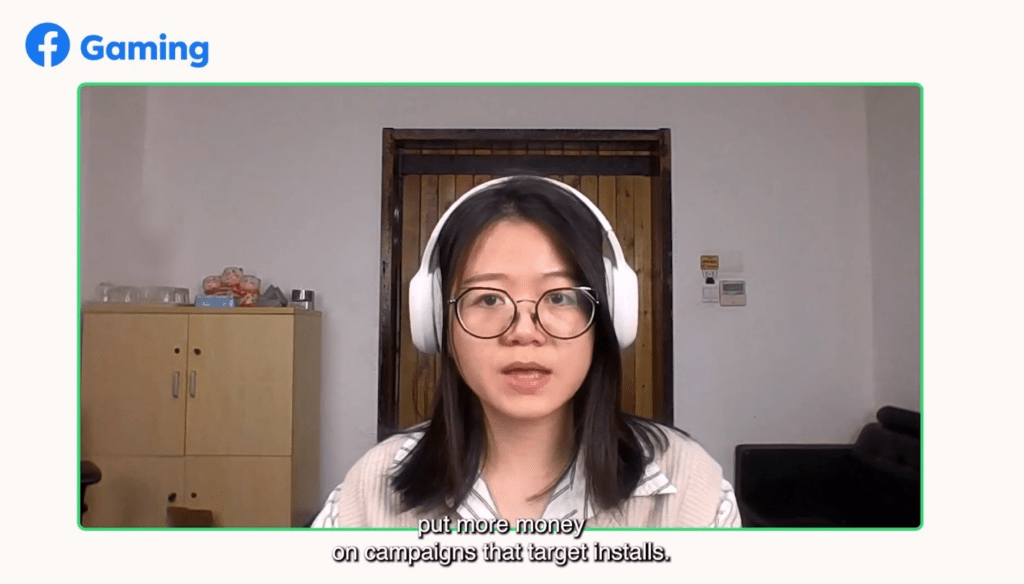
Ok that session was kind of just a PR thing for Analytic Edge. Not that useful. Next session looks more promising as it’s Meta showcasing a client directly: Warner Bros.
Measurement Insights From Warner Bros. Discovery
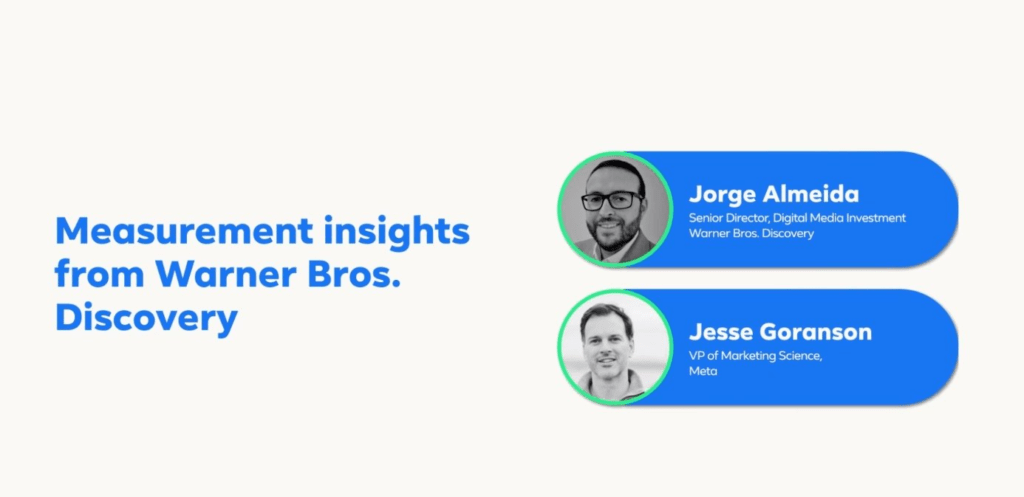
Ok that session was kind of just a PR thing for Analytic Edge. Not that useful. Next session looks more promising as it’s Meta showcasing a client directly: Warner Bros.
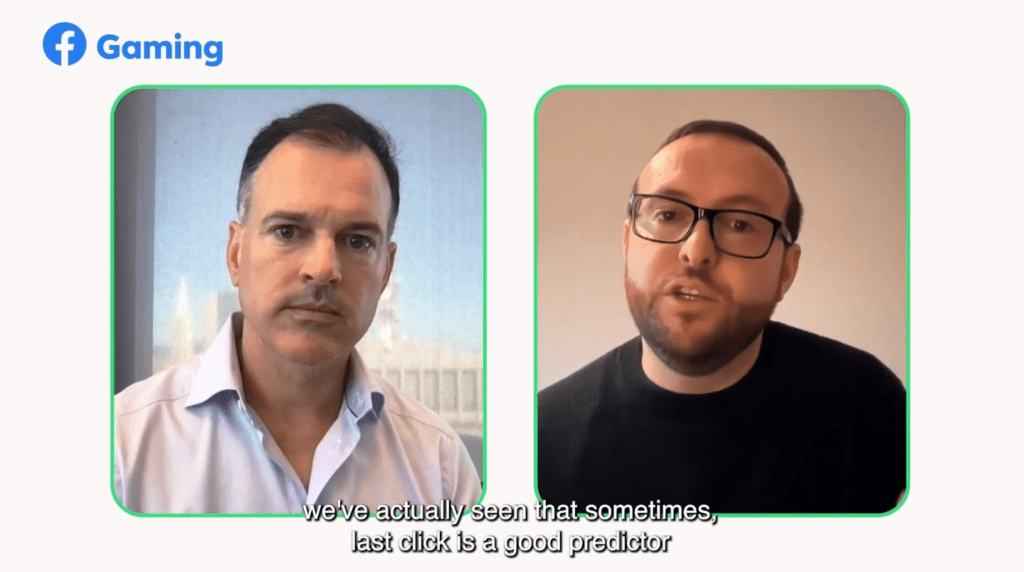
Important to note this given all the hate last click gets. Sometimes it’s actually a good predictor of incrementality!
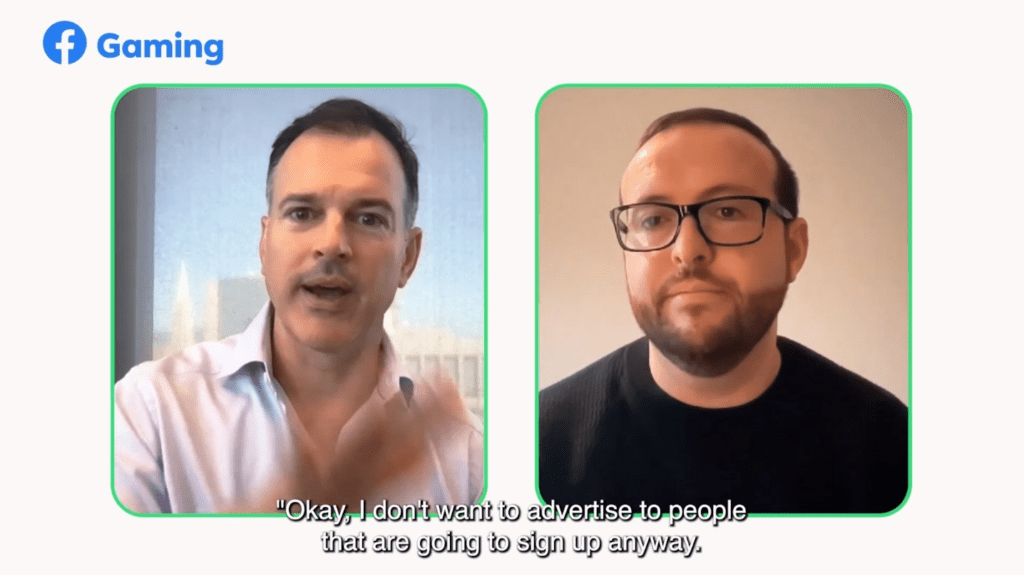
Scenarios where last click can be incremental:
1/ low awareness product
2/ simple channel mix (1-2 channels)
Otherwise you’re likely paying to advertise to people who were going to sign up anyway.
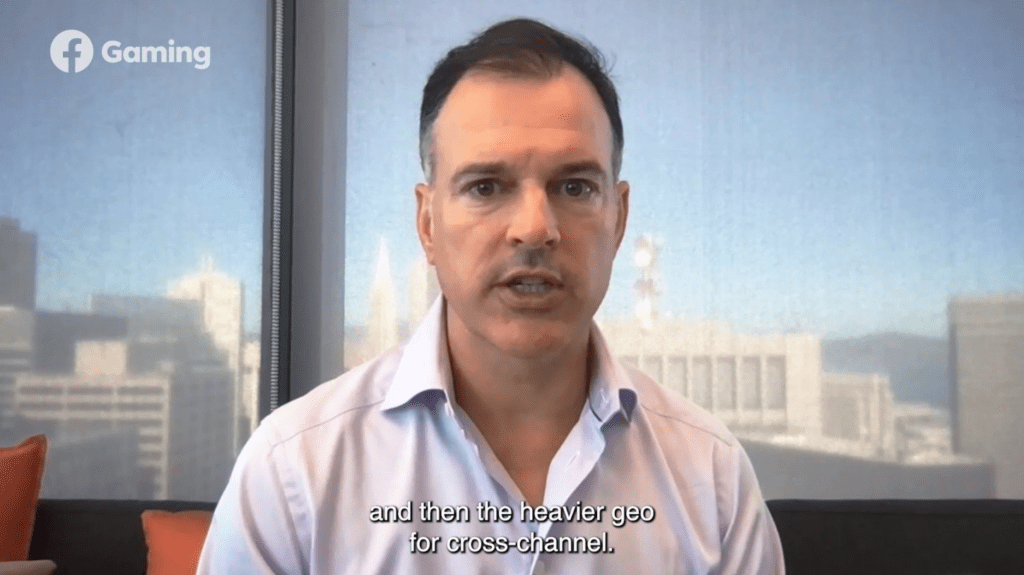
Common best practice is to use lift studies to measure in-channel, and geo-testing to measure cross-channel effects.
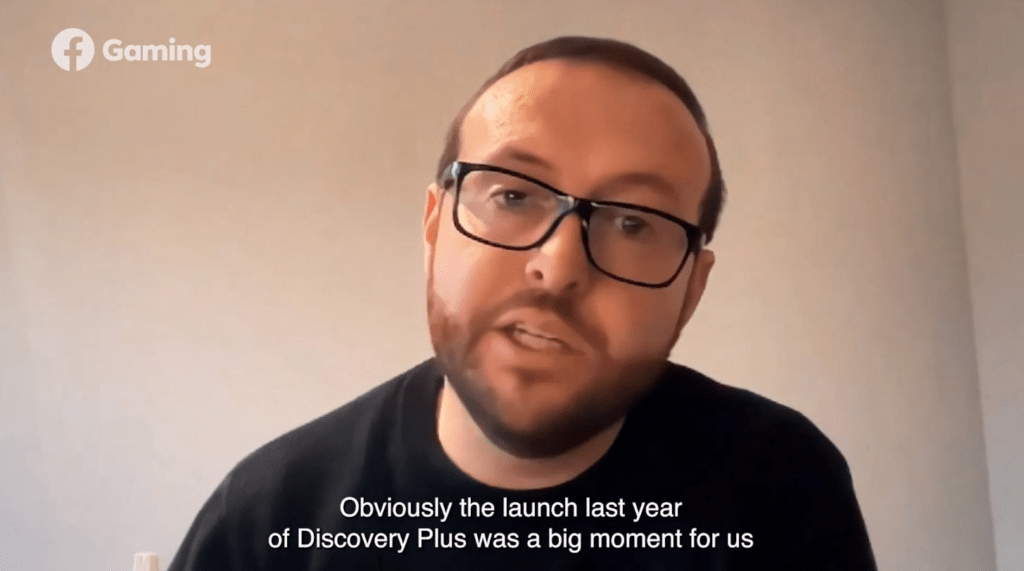
Some interesting color on their launch of the Discovery Plus streaming service, which necessitated a complete retraining of their MMM.
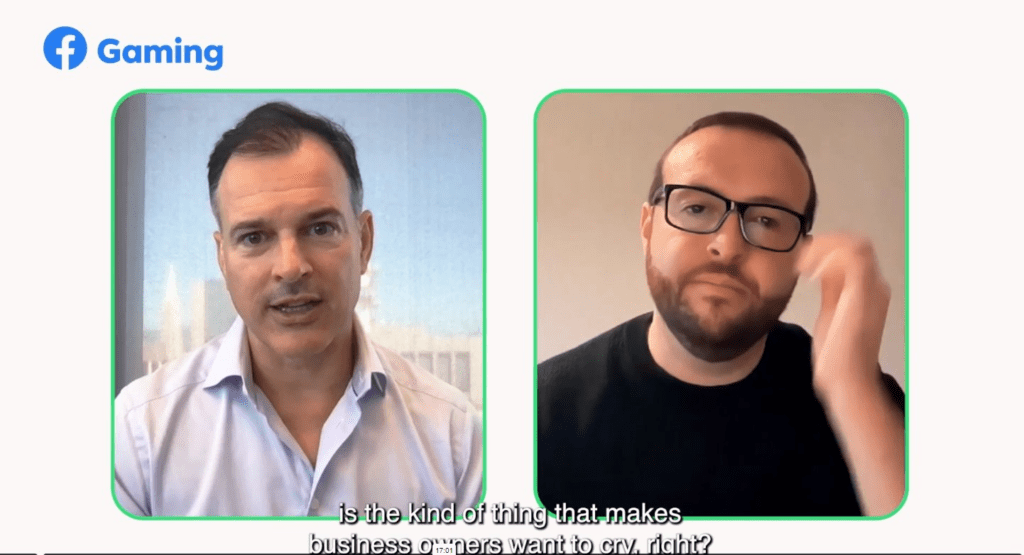
Yes! Advocating a 10% – 20% holdout even on big launches so you can measure incrementality. As he says this is the kind of thing that “makes business owners want to cry”. 🤣 This is exactly the topic we were writing about for Reforge in the ROI of Testing.
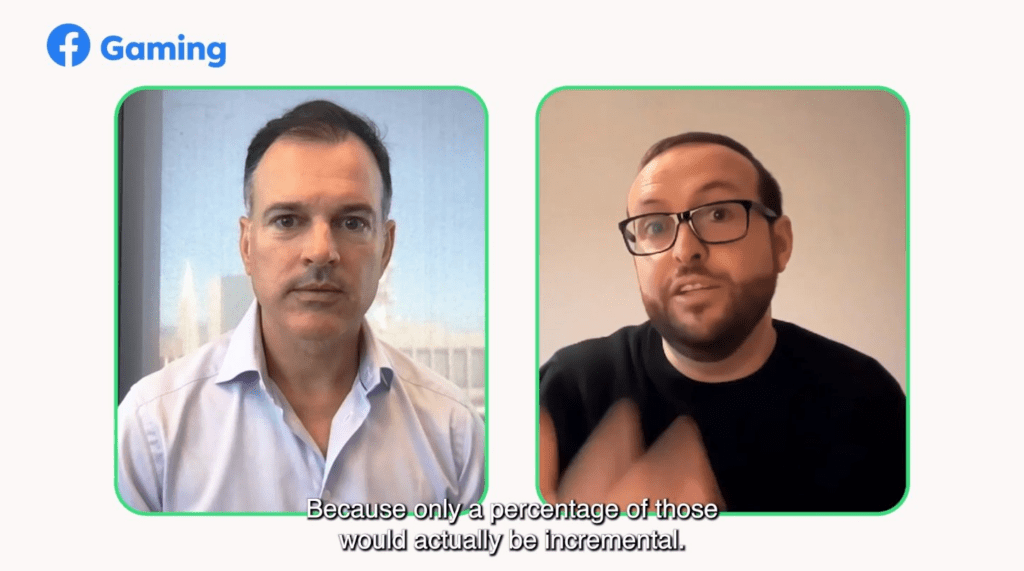
Exactly this: if you have a holdout group you’re not ‘losing’ conversions from that group if not all the conversions are incremental.
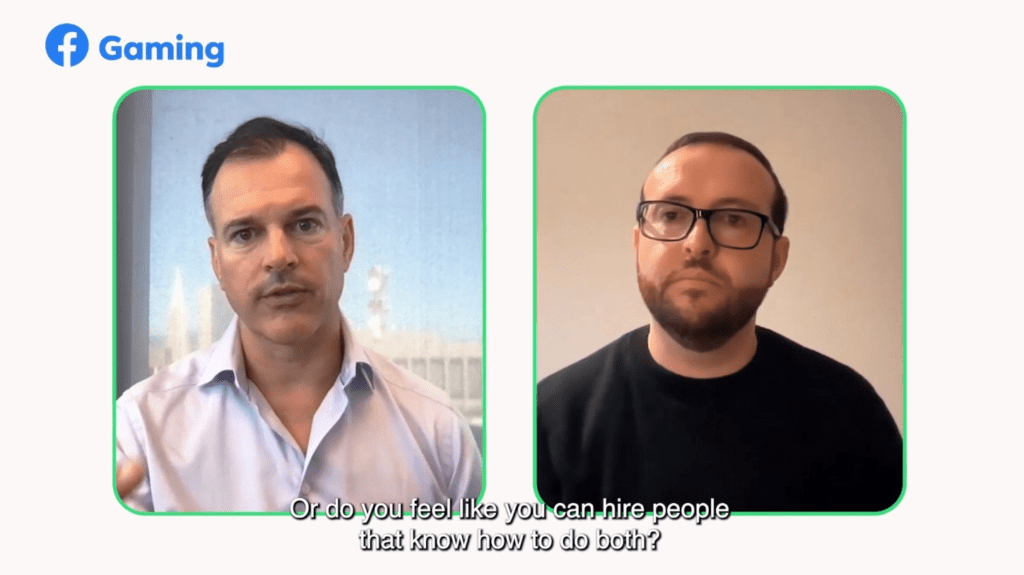
Making a distinction on talent between:
a) modeling skills
b) test design skills
You need to hire for both independently.
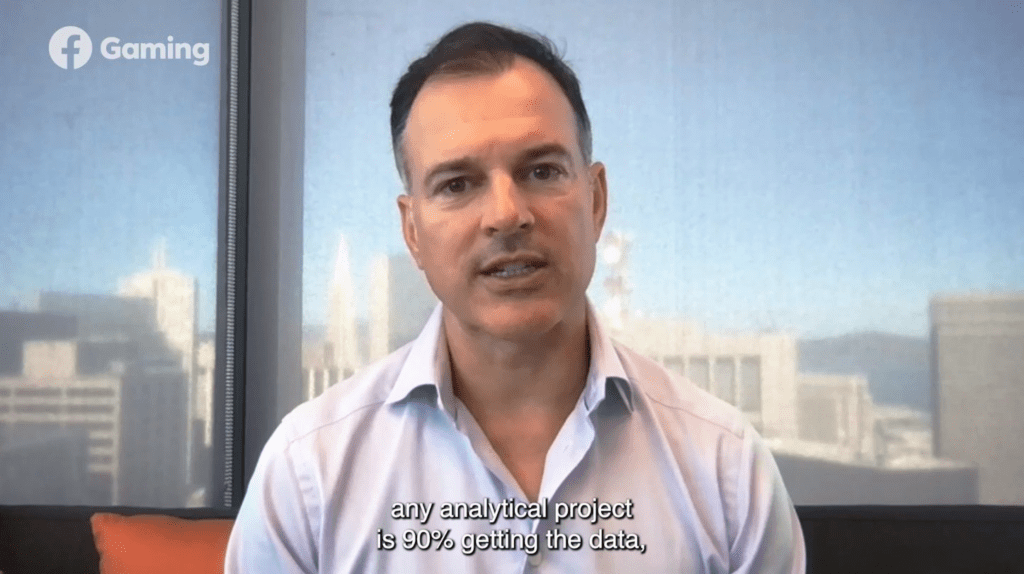
90% of any analytical project is getting the data in the right format. I find this often myself.
Stop Paying For Players You’re Already Acquiring
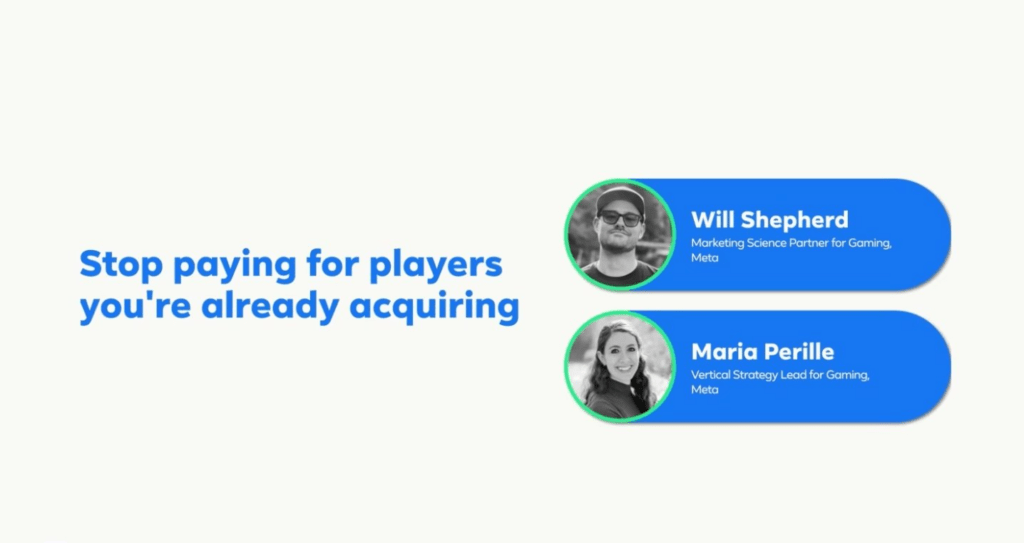
Ok ok last session, and you can stop thinking about statistics for a while. This is a session staffed by two Meta employees, and it touches on the main theme of the summit: incrementality.
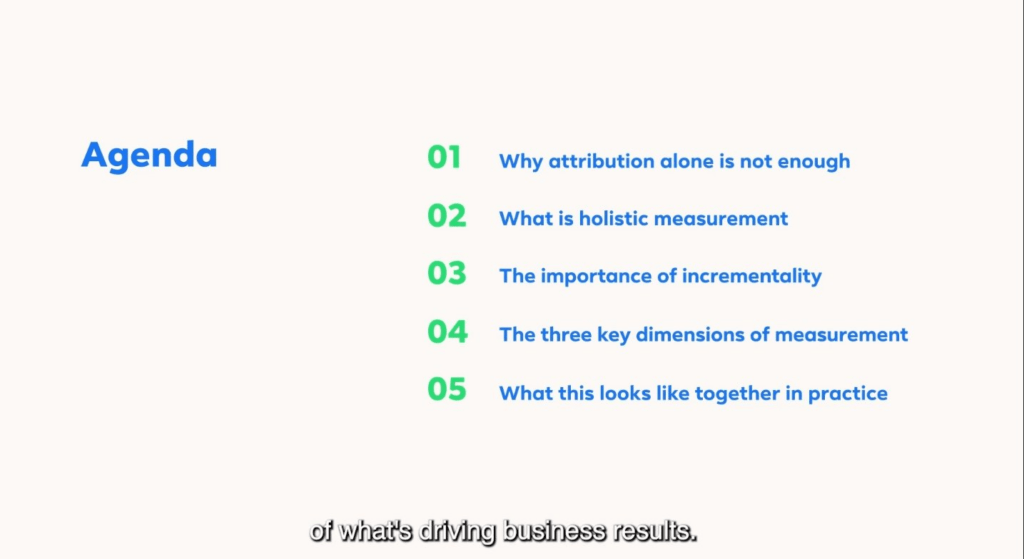
This one is a kind of roundup on how to operate in this new climate. Best practices for post-iOS14.
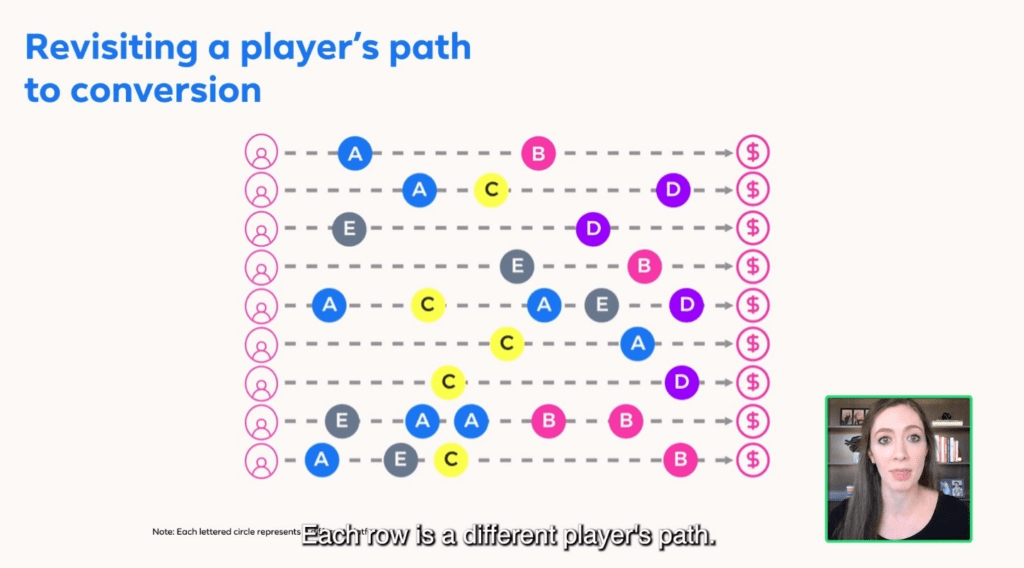
This is what it’s all about: every player (user) has a different path to conversion, and you don’t have a complete view of every path. Attribution is all about estimating what this really looks like.
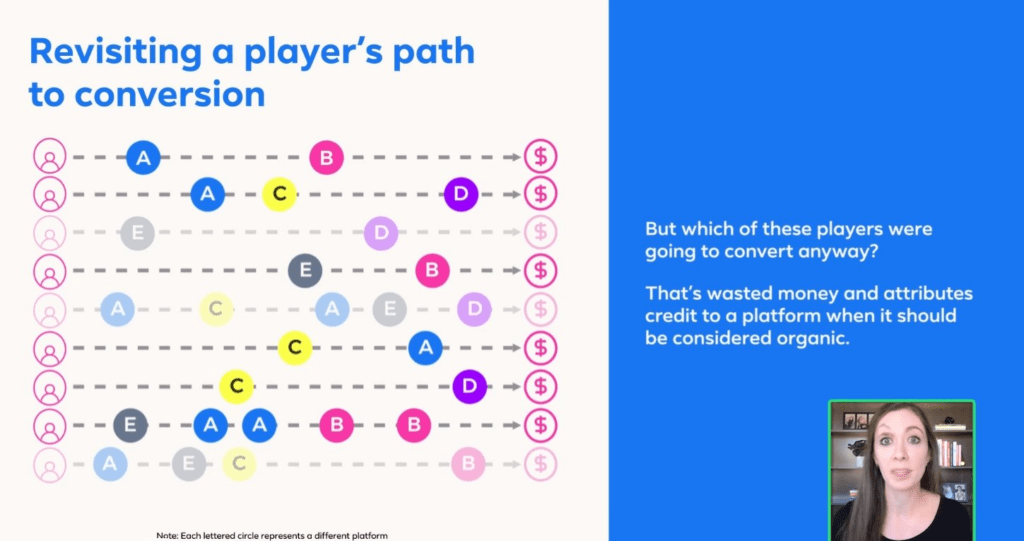
Incrementality is deducting the players (users) who were going to convert anyway, before you intervened by marketing to them.
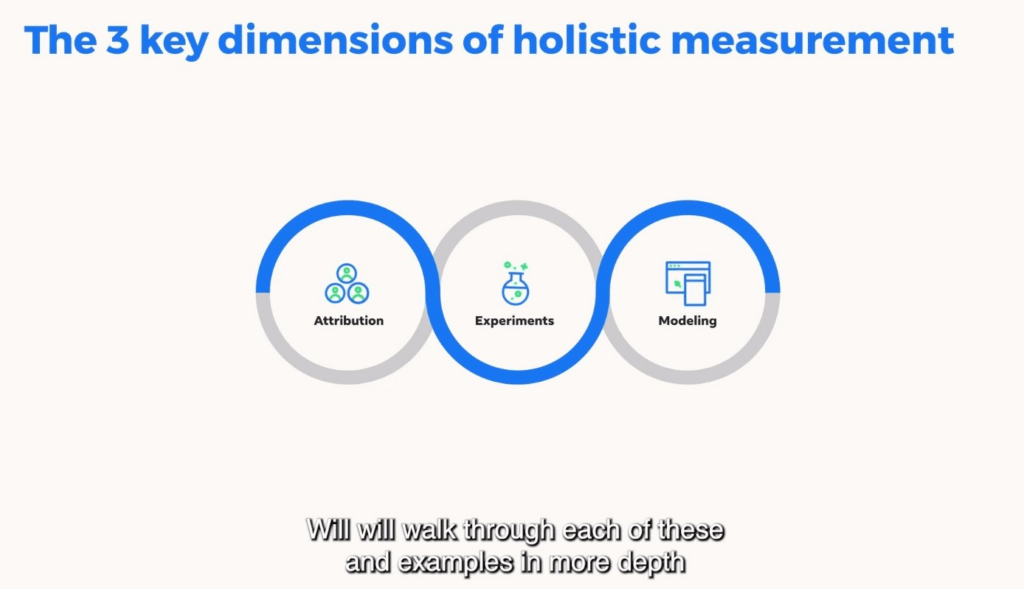
This is the standard triad of measurement methods that Meta talks about. In my opinion they’re missing a fourth: user surveys! We talked about that in Reforge in the Attribution Stack.
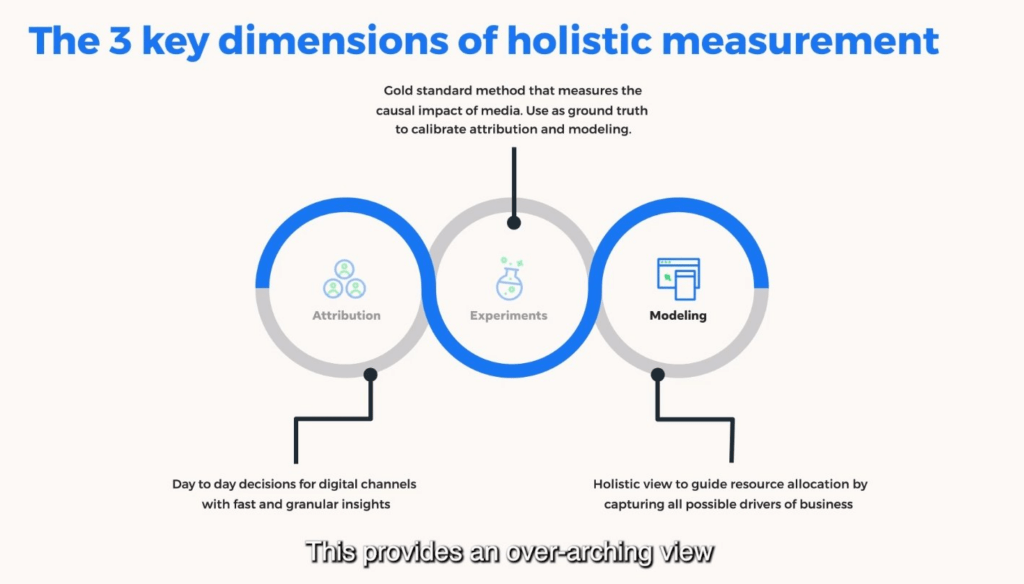
Nothing new here, but most marketers are still not there.
1/ daily optimization with attribution
2/ calibrate that with modeling
3/ calibrate that with experiments.
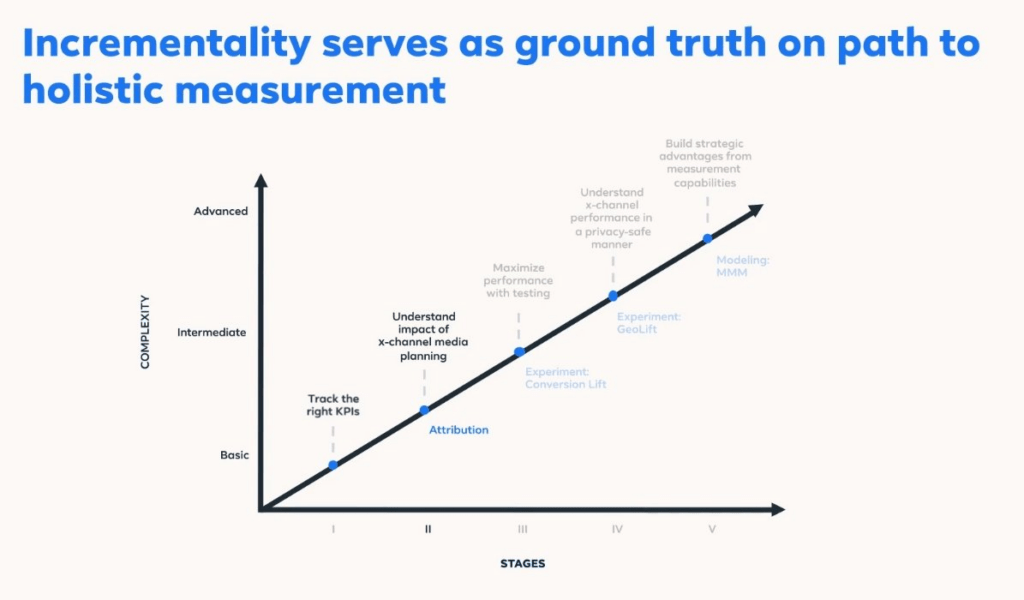
MMM at the top of the food chain 😀 Unfortunately that just means it’s the most complicated!
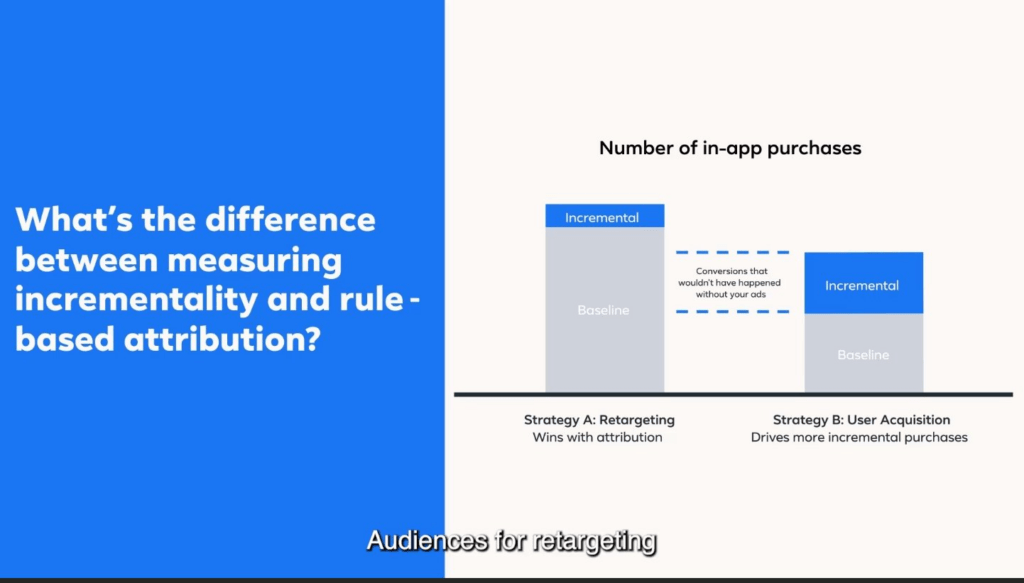
Great simple way to explain incrementality, and this is something I’ve seen time and time again. Retargeting gets good surface level results, but it’s not incremental because it’s targeting people who would have purchased anyway!
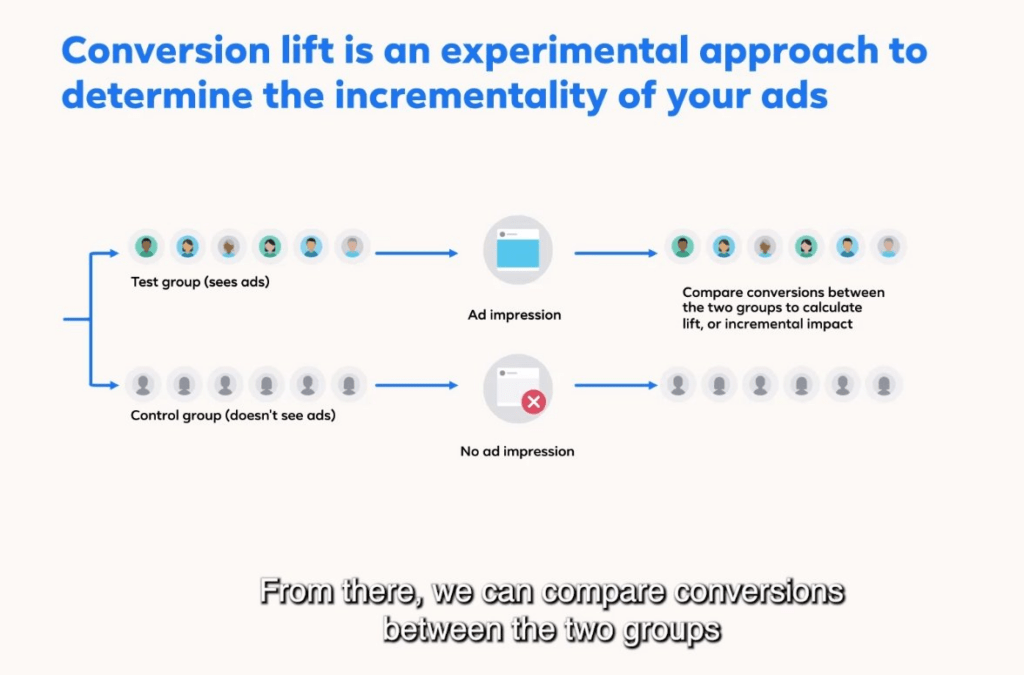
Meta always has a great slide on this. What a great way to explain lift tests!
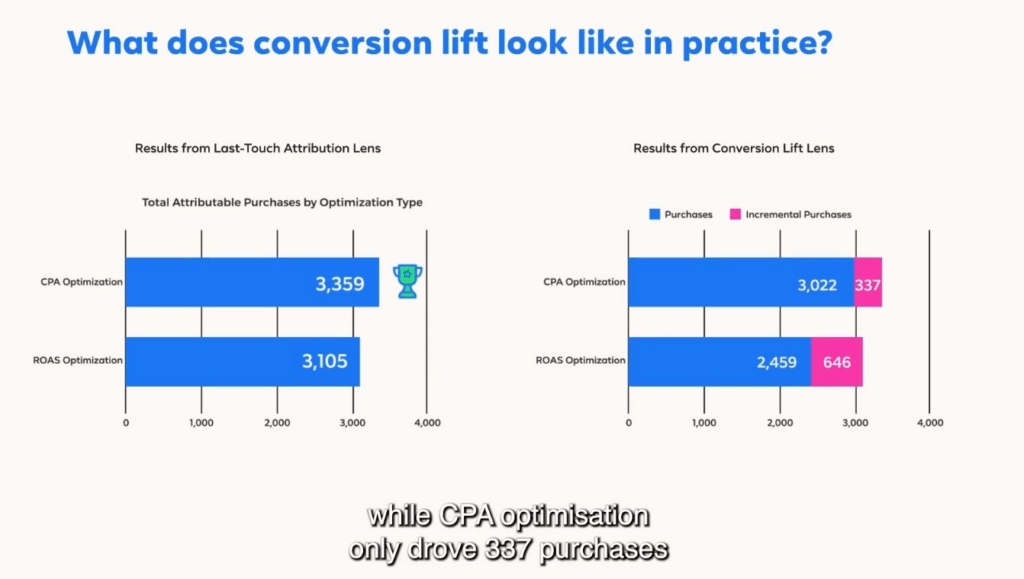
Here’s a cool insight: ROAS optimization actually drove more incremental purchases.
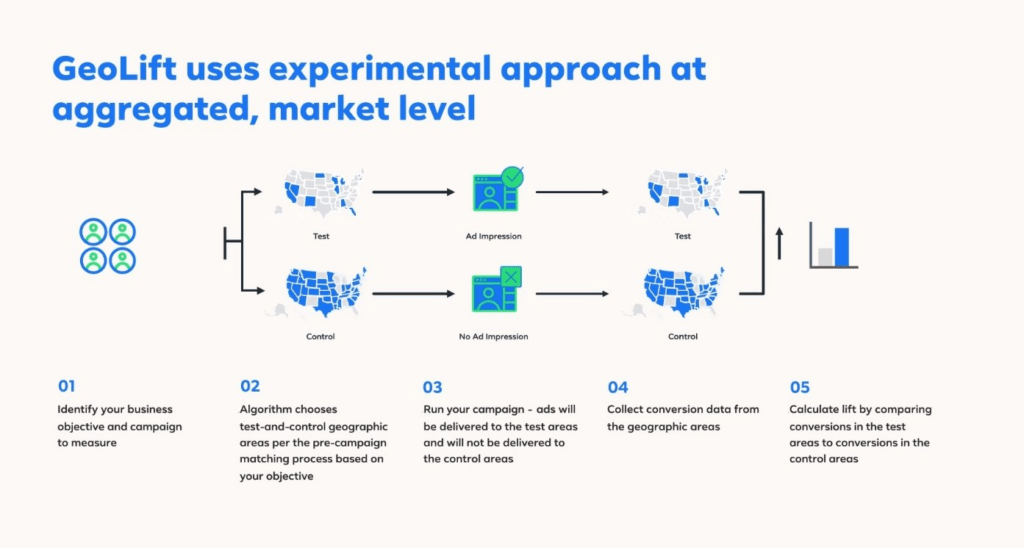
Meta is unmatched on these visualizations explaining complex statistical issues.
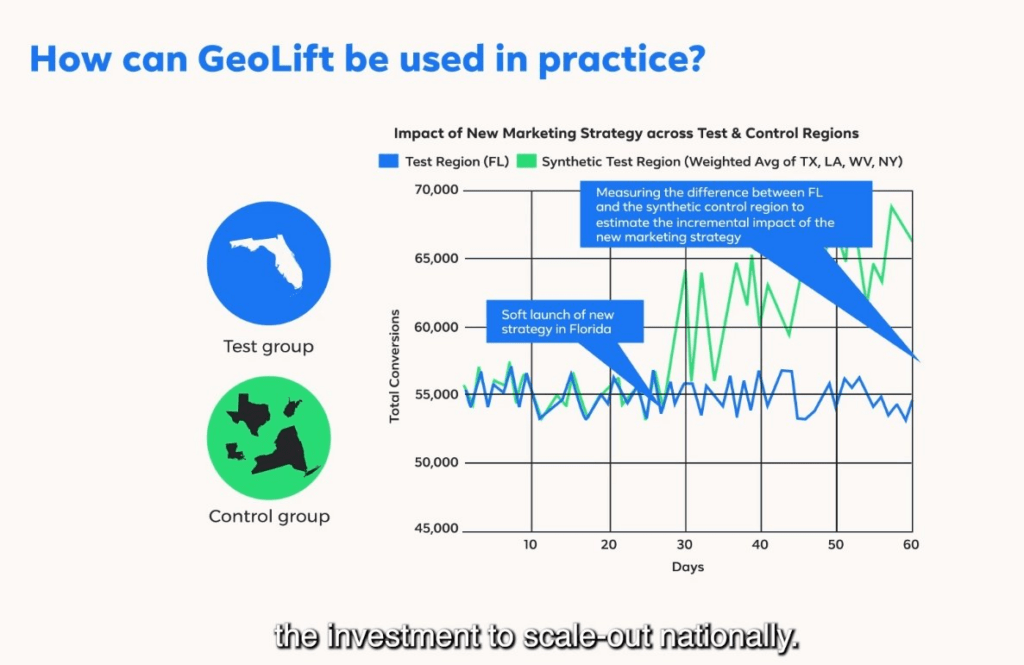
Before you’ve seen the results of a geo-lift test you might question the methodology. No statistics needed for this one: it’s such a clear huge uplift!
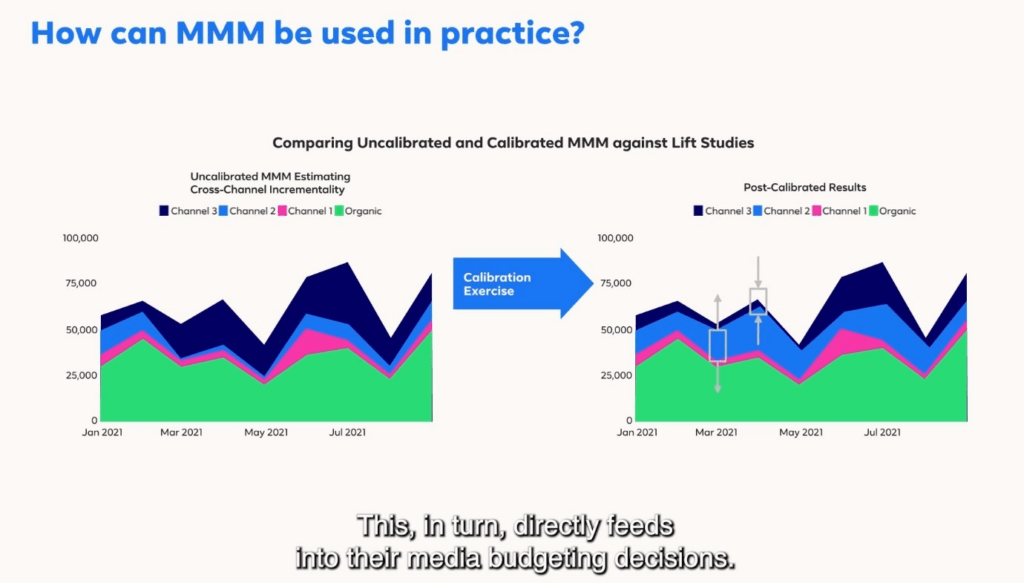
Here they’re showing in practical terms how calibration affects MMM: channel 1 was previously overstated and channel 2 wasn’t getting enough credit.
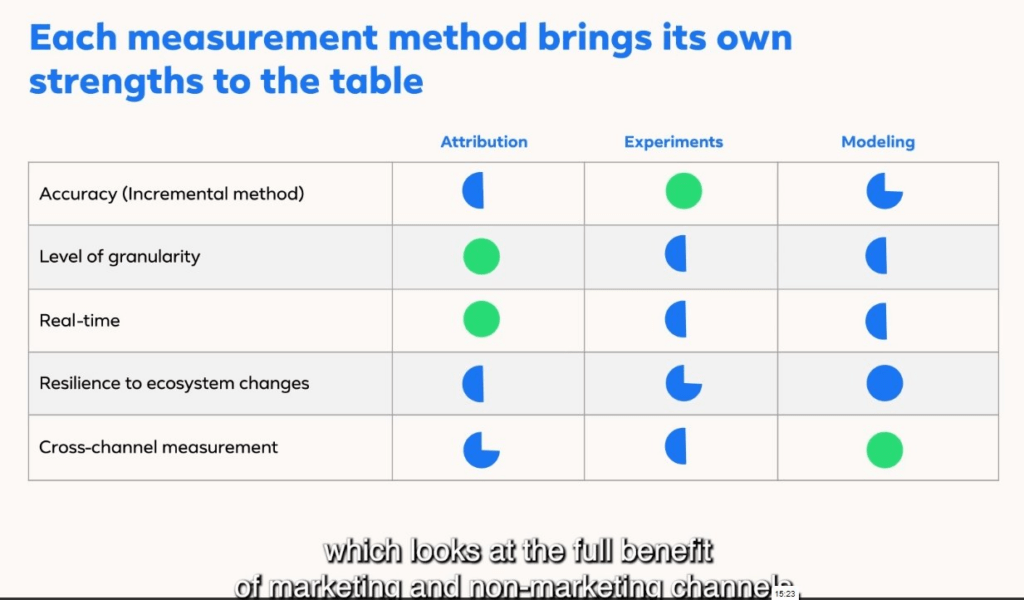
Funny, I basically have the same chart on my website.
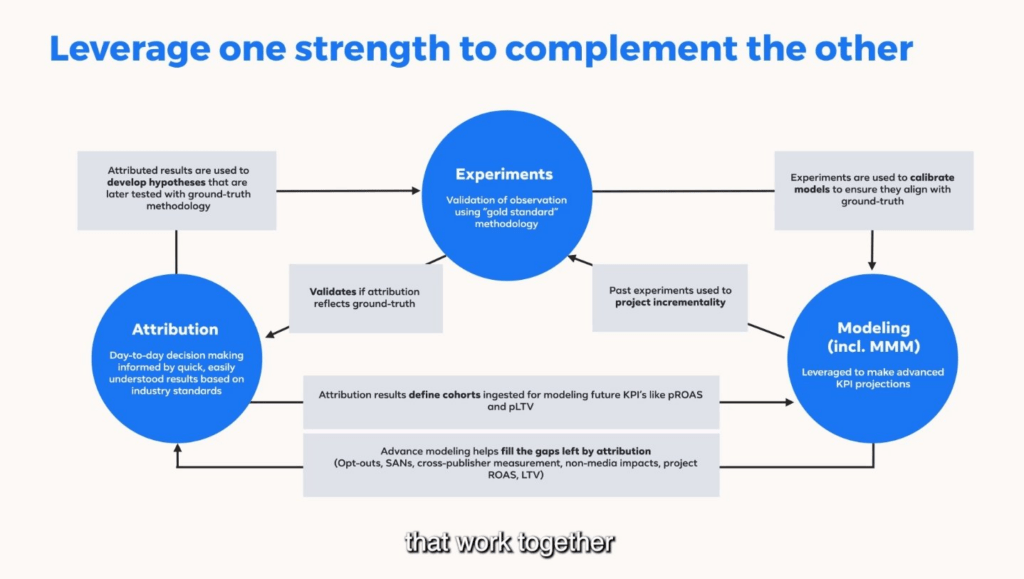
This looks complicated but it’s very worth ingesting. It’s how you can triangulate the truth between multiple methods and operationalize them in your marketing budget optimization decision-making.
Conclusion
That’s all folks! As it stands all the videos are publicly available on the event website. The big reveal was that MMM is the clear leader in terms of solutions to iOS14, with large double digit swings in interest in MMM amongst marketers. As with other summits by Meta, there were the common themes of combining MMM with Experiments and Attribution to triangulate the truth of what drives performance. We also saw a few interesting case studies which highlighted scenarios where MMM can help. For example channels that were overvalued can be cut, and channels with more potential can benefit from additional funds.