The Marketing Science team at Meta set the Marketing Mix Modeling world alight when they released Robyn, their open source solution. Entering the market at a time when everyone was scrambling to solve the iOS14 problem, helped to introduce the method to a wider audience, and validate that it was a reliable approach to use. Given the surprising level of interest the last time I covered Meta’s MMM summit, I suspected people would find it useful to cover round 2.
Meta opened the summit with a great piece of anecdotal evidence: 80% more companies they work with have adopted MMM since the last summit. Of course we don’t know the baseline – it could have gone from 10 to 18, or from 1,000 to 1,800 – but that’s refreshing news to anyone who has lived through an MMM industry in managed decline over the last 10 years.
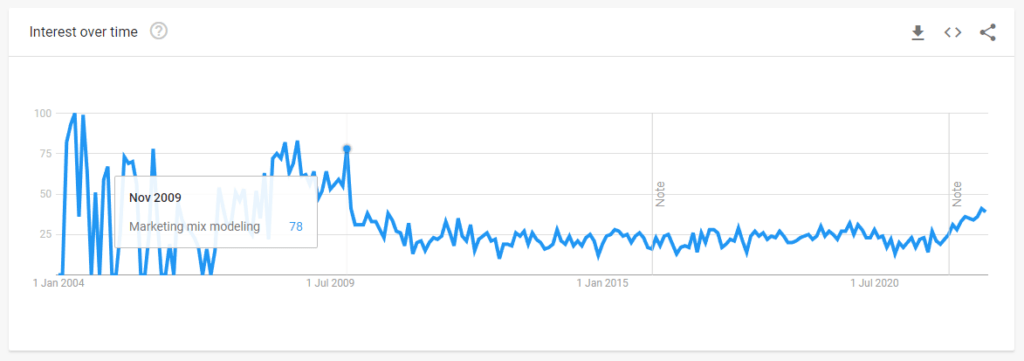
The agenda covered data granularity, advanced techniques including calibration, and innovation in the Marketing Mix Modeling field. They mostly gave the floor to partners such as Nielsen, Accenture, and Ekimetrics, as well as hosting a panel discussion by AppsFlyer, Adjust, Supermetrics and others incubating Meta’s open source project Robyn in their products. All of the panels were interesting, and while they promised they’d share the video on the event page after, I thought it’d be more convenient to be able to browse through a few highlights.
Next gen MMM: driving ROI optimisation in a privacy first world
The technique of building MMMs dates back to the 1960s, and honestly it hasn’t changed much since then, until more recently with Apple’s iOS14 update gave users the right to opt out of tracking. With digital marketers now just as in the dark as offline marketers, some of us started to look back and see what we used before to measure performance, back when there were no browser cookies or tracking pixels. MMM is a privacy friendly technique using only aggregate data, and it works holistically across every channel: which seems perfect… until you actually try and build one. It’s hard to build MMMs, which is why Meta’s more automated open source solution was so welcome.
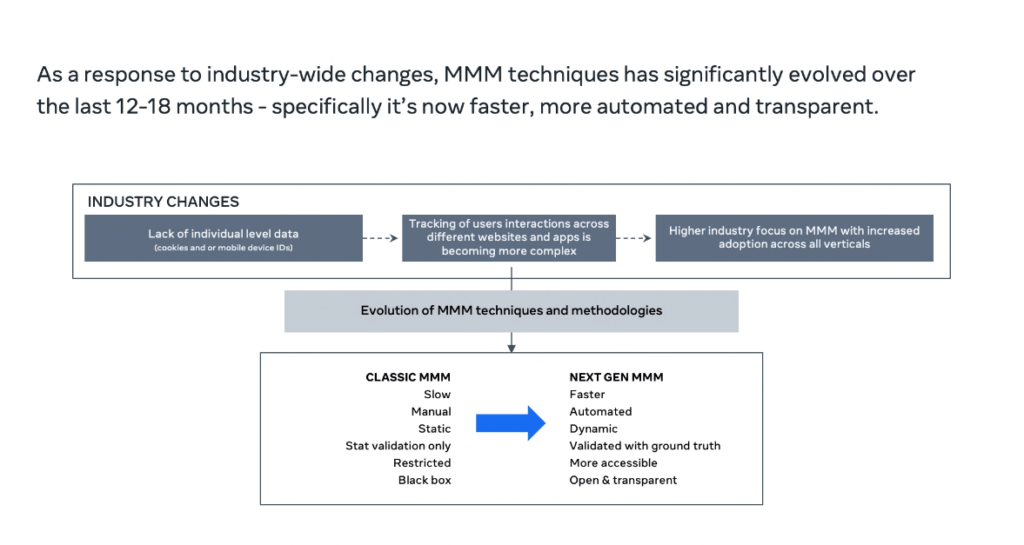
Whereas before MMM was a slow, manual process done once or twice a year at great expense, it has been reinvented for the modern age. Now data collection can be standardized and automated with API connections into your marketing platforms, and modeling can be (almost) fully automated, with insights delivered on demand. There have also been efforts made to make MMM less black box and more accessible to marketers, as well as adoption of calibration techniques to make MMM more reliable and robust.
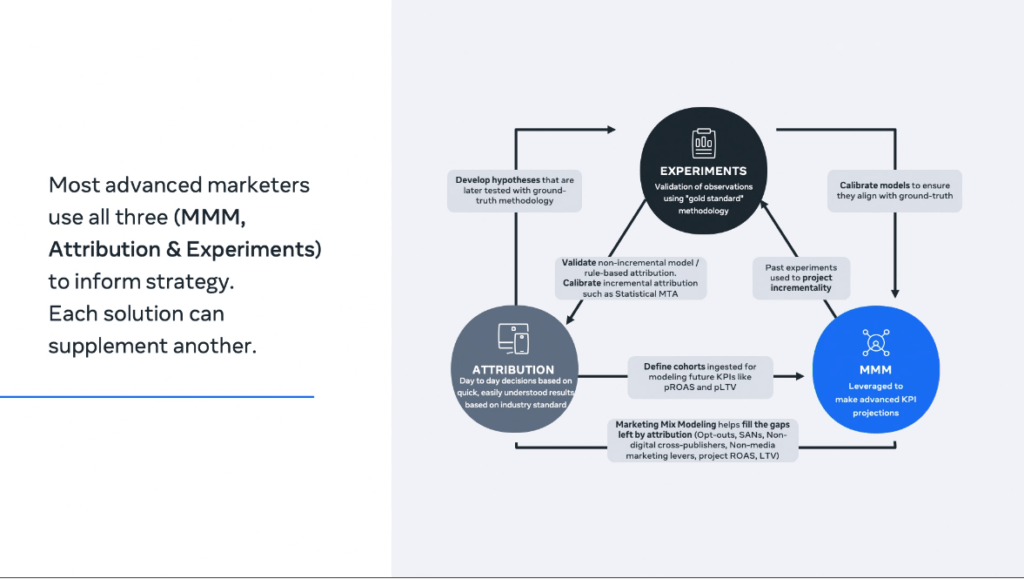
Almost every talk at the event talked about this modern phenomenon of triangulating the true answer to “what channels are performing?”, with modern teams using a mix of lift tests, attribution, and MMM to keep each method in check. This is something we’ve been big believers in as well, as can be seen by our post on Reforge about what to do about attribution after iOS14, though we’d expand that to a fourth pillar, surveys, which can offer a dose of common sense the other 3 methods can’t.
Self-service feed: MMM reports in ads reporting UI and MMM API
This was one of the few sessions by someone at Meta, and it was focused on Meta’s MMM feed, which I was surprised to find out had been in operation for 4 years! Collecting and cleaning the data can be 60% of the job of building an MMM in my experience, so it’s great to see work in this area. They built a very opinionated feed based on modeling best practices, so I would say this is ‘best in class’ and something for other channels to emulate.
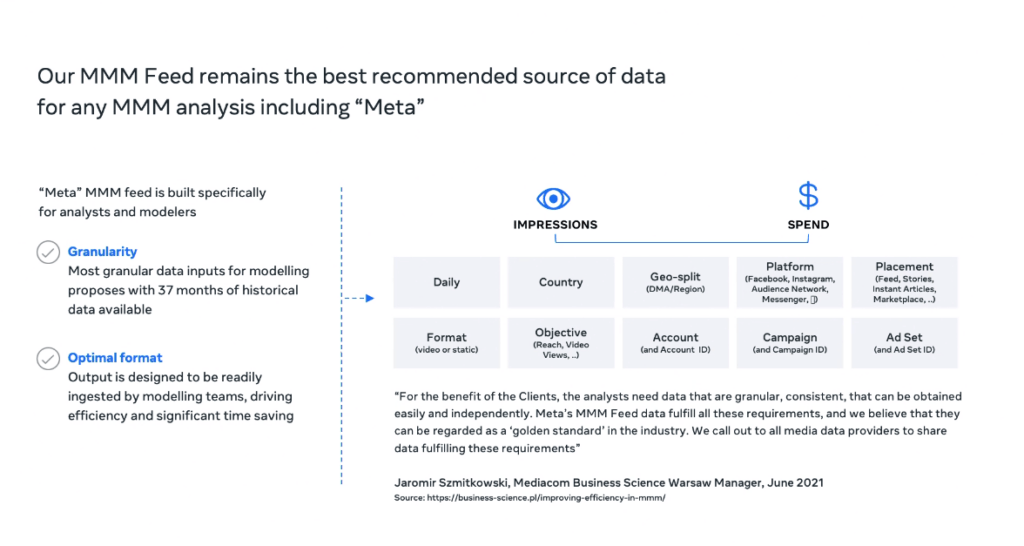
They listed all of their ‘badged’ partners which include mostly big consultancies. Not much relevance for all but the biggest MMM clients. No clue how to get into this list (I’ve tried with a few different clients I was advising who offer MMM) and was told by different Meta reps it’s “closed”. Robyn is Meta’s open source library and that team has been much more open (as you’d expect), and are extremely active in their Facebook group and GitHub repository.
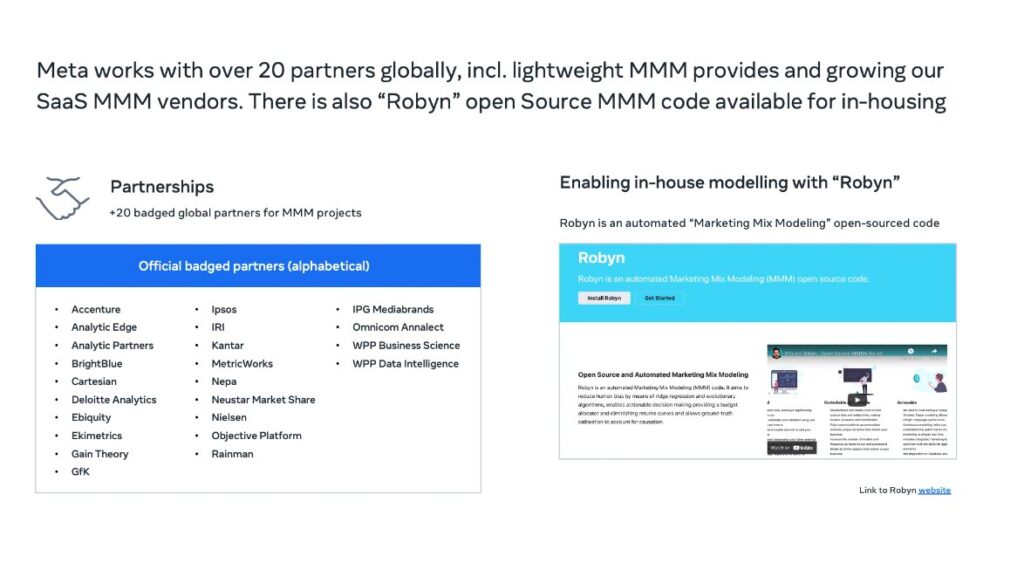
It was interesting to see the actionable tips Meta provides, as this is something I think they do extremely well. You get the sense they actually are working closely with partners to disseminate these best practices, which is sorely needed in a sometimes murky industry like MMM. They point out that breaking down to campaign level is important, but actually in my experience it can really throw off the model: granularity isn’t a solved problem within MMM. When using Robyn I’ve seen many campaigns go to zero in terms of impact, when I include too many. I do like the idea of standardizing approaches across a portfolio of clients for MMM, that should help address the cost issue, and should also help remove human bias.
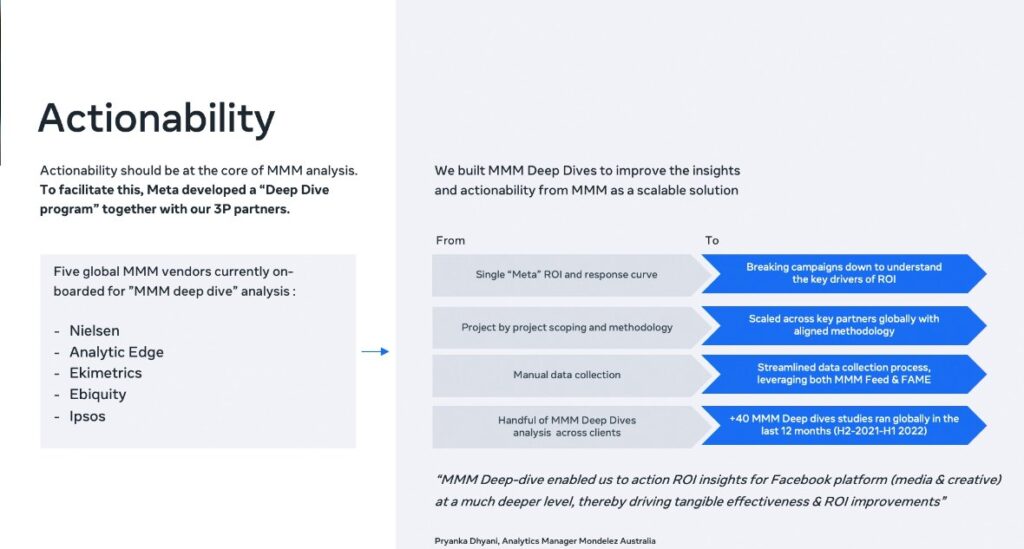
Calibration is the most important movement in MMM best practice. Previously models weren’t calibrated at all (most traditional vendors aren’t even doing a train test split in my experience!) and I think this is the biggest single thing we can all do to improve trust in the method. Mostly the issue with MMM is that it can produce ‘implausible’ results, and calibrating the model with the results of experiments can help move us towards more realistic recommendations.
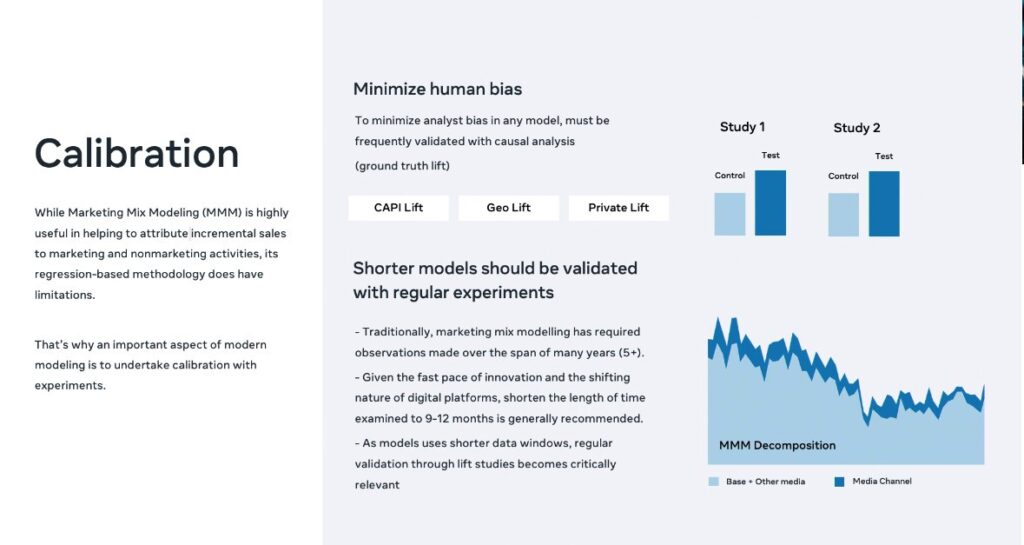
The big announcement here was that next year everybody is getting an MMM feed! They’re releasing the tool to the public both via CSV download and API. This will introduce 3 million advertisers to the word MMM, and would make it more accessible than it is currently, which would be a huge deal.
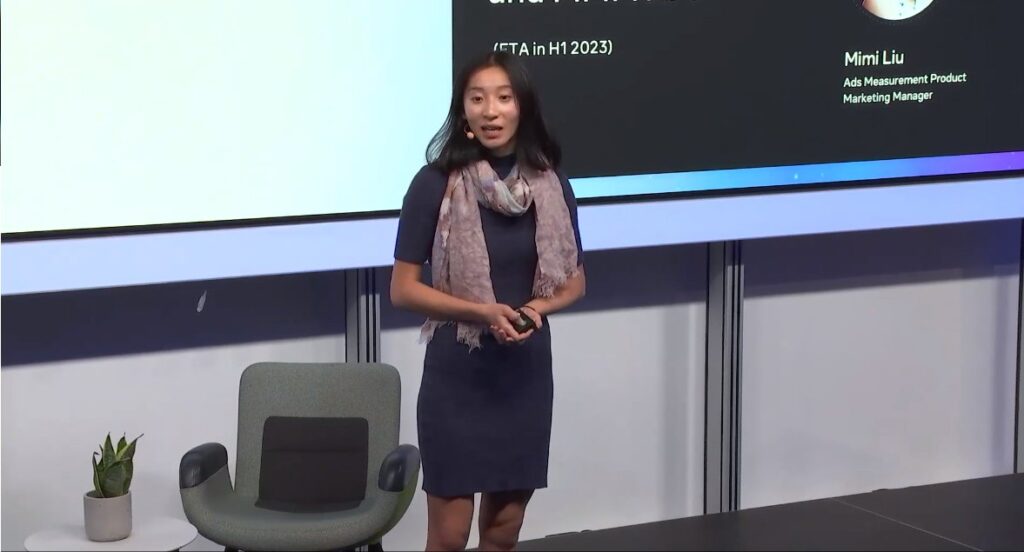
However good this is for Meta advertisers and professional modelers, it is an incomplete solution. It’s nice that Meta is keeping their data in order, but unless other advertisers follow suit, we’ll still be spending a lot of time and effort cleaning data. What we really need is an MMM feed from cross channel ad metric aggregators like Supermetrics and Funnel.io.
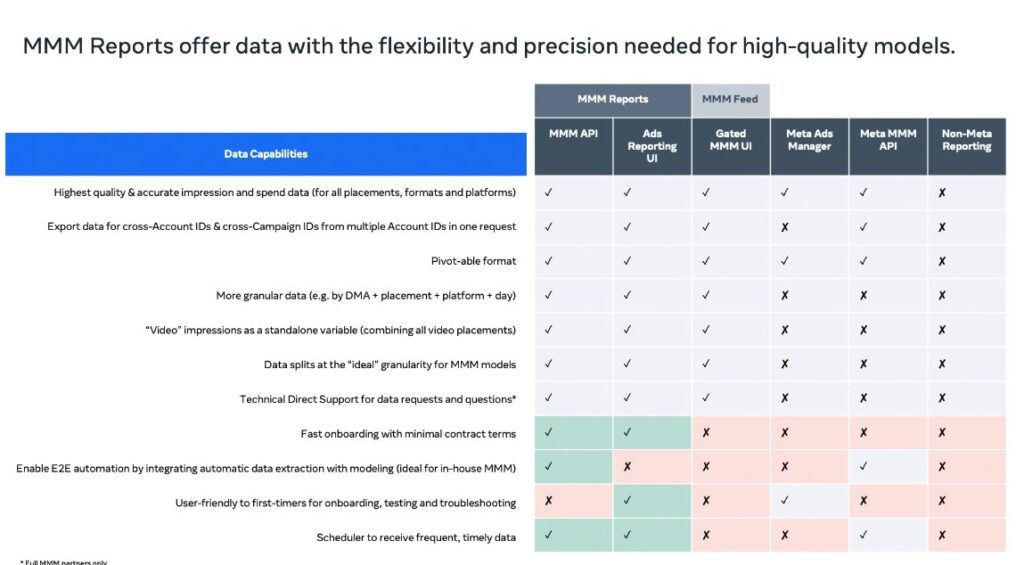
One other thing I found interesting in this talk is that they are providing geo level breakdowns. As far as I’m aware Meta doesn’t include the capability to do Geo-level MMMs with Robyn. In fact it’s one of the major design differences between their solution and something like Google LightweightMMM which does. Perhaps geo-level modeling is on its way?
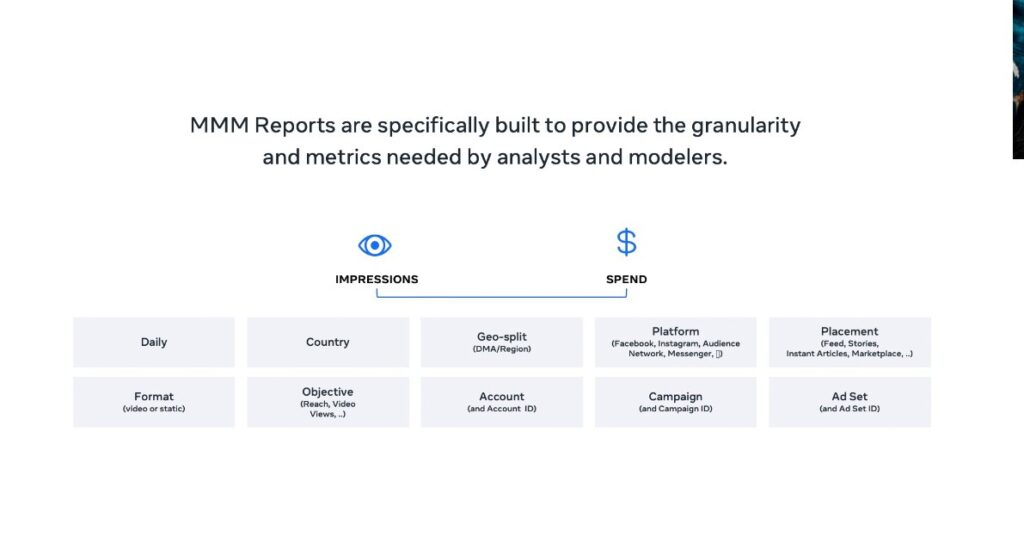
They finished up on some screenshots of the new reporting tool. Nothing unexpected here: it just looks like a normal ads manager report.
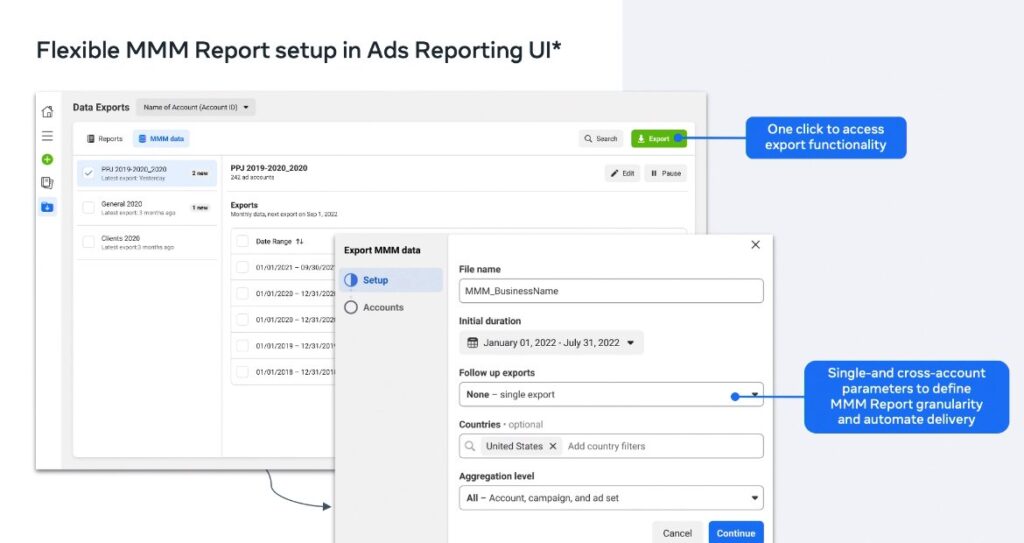
ROI @ the level you plan & buy
Next up was Nielsen. They kicked off by saying the previous iteration of MMM was about allocation between channels, but now their clients are demanding more granularity within channels. That seems to be the overriding concern of a lot of MMM service and tool providers throughout the conference.
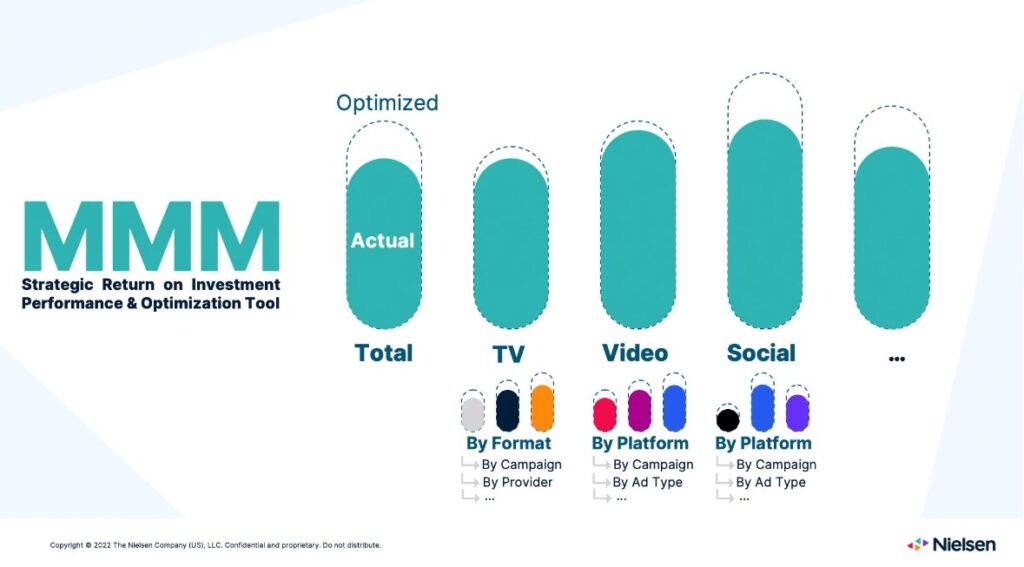
They did a comprehensive study of 2,220 clients across countries, and found that in some cases ROI was significantly higher on Meta ads than others. Not that surprising as a finding to be honest, given that there will always be natural variances in the relative performance across clients. Useful to know however that if your performance is below average, you could potentially have the headroom to triple or quadruple it.
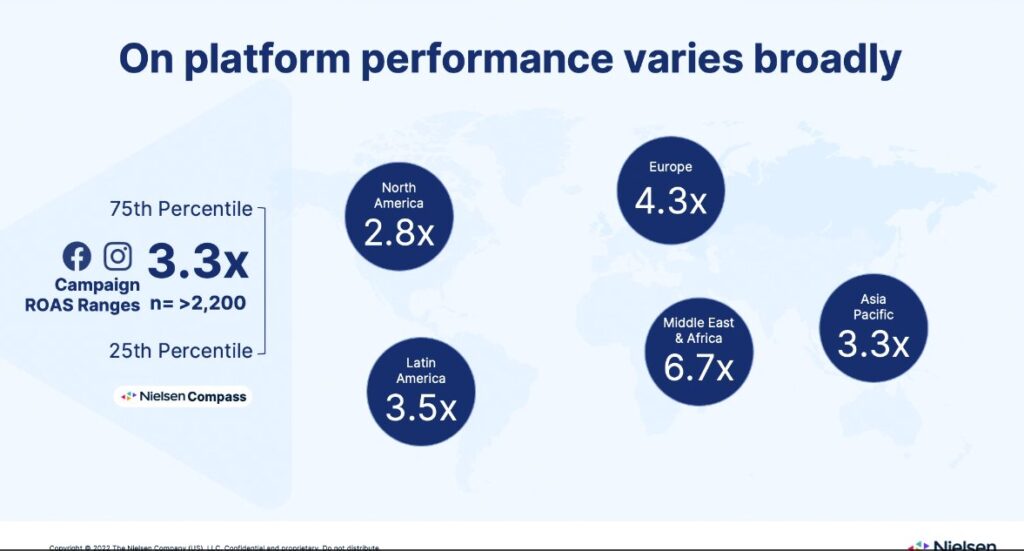
They provided a good breakdown of the strategic versus tactical decisions a media buyer has to make for a successful campaign. Super weird there’s no mention of creative though! It’s the biggest lever in my experience (confirmed by multiple studies, and written about here).
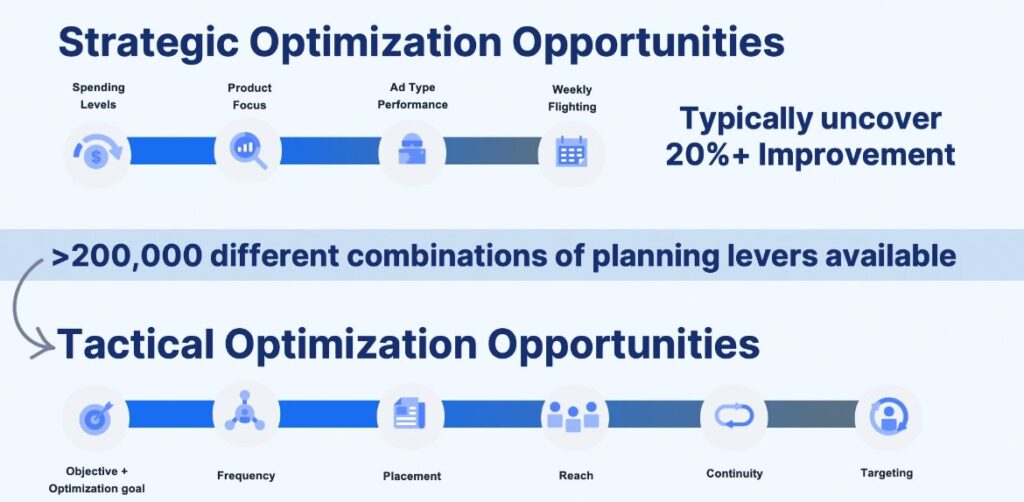
In the next slide they do mention creative excellence, but as one of the things that make up at least 50% of performance. No idea how relatively more or less important they found creative to be, as they only break down execution tactics.
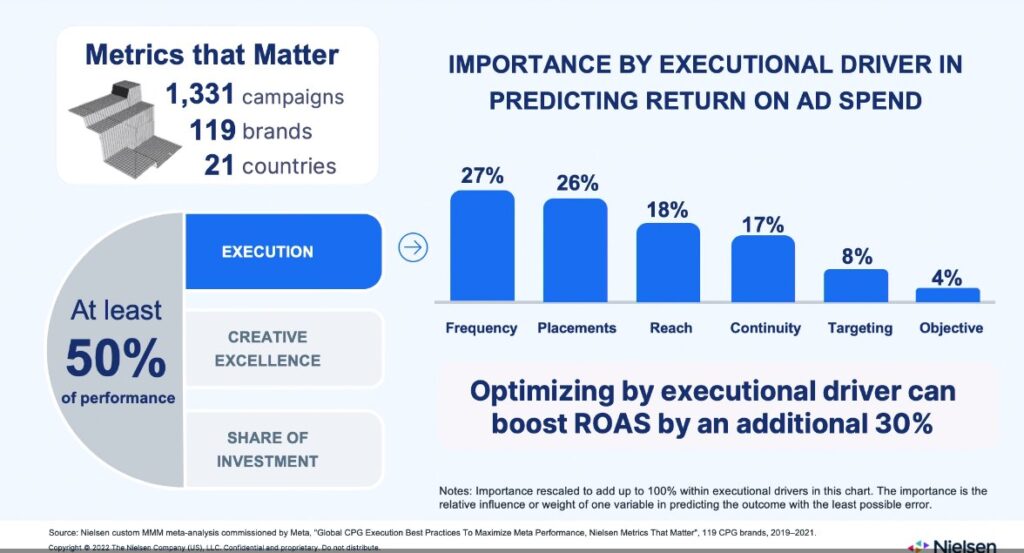
Confusingly they say that the benefit of improving these execution issues is only a 40% gain in ROAS… if the gap was 2.8x in North America in the previous slide, then this is relatively inconsequential. They say that the following tactics are what work:
- keeping your frequency to 1.5x to 2x per week
- running on all placements
- reaching 11-20% of the population
- broad targeting
- keeping your creative continuous
I couldn’t disagree more with some of these, in particular creative. You stand to lose a lot of money if you adopt these ‘best practices’. Though I’d say this probably only applies to big brand advertisers of the likes that can afford Nielsen. For example you can only control frequency when buying reach and frequency campaigns, so this doesn’t apply to performance marketing.
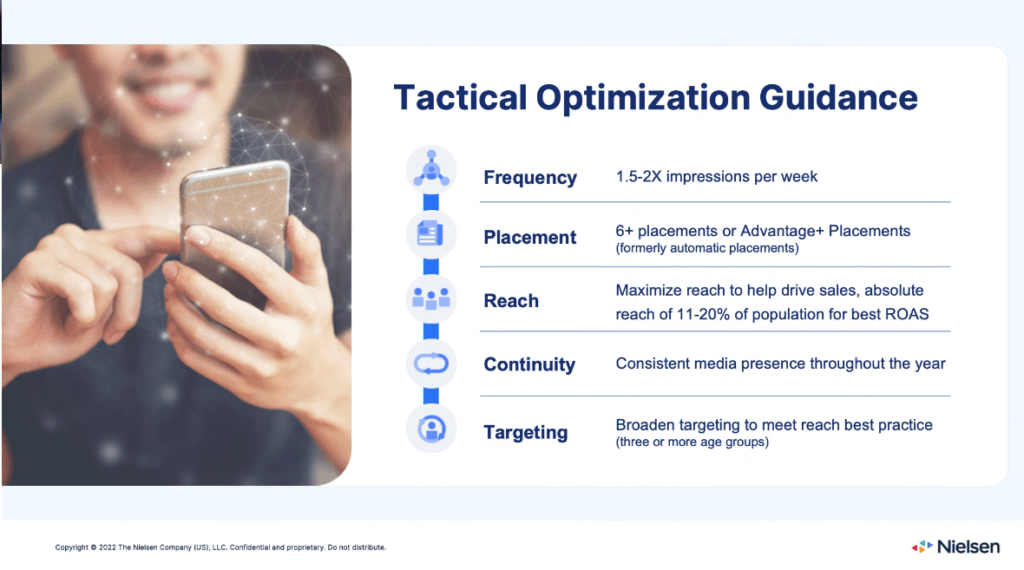
For some reason they buried the lede in this presentation, because when I checked out their study on their website, the number one factor driving performance is creative! No idea why they didn’t talk more about it.
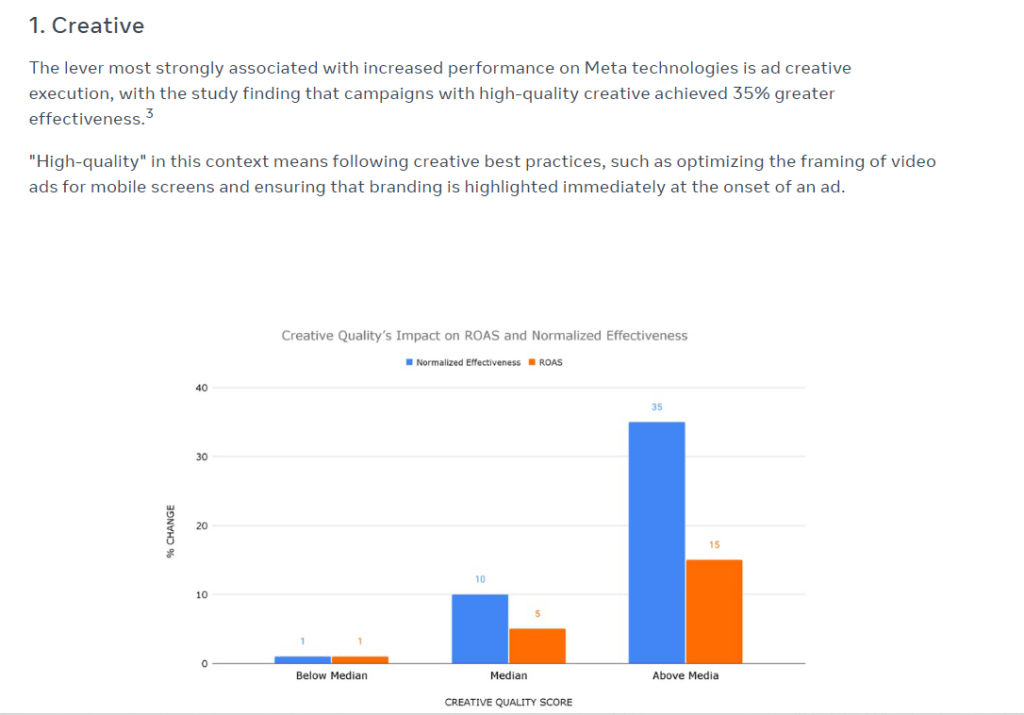
A new day for MMM
Now we’ve got Accenture on stage. They’re claiming typically it improves Marketing ROI by 14-38%, which is consistent with my experience too. I laughed out loud when Paul said “It used to be that you didn’t use MMM if you didn’t have to”. Very true, in many ways (pre-iOS14) it was an unforgiving technique, and was only really done for brands that had to, because they were running offline campaigns.
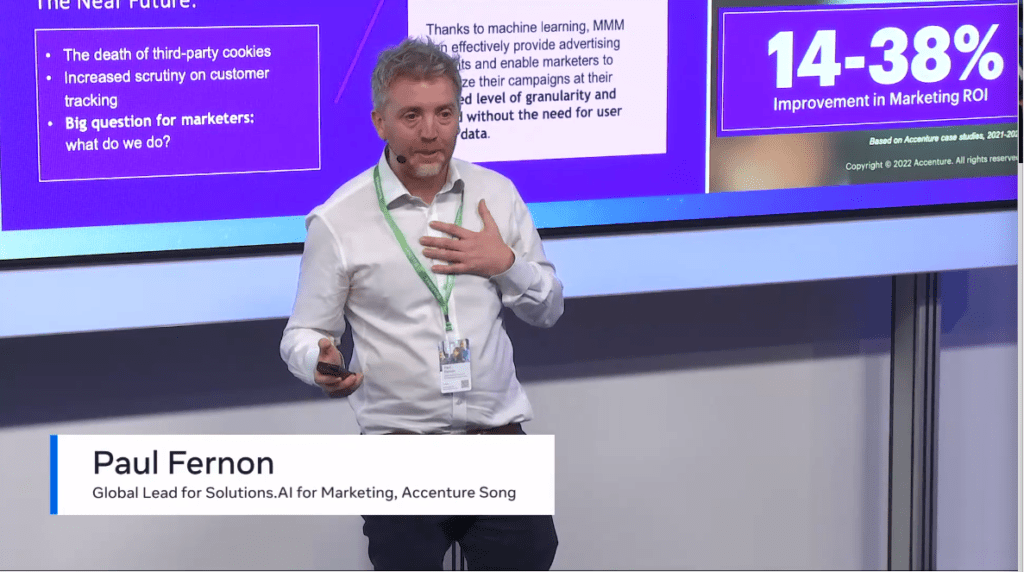
One thing that stood out, is that it took them 3 years to compile & clean the data for 30 advertisers! Just shows how much of a pain that part is. They mention Bayesian Belief Networks, which is some new lingo to me. Weird to mention Bayesian MMM at a Meta event, when they famously don’t use Bayesian approaches for their MMM tool Robyn.
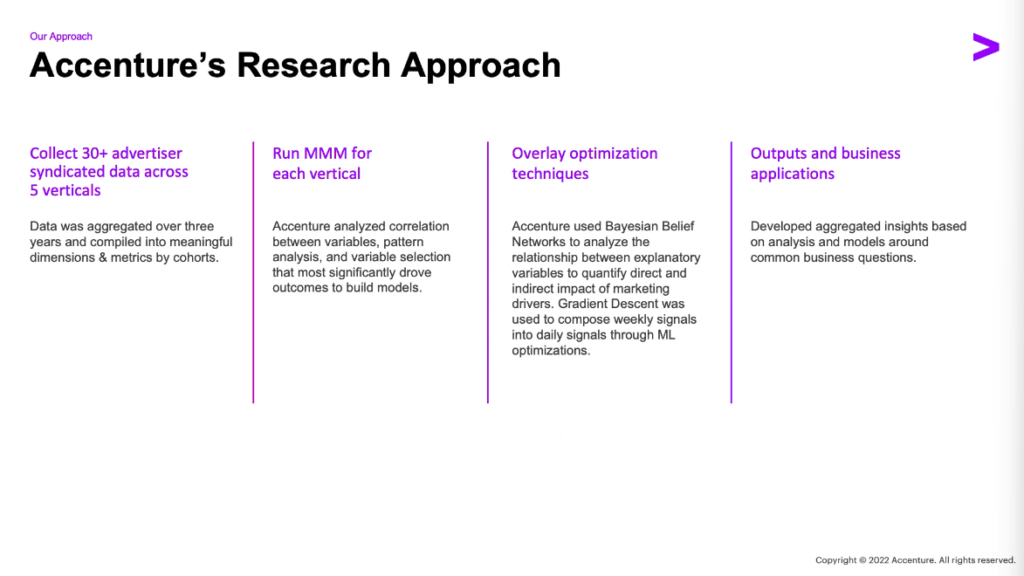
They’re saying MMM has progressed from a “dark art” to something more accessible, thanks to open source libraries and the use of APIs to be able to pull data. A breakdown on why Accenture believes MMM is more accessible now.
1. Cheaper compute
2. Access to data via API
3. Open source packages (i.e. Robyn)
4. KPI customization (no idea what this is)
They establish an industry standard measure of <5% MAPE (an error of measure) which is very useful. I’ve been told anything below 10% is acceptable, though it matters a lot whether they’re using in-sample (data the model has seen) versus out-of-sample (data the model hasn’t seen yet). In general error isn’t a perfect predictor of model usefulness however, because it’s perfectly possible to make an ‘accurate’ model with completely implausible assumptions and results.
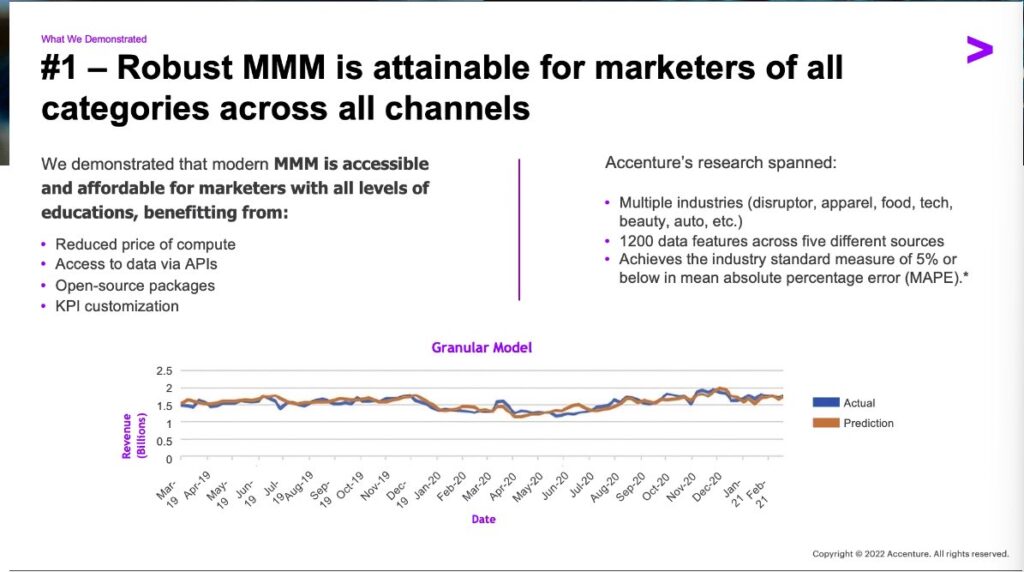
Granular data outperforms non-granular for ad optimization. It’s worth 0.11 – 0.28 more points of ROI to get that granularity. What they leave out here is how much harder it is to get a granular model, and how much granularity affects the plausibility of results. Often stuffing more variables in can harm interpretability and give you nonsensical results, because many campaigns are correlated with each other and tough for the model to tease out.
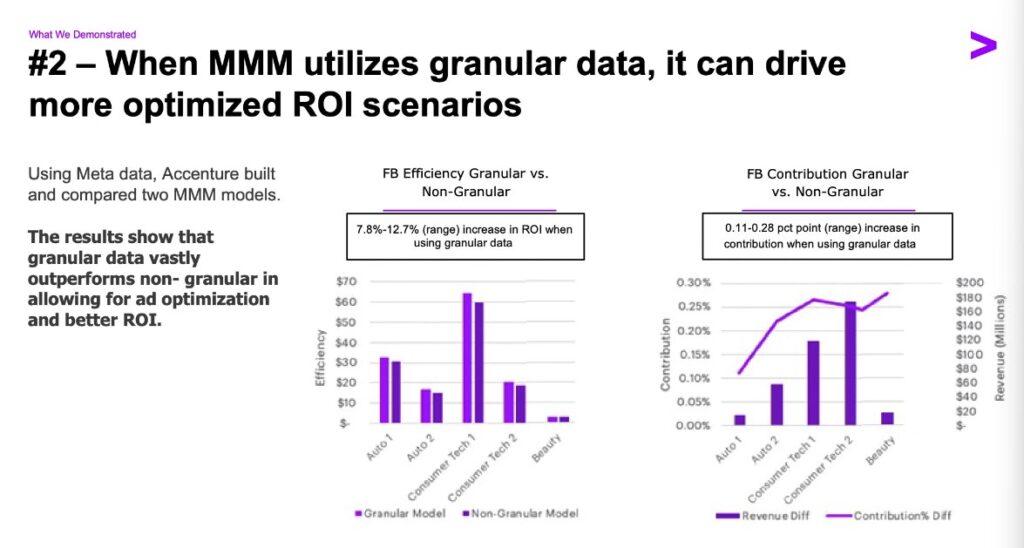
This part is very interesting as I’ve never heard of it before, and it looks promising. It seems like they’re modeling dependencies between channels here, with a Bayesian model. Note this isn’t close to possible in Meta’s Robyn tool, so it’s kind of odd to me that they’re letting a partner talk about it at their event. Anyway if this really does work, it looks like a powerful new way to do MMM, so I’m eager to learn more.
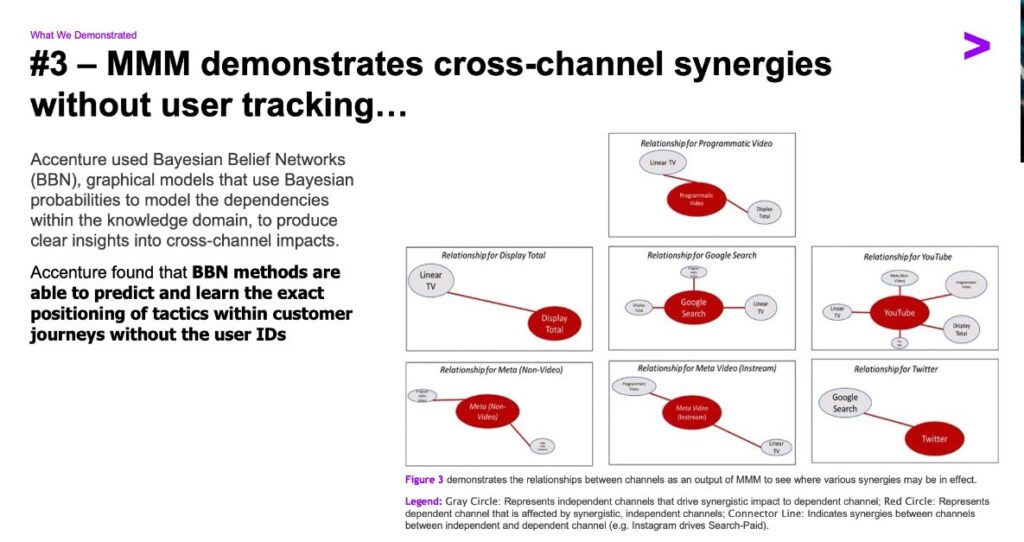
They used their Bayesian Belief Networks to show the interaction between channels here. However something doesn’t add up to me. For example ‘Direct’ isn’t really a ‘channel’, it’s lumped together traffic from other channels. It also just mostly says that spending on a channel drives a lot of activity on that channel. Not that useful (unless I’m misreading). It would be cool to learn more about this technique, if it can really offer user paths without tracking clicks.
Why calibrate MMM with lift experiments?
We’re back with Analytic Edge, one of the great winners out of the Meta MMM bonanza. They got in early and deep with the Meta partnership, and has earned a lot of media.
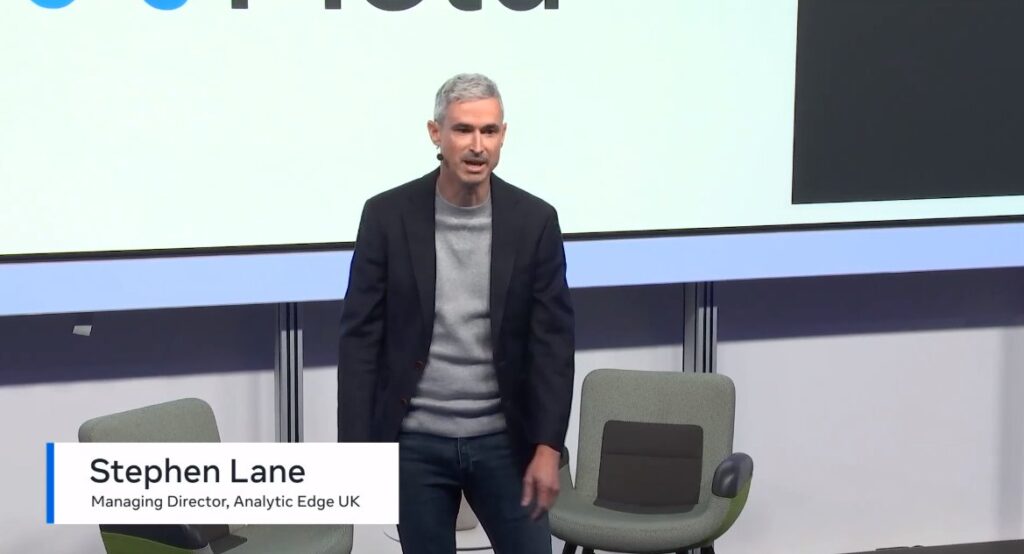
The main thing they’re looking at are MMM projects where lift studies accompanied them for calibration. Note these lift studies weren’t used in the MMMs at the time, they just went back historically and found instances (with Meta’s help) where lift tests and MMMs were both available around the same time, so they could see where they disagree, and if it was possible to rebuild the models using calibration.
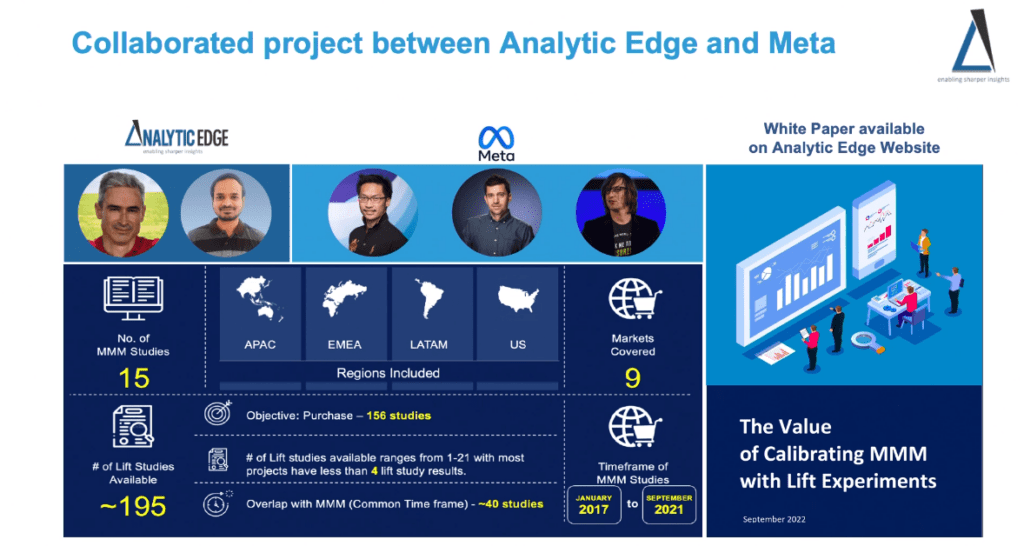
The main finding was that if you rebuild an MMM using the lift study to calibrate, in 2/3rds of the studies it improves accuracy enough to get you 25% more ROI (estimated). The rest of the time calibration doesn’t help. Neat insight!
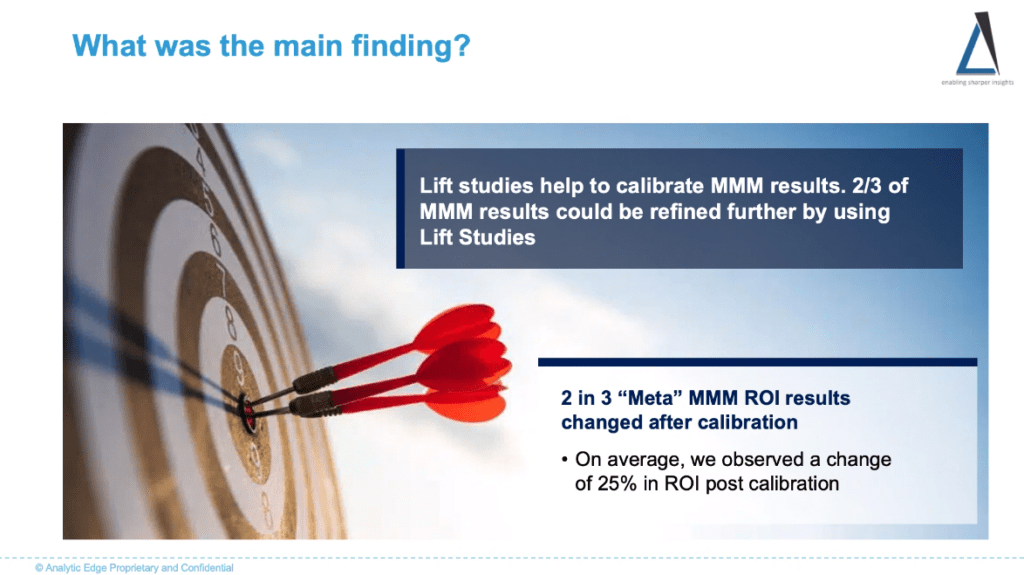
One really important slide was this one, about all the reasons why lift tests might sometimes not be ‘ground truth’. Most people do treat them as gospel when calibrating MMM, but like any attribution method they can be wrong too. For example if the lift test was for a few months ago, performance might have changed since. Additionally if you have a long purchase cycle, lift tests might not have run long enough to capture the full lagged effect.
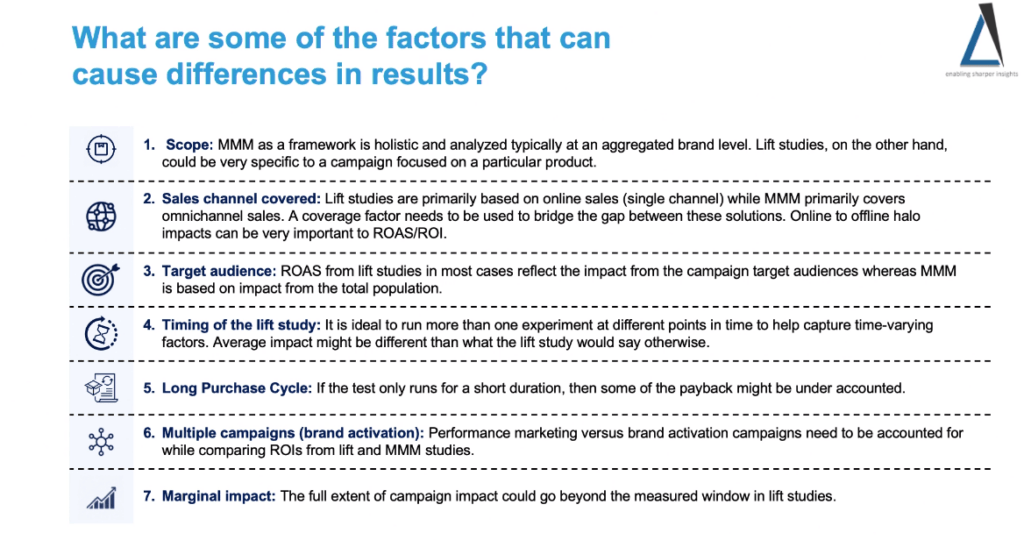
This is advanced: if they have multiple lift studies that disagree, they essentially build the model with different weights for each study, and test what gets the best fit with the data (basically hyperparameter optimization). This is a great approach if you have multiple lift tests, but in my experience the more common problem is that they haven’t run any lift tests at all.
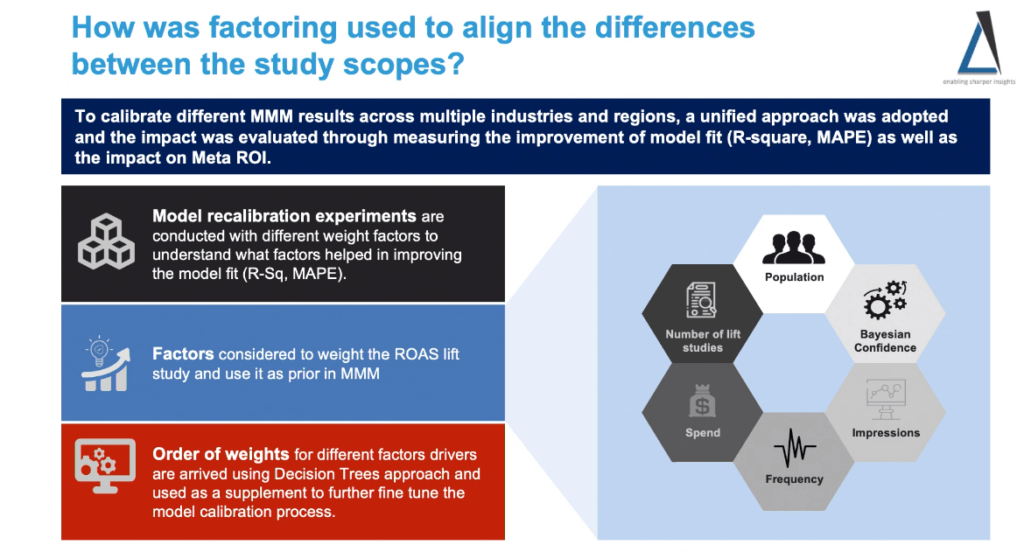
When they have weighted the lift tests, these are the factors they found to be most important. Mostly the coverage of the test was important, as well as the frequency. Carryover effects and seasonality also had a decent sized impact on whether a lift test could be trusted. Getting the right lift test can be worth 2-10x better calibration.
Building a MMM Saas solution: opportunities & challenges
These are Meta’s incubator program participants. They don’t say much about what that means, though I assume they’re companies building products using Robyn? Anyway it’s a strong panel of smart people, including three of the most powerful attribution tools in the market, AppsFlyer, Adjust, and Branch, as well as the market leader in data aggregation, Supermetrics.
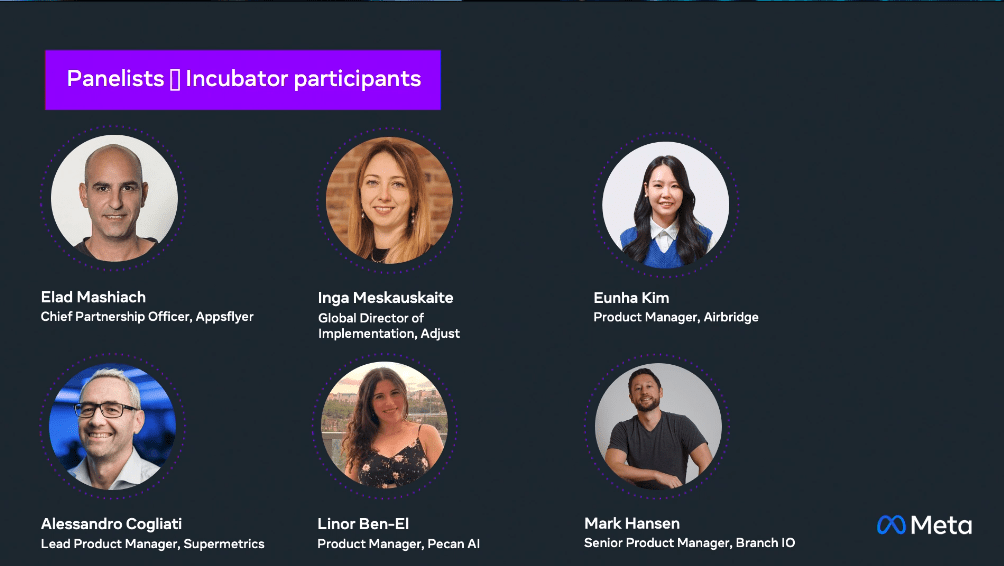
This details the program which seems to dictate a few things they need to do to get Meta support for their project. None of these are too restrictive, and this strikes me as a good approach for anybody testing out MMM. They don’t even demand you use Robyn!
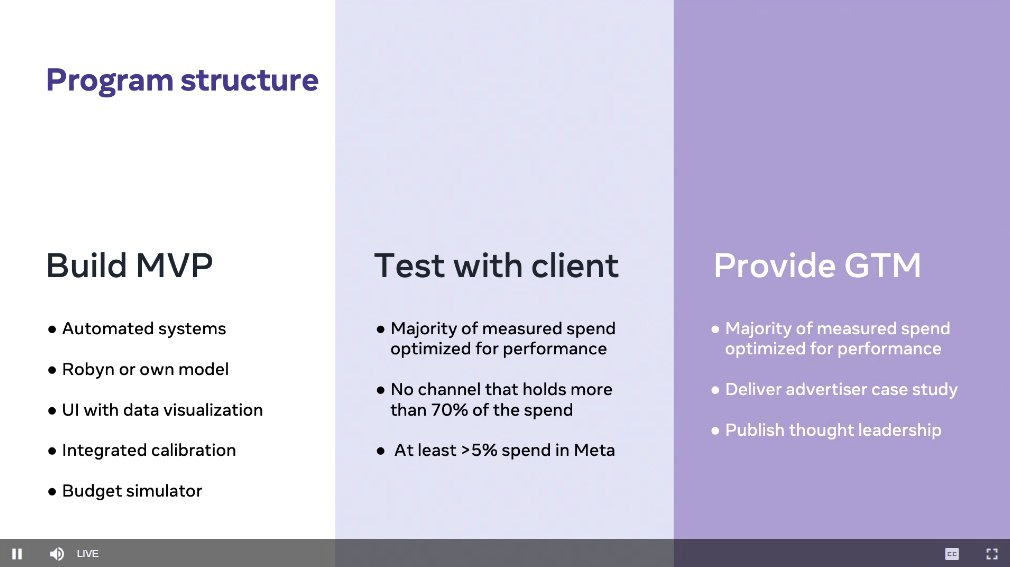
“We look at MMM to measure things that are not measurable” – Elad from AppsFlyer. He makes the point here that MMM is typically used for offline channels, and increasingly unmeasurable online channels like Influencers, Podcasts, etc. Because of the cost, difficulty, and decreased granularity, this has historically been true, but things are improving.
“In the long term we want to understand how can we standardize the data input” – Alessandro from SuperMetrics. It sounds like they’re on a mission to provide a universal MMM feed, which is indeed a noble cause. This is 60%+ of the job most of the time, and the biggest blocker to having an ‘always on’ automated model.
“Once we looked at alternative measurements like assisted conversions [vs last click], we found 80% of all the installs that were attributed actually have seen CTV ads, and were claimed by search channels” – Inga from Adjust. This is a big claim from Adjust. Connected TV is an emerging channel that I’ve heard is getting good results, but if 80% of installs aren’t being attributed, that implies it’s 5x better than most people give it credit.
“Where to put the next dollar is a question that can’t be fully addressed with attribution alone” – Linor from Pecan AI. Yes this is an underappreciated view with regards to MMM. You can’t look at your last click attribution and figure out how much money you’d make if you doubled your budget, but with a good MMM that factors in diminishing returns, you actually can.
“This is a really daunting technology for anyone to ramp up on” – Mark from Branch. Truth.
Melize, a fashion comparison site, got 5% more app installs using MMM to reallocate budget. But actually being able to forecast different allocations was the biggest benefit. – Eunha from Airbridge. Small relative improvement, but sometimes the peace of mind of being able to give a great answer to finance when they ask for budget justification is worth more than better performance.
“We’re automated but not full self driving yet” – Mark from Branch. Good analogy. The process can get pretty good results on its own, but I’m finding in general you still need a smart person following the model’s results and digging into any anomalies.
“Having the MMM model is only the first step. The outputs of MMM can be very complex” – Linor from Pecan. This is true, and the aftercare is the part that most vendors fall down on. Models are worthless if nobody ever takes an action from them.
“Build trust by showing reality matches the predictions” – Inga from Adjust. Simple but effective: if the model can predict the future with reasonable accuracy, the client will trust it. This isn’t the only way to validate you have a good model, and it’s not sufficient for proving your model is correct (it also has to be plausible), but it’s the most important thing to get.
Linor from Pecan said she’s seen some channels where attribution has been overstated due to ad fraud, found via MMM. Elad from AppsFlyer said he’s seen the opposite, where legit channels were credited with zero performance by MMM, as the model got it wrong. I think they’re both right here: I’ve seen it go both ways. Sometimes the model tells you another attribution method is wrong, and sometimes the model is wrong and another attribution method helps us validate that.
Eunha from Airbridge said she got good accuracy in calibrating the MMM with MTA (Multi-Touch Attribution) results, for a client that had never run a lift test so couldn’t do the normal calibration. To me this kind of goes against the point of MMM (which is to provide an alternative to MTA). However if you calibrate the model on MTA and it still deviates, you can be more confident that the deviation is true.
Exploring the links between creative execution and marketing effectiveness
I loved this talk because it was on one of my favorite topics, measuring creative in marketing mix modeling. This ambitious talk was given by Matt Andrews of Ekimetrics, another Meta MMM partner which has built a strong profile from getting in early with Meta on Robyn.
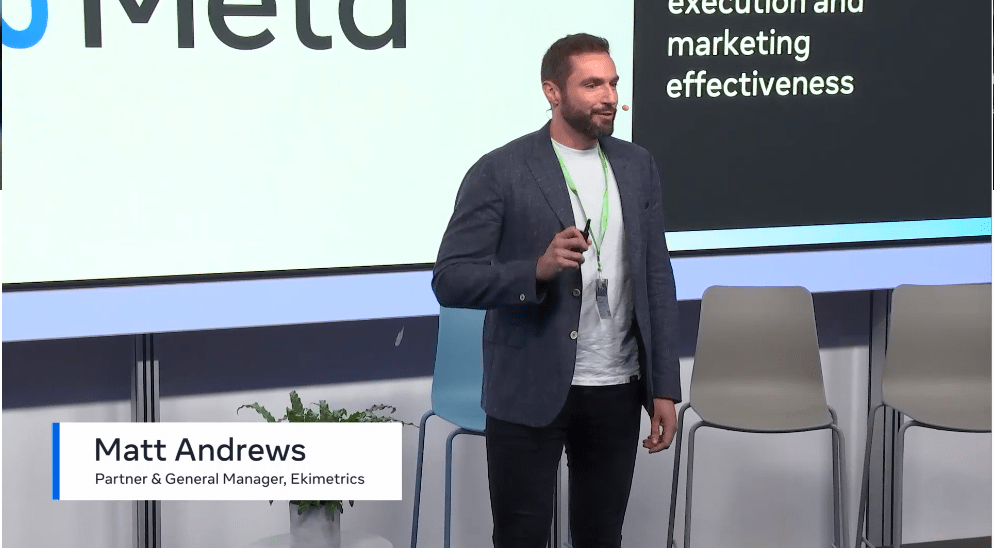
They took the interesting approach of tagging 22 thousand creatives over 3.5 years and $8.9m worth of data, then used those labels as parameters in the model. I’ll be honest, the biggest questions this leaves me with as a practitioner are:
- How they stuff thousands of creative tags as variables into an MMM
- How they got enough instances of the same tag across creatives to spot patterns.
Unfortunately not much detail was given on those issues. I know for a fact that this is hard to do, because I tried to do it myself a couple of times! First was as a product I built for my old agency Ladder, and second more recently when putting together a Vexpower course on creative insights. The most I could do was show relative performance differences by tag: there were way too many variables to include in an MMM!
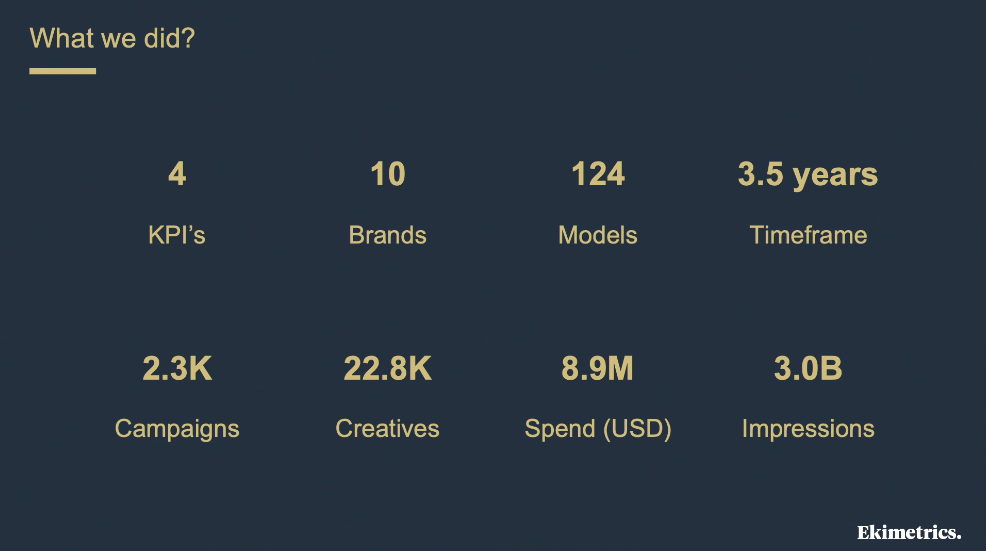
There’s a lovely slide on computer vision, which looks very cool, and is actually more accessible than you think! Even for video, it’s relatively easy to use an external API like Google’s video insights tool to see what labels are in a video. This is something I did as a followup to the creative insights course at Vexpower, to show creative tagging was possible for videos too.
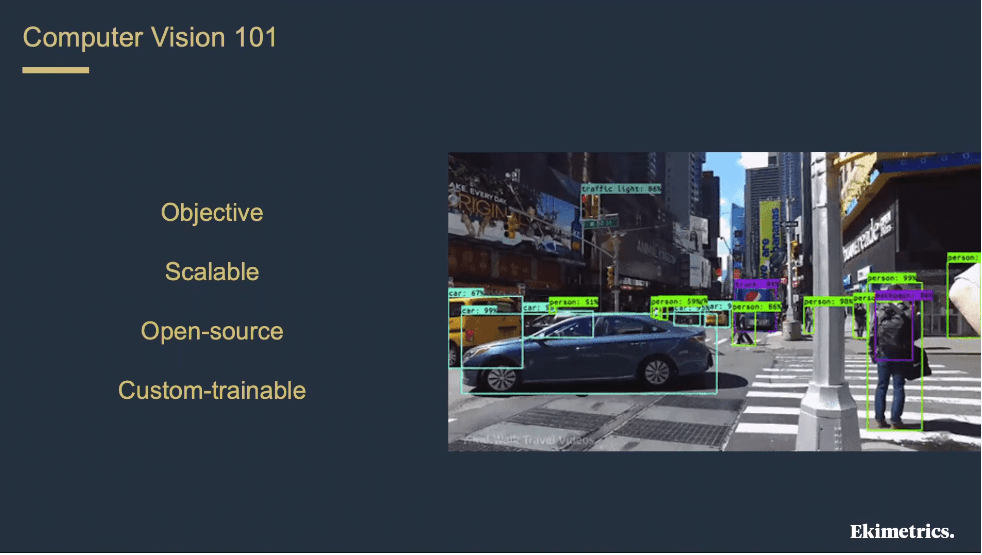
They mentioned that a lot of the work was actually tagging unique features with regards to the brand. This I haven’t done before and would require a custom trained model. It’s definitely worth testing, but the cost would be prohibitively high of training a new model for each new client, unless that client was a very big spender.
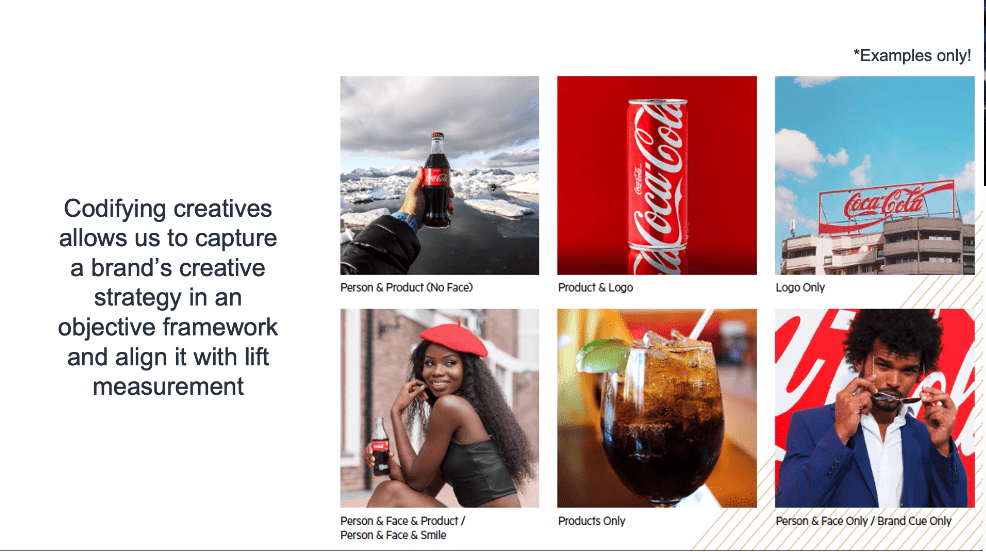
They found some interesting correlations, for example people work in Hospitality and Automotive, Insurance sees an uplift from linking the service to the theme of “protection”, and in Cosmetics you need both text and product images to get the best ROI. Limited insights here for the amount of work done though! I guess creative in MMM still isn’t a solved problem. There is more detail in the paper they shared though, so it’s worth checking out.
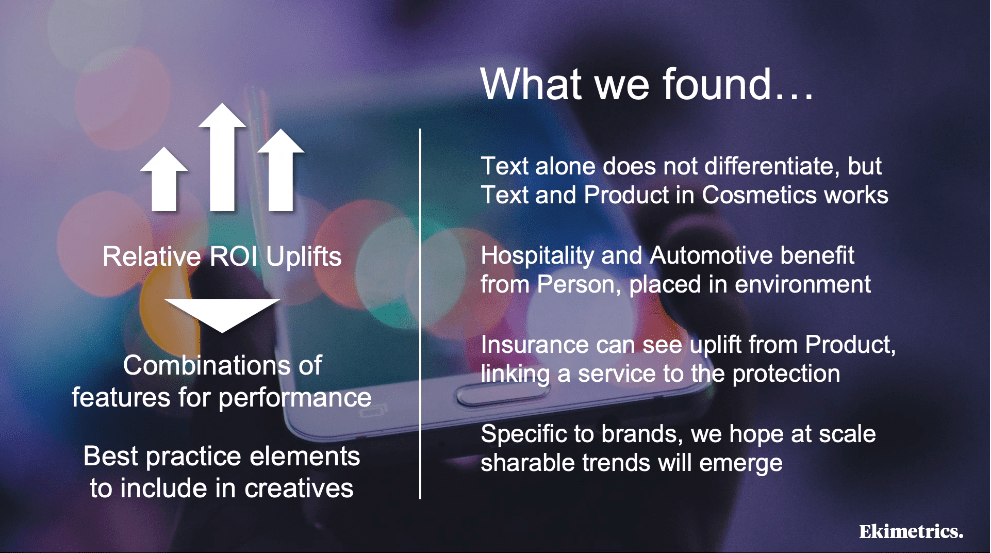
Measuring long term effects with MMM
This next session was one of the most enlightening and actionable segments of the summit. GfK and Meta teamed up to answer the question of how to include the long term effects of brand in MMM. I’ve asked this question a lot, but almost always I’m met with private discussions in my DMs where people candidly admit they haven’t seen it done well. I’ve even consulted with billion dollar public companies who spend hundreds of millions on brand advertising and haven’t figured it out. Well apparently these guys did, and it’s a rather elegant, accessible solution.
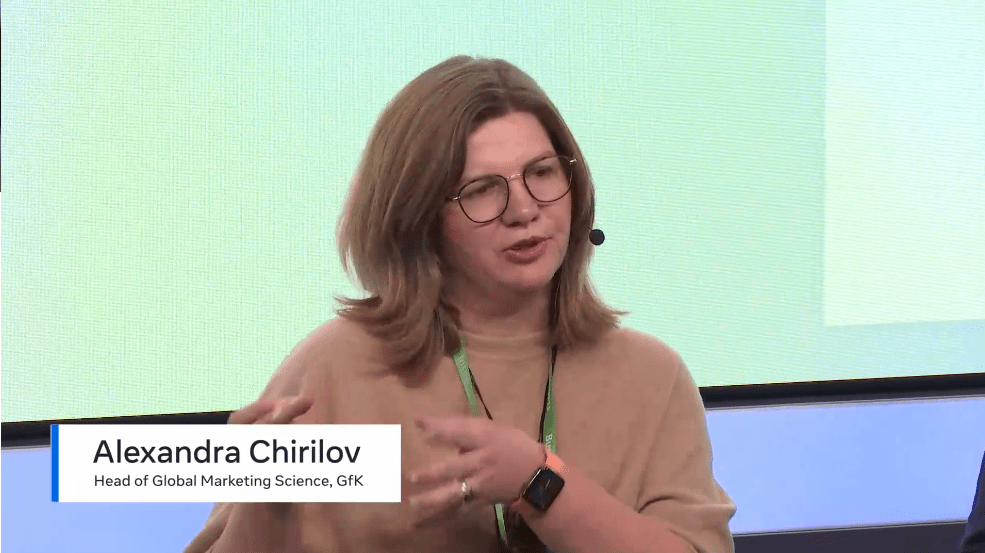
“Short term is not a predictor of the long term” – Alexandra from GfK. She’s seen campaigns that had no impact in the short term but great impact over the long term, and vice versa. This is a big deal! Most assume there must be a short term effect for there to be long term value.
The method that works for Meta and GfK to measure long term brand effects is to use a third party survey that continuously measures brand equity / strength. Can also use Google search term volume as a decent proxy apparently, which is also echoed in the share of search work done by James Hankins.
Their method is simple but effective. They first measure how marketing drives brand, then second how brand drives sales. So they’re describing an embedded or multilevel model, where one model’s output serves as the input for the base sales model. Brand is measured as brand awareness measured by continuous brand tracking surveys, or one of the other brand salience metrics.
Alexandra says that they found dynamic linear models (DLMs) to work best, where there are time varying coefficients. This isn’t a term I’ve heard before, but it’s something I know as Time Varying Coefficients, which Uber Orbit (and Recast) does.
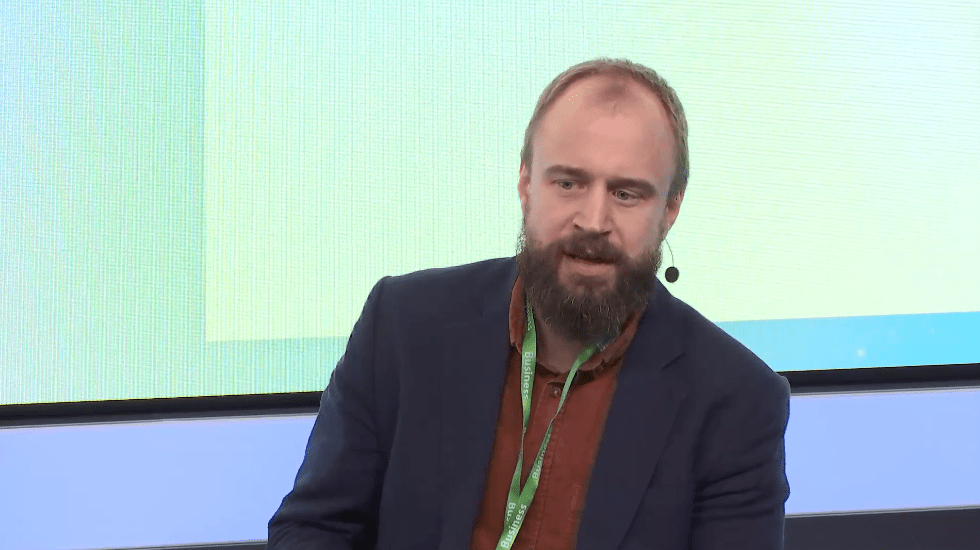
Kalle warns that when using brand tracking data in MMM it can be very sensitive to sample bias and noise. They use noise reduction techniques to clean up the data, and aggregate multiple brand KPIs to reduce variance, though don’t give examples of what they use. The other issue is double counting, because media affects brand but also affects sales, and sometimes those two can get mixed up in the model. Something to be aware of but not a deal killer.
Using DLMs is an elegant statistical approach, because it explicitly includes brand effects in the model. It makes it far more intuitive than simply regressing long term brand survey data alone. DLM / Time-varying coefficients are a must when we have volatility like COVID, recessions, wars, etc. The assumption that one single coefficient explains channel performance across that 3 year time period doesn’t hold anymore.
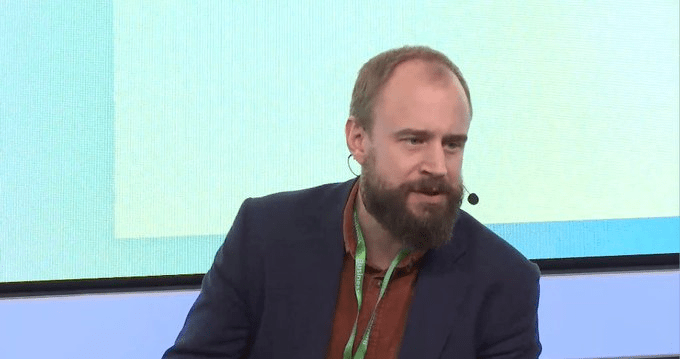
“We’ve seen campaigns that were intended to drive short term performance, also drove long term performance. So don’t go in with assumptions about the short or long term effect of each campaign” – Kalle from Nepa
“How long is long term?” – Alexandra from GfK. She found that some brand campaigns only had an effect of 6 months, which is shorter than expected. Though she says that many of them only ran for around 20 days, which is likely too short to have a lasting impact. Common problem in the branding world: campaign thinking rather than system thinking. However it’s also important to consider that ads that this might be correlation not causation (maybe poor ads get turned off quicker, or less important campaigns run for less time). Also important to account for ad fatigue, as running an ad for too long might hurt performance too.
“A lot of the long term effect is through price elasticity” – Alexandra. Essentially the effect of brand is partially in making customers willing to pay more for the product, and that is part of the long term impact. This is something traditional marketers, with the 4Ps drilled into them, tend to get right more than performance marketers. More details can be found in their paper “The short- and long-term impact of advertising”.
Call to action: how travel industry can leverage Robyn to increase web conversions
This was a pretty unique presentation by Deloitte once I realized what they were talking about. At first I thought it was a project to do MMM for 4 hotel clients. But then I realized they didn’t ask for permission to do these MMMs! They went renegade and used publicly available data and 3rd party paid for data sources to piece together the marketing mix for these hotels. The main data source was SimilarWeb, which I’ve used extensively in the past and it can be really good and useful. However it is limited to desktop data, so I would be careful if you were using this approach to make sure you’re doing it for an industry where desktop is a good proxy for all visits (not a good assumption for most industries now mobile dominates most purchase paths). This is a cool approach that makes me think about all the interesting work that could be done to build rogue MMMs for different industries and reverse engineer their marketing strategies!
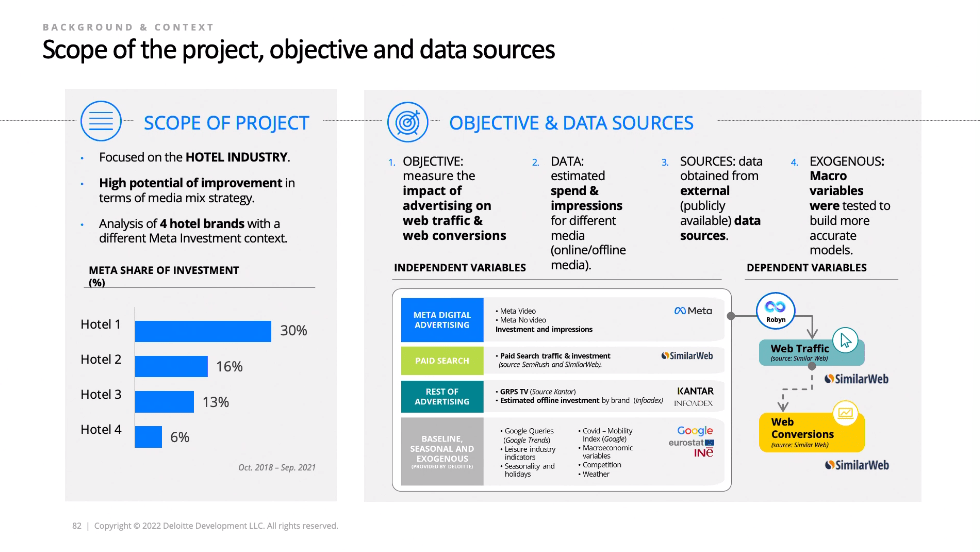
This talk covered again the “triangulation” theme, with MMM being one of the three pillars of measurement that helps you zero in on the truth. This diagram was quite nice though, as it shows an actual triangle, not just a metaphorical one. It showcases the tradeoff between strategic and tactical, as well as model update frequency. In my experience MMMs can be updated daily with the right system, and experiments can be always on with a holdout group, so the analogy doesn’t quite hold, but I appreciate the attempt to map the tradeoffs.
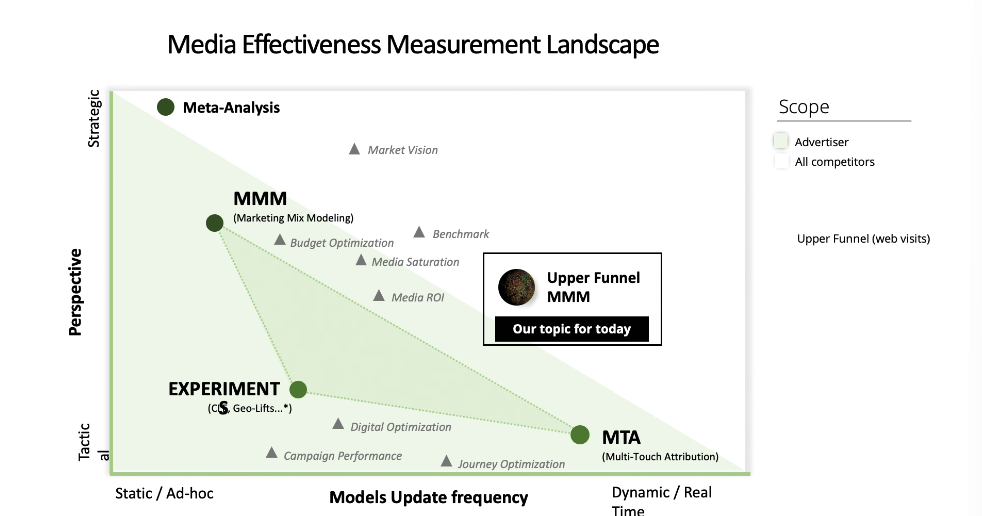
Most advertisers are not using MMM in the travel industry in Spain. Not surprising, as I don’t know of any industry that’s fully utilizing MMM as standard best practice. They did this case study as a kind of showcase of what MMM can do, and specifically Robyn as the great hope towards standardization of approaches. If you standardize the way you do MMM you remove (most) human bias, and can get cross industry insights. One thing that stood out to me was the impact of COVID on the travel industry: if you’re not dealing with it (though maybe don’t control for it) in your MMM you’re definitely doing something wrong. Same goes for seasonality, which is another topic that’s hard to get right.
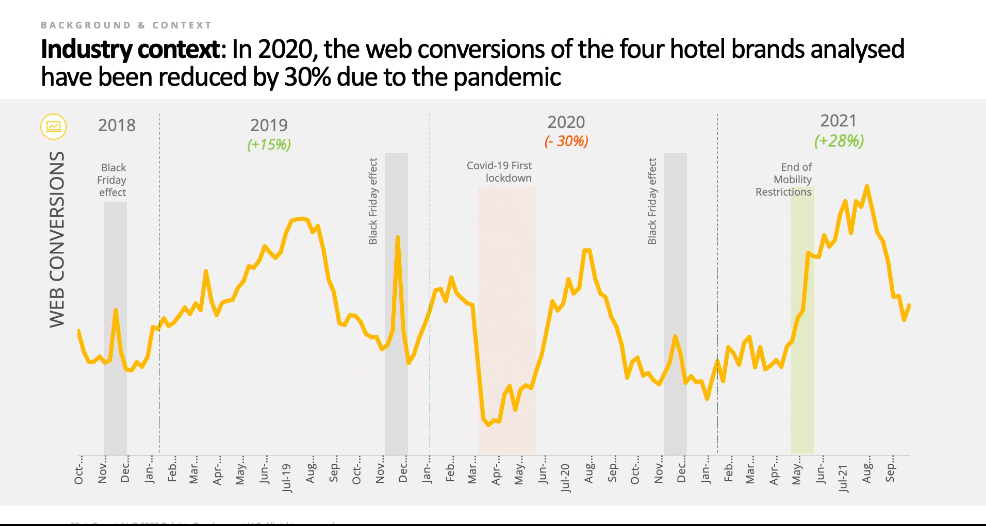
Looks like most of the ad spend is paid search for travel, with Meta being a decent second tier channel. I used to work in the travel industry and if anything it was even more weighted towards paid search back then. Interesting to see Out Of Home taking up 12% of ad spend as well as newspapers and magazines taking a good chunk, and then they all get wiped out by Paid Search and Meta over the next few years. I’m not sure where they got the meta spend data, that would be good to know!
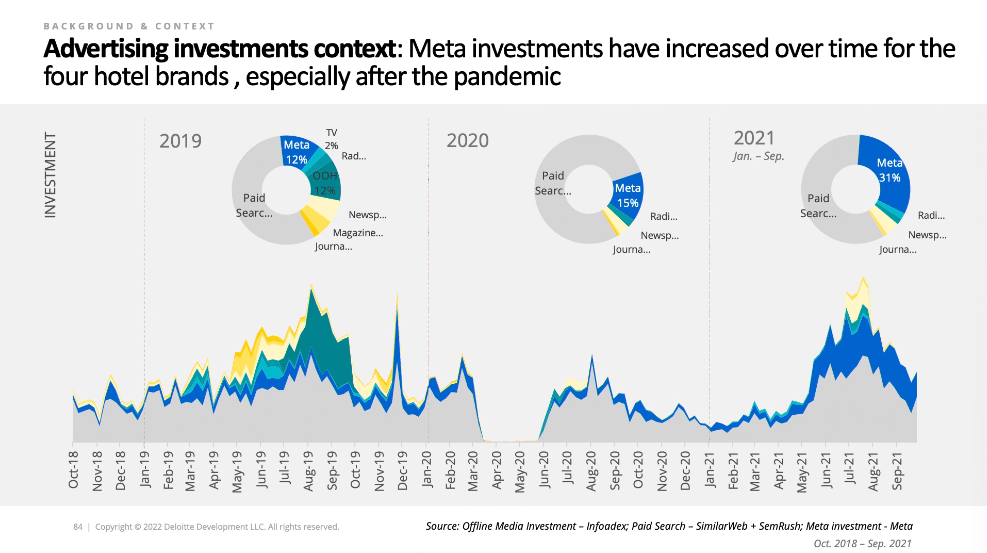
This has always been one of the sexiest views of the Robyn tool. Seeing visually all 10,000 models it ran for you is just really cool. The pareto curve of which models had the best accuracy versus plausibility is a masterclass in data visualization. Feels like proof of work. Makes it look a lot more trustworthy. Then the one pager model report backs it up with just the most important information. The one area where Meta falls down is on model selection: they still give you too many models to choose from, and no real guidance on how to choose.
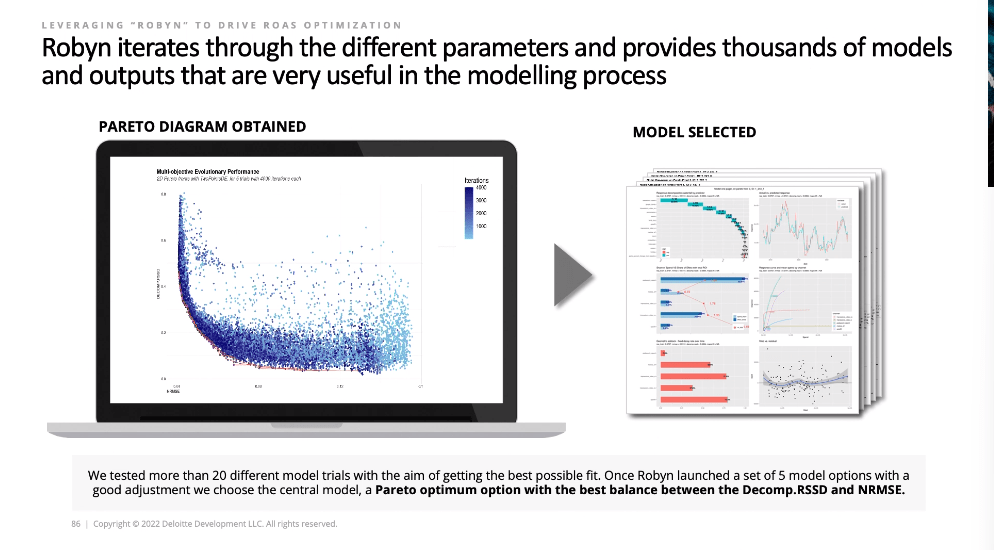
Always a crowd pleaser is the decomposition chart. It increases trust further if you can show your boss or client what the model thinks drove the most contribution to sales at different times. They immediately start tracing lines with their eyes and seeing if it lines up with their experience. One thing to point out is that the baseline sales uses web queries, which is a technique I’ve also found useful for controlling for seasonality. I’d love to know what they mean by overnight stays though: how would that be counted as baseline sales?
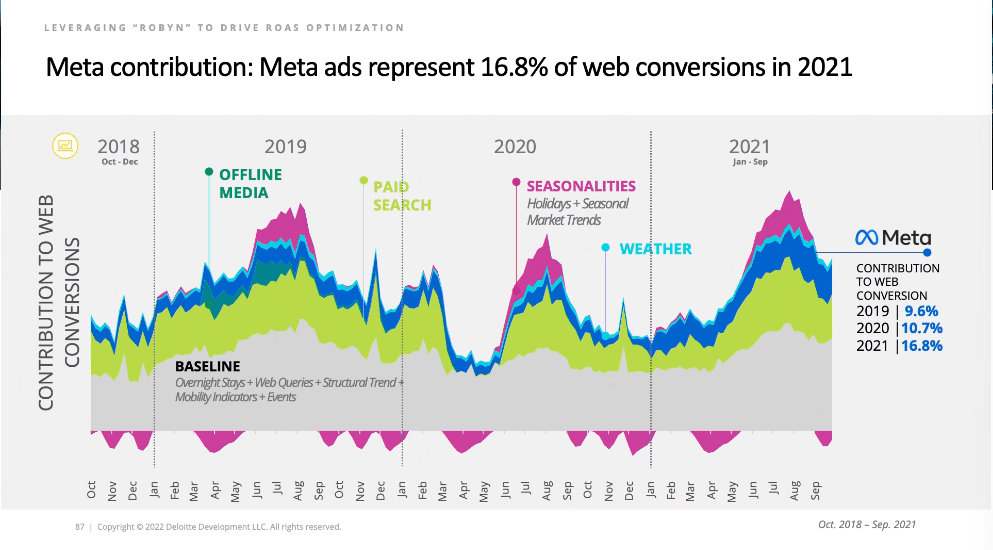
There’s no surprise here, TV, Video, OOH has higher adstocks than other channels, PPC has the shortest adstock. I’ve never once seen a result that doesn’t look like this, but it’s always nice to have it reconfirmed. If you were one of these hotel brands you could use this info to justify more investment for top of funnel channels. It’s also important to take this into account when designing a lift test: If video ads have a long adstock you have to make sure you don’t turn off the lift test too quickly or the result will be invalid.
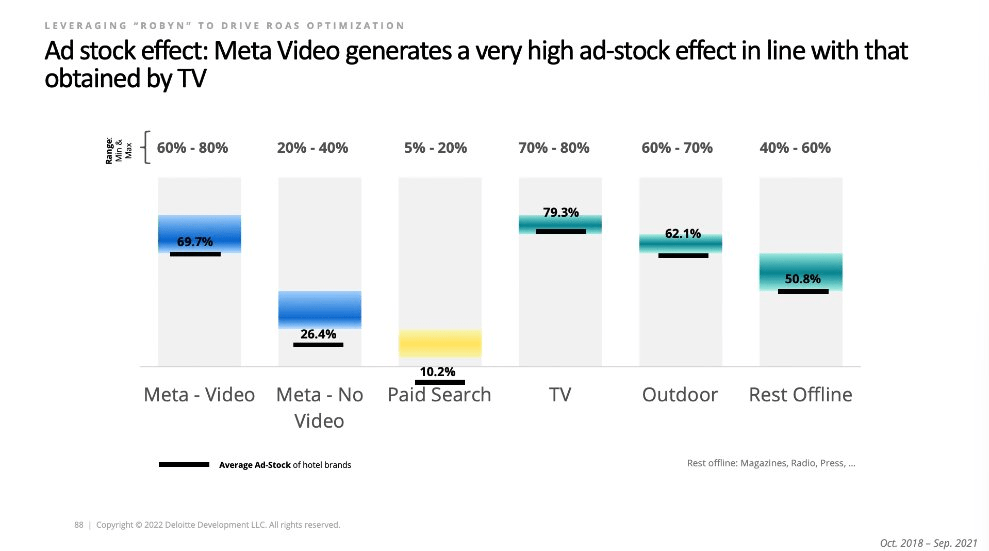
The key part of making MMM actionable is giving marketers a better budget allocation based on the results of the model. It has been mentioned by a number of speakers today. The best practice here is to give the team a simulator tool that lets them play around with different spend allocations and see the dynamics, but you can also go the Robyn way of finding the right budget split for you.
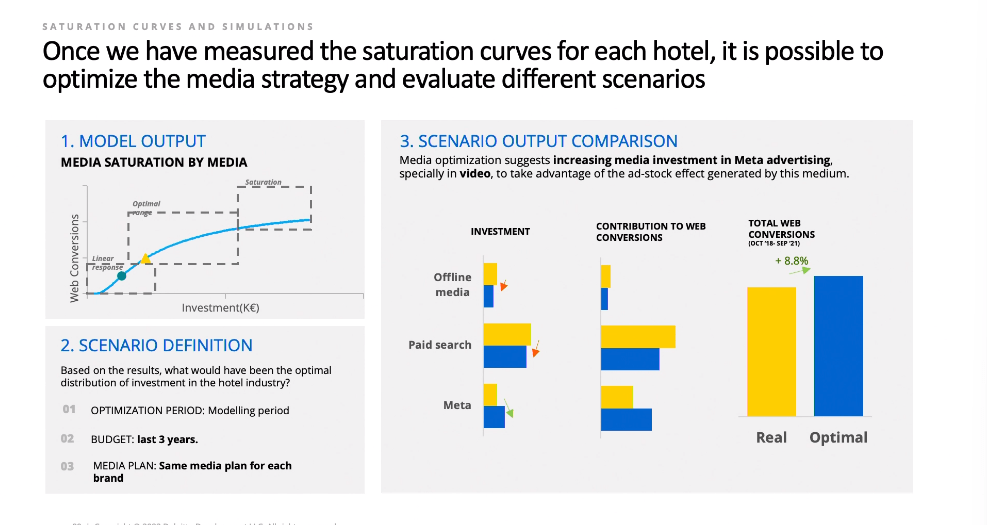
The main benefits of Robyn honestly map really well to any modern MMM vendor. Removing human bias, testing multiple configurations, and making decisions quicker is the promise of all the reinvention that has been going on in the space. For more information check out their case study, How the Travel industry can harness Robyn.
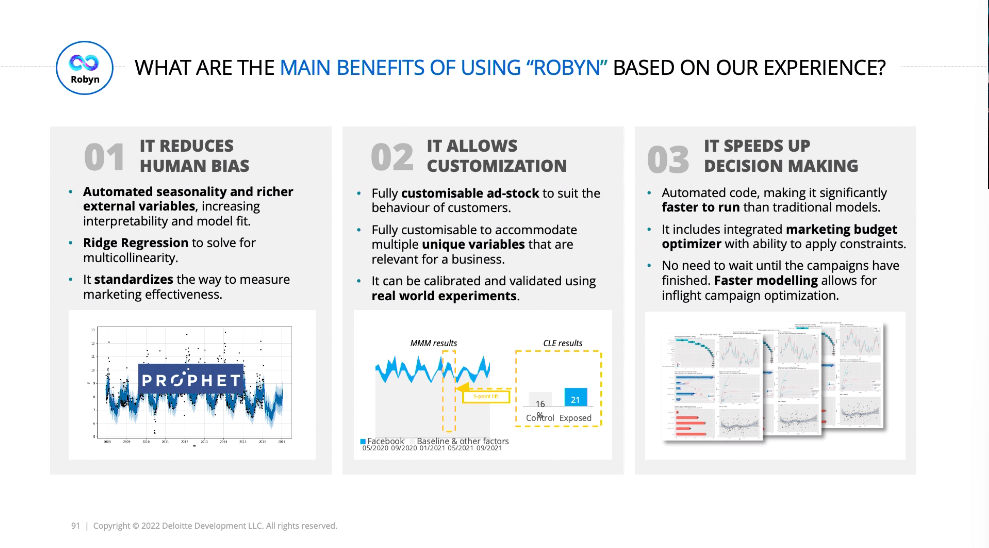
Conclusion
There were many takeaways from the summit, but I would say on balance more questions than answers. We’ve come a long way as an industry even over the past year, in part thanks to the stellar work Meta has done to push the industry forward and bring awareness. We have made strides in automation, both in automatically collecting and cleaning data, but also in automatically building robust and reliable models that can handle being updated regularly. There is also increased mention of time-varying coefficients, which is I think the next big leap forward. It also looks like there’s hope of standardizing the way we incorporate long term brand effects.
MMM is becoming more accessible with open source tools like Robyn, and frankly all the information out there on best practice, of which this webinar was a fantastic example. Now most people agree on the ‘triangulation’ approach, of considering multiple attribution methods and finding the truth about performance in the messy middle. Also calibration techniques have started to become industry standard, which is an overwhelmingly positive approach which will weed out a lot of bad actors and biased modelers. MMMs are also becoming more actionable, with better data visualizations and budget allocation tools.
However the same old problems remain. There’s still no good solution for incorporating creative in MMM, which is consistently shown to be the most important factor for performance. Granularity is being preached as important now, but I don’t see many valid solutions for including a lot of extra variables in models. Robyn does it by shrinking coefficients to zero with Ridge Regression, but then you have the unfortunate result of telling a client one of their channels or campaigns drove zero sales (unlikely to be true!). There was also no talk of how MMMs can model new channels, a perennial problem that has yet to be solved. Maybe next year!