Marketing Mix Modeling (MMM) has been around since the 1960s, where it was used to correlate spikes and dips in sales to marketing activity. There has been a recent resurgence of interest in marketing mix modeling, as consumers increasingly opt out of digital tracking and digital-first brands mature to offline channels. We’re now living through a renaissance period in marketing mix modeling software as modern data pipelines, new Bayesian methods, and open-source options like Robyn from Facebook (Meta) and LightweightMMM from Google, help decrease the cost and complexity of MMM.
Avoiding wasted advertising spend is an age-old problem, but one that has become more urgent with Apple’s iOS14 update allowing users to opt out of tracking in droves. Every brand is being forced to reassess how its attribution stack measures up, and realizing they need new tools and talent to properly assess the marketing effectiveness. Another age-old problem – “should I build or buy?” – is made more difficult to answer due to fast-changing, uncertain conditions. We’ve put this guide together with the build vs buy calculator to help you decide.
Building Marketing Mix Modeling In-House
Marketing Mix Modeling software is hard to build, because it’s not just a prediction problem: your model has to give plausible estimates for the ROI of each channel to be believed. Marketing in particular can be hard to model because there’s often multicollinearity – marketing channels are correlated with each other – meaning it’s easy to get the model wrong. There is also a long checklist of features your model should have, as well as the various charts, tables, and recommendations required for decision-making. Facebook (Meta) estimates it takes 12-22 weeks to build a model manually, which doesn’t include the development and maintenance work to automate the process on an ongoing basis.
We’ve found for smaller companies a ‘good enough’ marketing mix model can be built over the course of a few weeks, although these timelines are typical in our experience for larger organizations. Let’s assume that it takes 6 months on average for a team of 2 Data Scientists working full time to build your initial model and automate it. The average salary for a Data Scientist is around $100,000 according to the US Department of Labor, though it can be much more in New York, Austin or the Bay Area. Following completion of the model, it will take 1 day every 2 weeks, plus a bigger 4 week project each year to maintain and update the model.
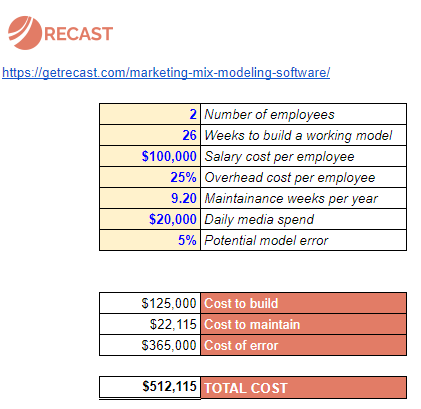
The resulting calculation leaves us with a $125,000 bill for the project, and an additional $22,000 per year in maintenance. Of course if we find the model significantly degrades or a new technique comes out that we want to incorporate into our marketing mix modeling software, that will incur additional development costs. This isn’t including what could be the most significant cost: getting your marketing budget allocation wrong. If you’re spending $20,000 per day on media and your model misallocates just 5% of your budget, that’s $365,000 wasted for a grand total of over half a million in costs! The link to the calculator is below – make a copy of the template if you’d like to adjust the assumptions.
Buying Marketing Mix Modeling from External Vendors
There are multiple MMM vendors on the market now, including Recast. One major philosophical divide is between frequentist and Bayesian methods. Traditional marketing mix modeling used ordinary least squares (OLS) for linear regression, which is a frequentist approach and commonly used by consultants. Facebook Robyn uses Ridge Regression, which is a more modern take on the same category. Google and Recast use Bayesian methods, which is a more recent development, and offers significant flexibility in incorporating prior domain knowledge into your model, leading to more plausible results.
The biggest difference in cost and maintenance will be whether you decide to use automated software or have MMM done as a custom consulting project. Most traditional MMM consultants have their own style of doing things, and can build something custom for your business if needed. However the manual approach requires a lot of trust, because MMM is susceptible to human bias – it’s easy to be fooled, or worse, to fool yourself. Automated tools can use modern data pipelines to update the model regularly, so decisions can be made more often without redoing the analysis. It’s also possible to automatically make budget allocation recommendations with automated models, which make them far more actionable.
When it comes to selecting a vendor, remember that the cost of the engagement or software is probably not the most important factor: if the model misleads your company, you could end up losing 10x (more) than what you paid to the modelers. So you’re going to want to evaluate a vendor along the following dimensions:
- How much of the MMM checklist do they have good answers for?
- Have they worked with similar companies or brands you admire?
- What’s the cost of the initial model build?
- What are the ongoing costs of maintenance / running the model regularly?
Once you’ve made decisions on what statistical approach and whether you want to use scalable software or build something custom, the final hurdle is in the detail of what the model will do. The field is nascent so every vendor does things differently. There are several standard things to watch out for like diminishing returns (saturation of channels at high spends), adstocks (delayed impact on performance) and a number of other model features. There are also more opinionated approaches that can significantly change the model’s recommendations, like how you account for changes in channel performance, handle creative or deal with seasonality. If you’d like to hear about how Recast handles these questions, we’d love to hear from you.