For years after I left my agency I described myself as a “recovering agency owner”, and it would always get a knowing laugh from anyone who had experience running a services business. From the outside it might look like agencies print money, but I assure you that the economics aren’t as good as you’d think. We were successful by any measure – over 50 employees, working across 3 global offices, for an enviable roster of clients – but the nature of agency life is that you’re always going from one fire to another. One of those fires started with a naive switch to Google’s Data-Driven Attribution model, and it ended up torching $40,000 of ad spend that we ultimately had to refund the client.
I can’t name them due to NDA, and we didn’t part on the best of terms, but you just need to know they had a portfolio of local event venues across the United States, and our job was to drive booking enquiries from Google Search Ads. Every ad was geo-targeted to a radius, in which people were within traveling distance to that event venue. It was a high-spending account – $100k to $200k per month – and we ran a sophisticated operation, with 100s of campaigns across 10s of thousands of keywords, automatically generated and updated based on templates. They kept us on our toes, and we had some of our best people working on the account, which I checked in on at least weekly as the co-founder in charge of operations.
Data-Driven Attribution
At the time we were optimizing campaigns based on last-click attribution, which is the default, but everyone knows that it’s flawed. Last click tends to overweight extremely expensive commercial terms, and undervalue more informational searches where there may be an opportunity to get ahead of the competition. There are different ways to solve this problem – first touch, position-based, time-decay – which share the credit for conversions more equally across multiple touches in the search journey. However the most advanced was Google’s Data-Driven Attribution model.
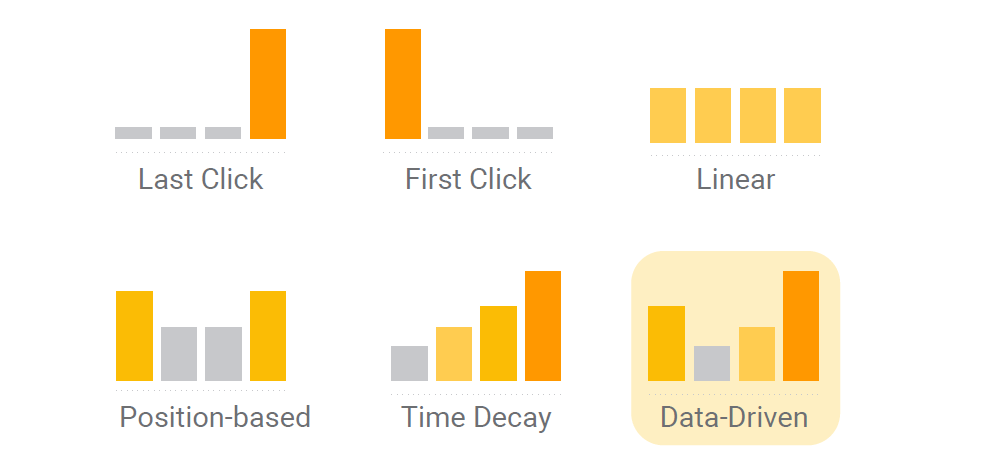
Google provides the following helpful example:
Example
You own a restaurant called Ristorante Abigaille in Florence, Italy. A customer finds your site by clicking on your ads after performing each of these searches: ‘restaurant tuscany’, ‘restaurant florence’, ‘3 star restaurant florence’ and then ‘3 star restaurant abigaille florence’. She makes a reservation after clicking on your ad that appeared with ‘3 star restaurant abigaille florence’.
https://support.google.com/google-ads/answer/6259715?hl=en-GB
In the ‘Last click’ attribution model, the last keyword, ‘3 star hotel paulina florence’, would receive 100% of the credit for the conversion.
In the ‘First click’ attribution model, the first keyword, ‘restaurant tuscany’, would receive 100% of the credit for the conversion.
In the ‘Linear’ attribution model, each keyword would share equal credit (25% each) for the conversion.
In the ‘Time decay’ attribution model, the keyword ‘3 star restaurant abigaille florence’ would receive the most credit because it was searched closest to the conversion. The ‘restaurant tuscany’ keyword would receive the least credit since it was searched first.
In the ‘Position-based’ attribution model, ‘restaurant tuscany’ and ‘3 star restaurant abigaille florence’ would each receive 40% credit, while ‘restaurant florence’ and ‘3 star restaurant florence’ would each receive 10% credit.
In the ‘Data-driven’ attribution model, each keyword would receive part of the credit, depending on how much it contributed to driving the conversion.
Rather than assign credit based on arbitrary rules made by (fallible) humans, it uses sophisticated machine learning algorithms to assign credit closer to what really drove conversions. This powerful model would normally cost thousands of dollars, but was made free by Google thanks to their acquisition of Adometry: I had a few smart friends working there so I knew the model was top notch. Our Google Ads lead pitched me on making the switch, something we had done multiple times before with no issues, and I agreed: it was a good idea. The change was made near the end of the month, as one of multiple optimizations on the account that week.
Note: Since the release of Google Analytics 4, and in the aftermath of Apple’s iOS14 release punching a big hole in tracking, Google moved to make Data-Driven attribution the default. To find out why you should be wary, keep reading.
The $40,000 Mistake
Fast forward to the following week, which was the first week of the new month, and we’re doing our regular reporting. It’s always busy in agencies on the first Monday of the first week of the month, because not only are your weekly reports due, but you also have to report on monthly performance. I was in the middle of fielding the many questions and requests that inevitably come my way as the analysts compile their reports, when I see an email come up that gives me pause. The subject line was “stallion springs: screw up?”. Actually I changed the name for anonymity: it was another location where I knew the client had a small event venue.
At first glance I remember the performance on the account in that morning’s report had looked great: Google was reporting a 156% increase in new booking enquiries across all venues, which was consistent with our increased ad spend towards the end of the quarter to hit aggressive targets. In fact, normally when you scale spend you hit diminishing returns – cost per conversion gets worse at higher spend levels as the channel gets saturated – so the team had pulled off quite a feat by keeping customer acquisition cost in check despite a ramp up in spend. I wondered why the client would complain about performance in one of their smaller locations when overall performance was great. I nervously opened the email.
In looking at the email, I could immediately see three things wrong.
- The Stallion Springs campaign, normally a low spender, had increased by over 2,000% in the final 4 days of the month
- The majority of the excess spend had found its way onto the display network (an optional setting that’s default on, but normally not impactful), rather than search
- Stallion Springs bookings in the CRM were flat week on week: in fact Google was claiming hundreds more enquiries than the venue had total from all sources!
Later investigation concluded that it was Data-Driven attribution that went haywire, thinking that it was getting all these conversions from display advertising for this one minor location. We held out hope that potentially it just drove a load of people to the website who converted elsewhere, but unfortunately no, that wasn’t the case: we found other factors that explained the relatively better performance in the rest of the account. We even escalated with our Google rep and got nowhere: even the engineers don’t really know what the machine learning algorithm is doing, it’s a black box that (mostly) works better than the other models.
You might say it was the team’s responsibility to catch this, but ultimately I’m a believer in systems. We had automated reports, but there were no automated spending alerts set up to notify us of anomalies. Google’s attribution is normally top notch, but we had no 3rd party attribution method like Marketing Mix Modeling running to sense check Google’s numbers. The team did check the campaigns and they looked fine… but our standard operating procedures (SOPs) didn’t specify the team should investigate at the location level. I only assumed that they would catch these sorts of things, because that’s what I would do. Bad assumption. Unless your employees are being careless or malicious, it’s the system that’s wrong.
The client was justifiably upset with us. After a long and protracted series of difficult discussions, we ended up having to refund $40,000 of the client’s money, in the hopes of keeping them. They left anyway. This wiped out 2 whole months of fees, but we still had to find a way to cover salaries. It was just blind luck that we could pay the cash without letting anyone go. The real wake up call was when I realized how lucky we were this only happened once: there was nothing to stop this happening all the time! Without better safeguarding systems in place, better processes that explicitly defined daily checks, and alternative attribution methods running to keep Google honest, we were at risk. All of these were in place within a month. Nothing motivates you like an existential threat and $40k down the drain!
Conclusion
Hopefully now you’ve seen that the importance of good attribution can be an expensive lesson to learn. I hope you take this post to heart and never have to learn it the hard way like we did. This isn’t a post bashing Google’s modeling ability – I’ve used Data-Driven Attribution to good effect since the incident – but even a company with the resources of Google can’t build an attribution model that’s 100% reliable. The harsh truth you need to hear is that no single attribution method can be trusted. They all have their strengths and weaknesses, and they’re all flawed in their own way. The key is knowing what attribution methods are available, and combining them to triangulate what true performance is.