If there’s one thing everyone knows about statistics, it’s that ‘correlation is not causation’. Ice cream sales and shark attacks both rise in the summer, but one doesn’t cause another. The hot weather gets people both buying ice cream and swimming in the sea in greater numbers.
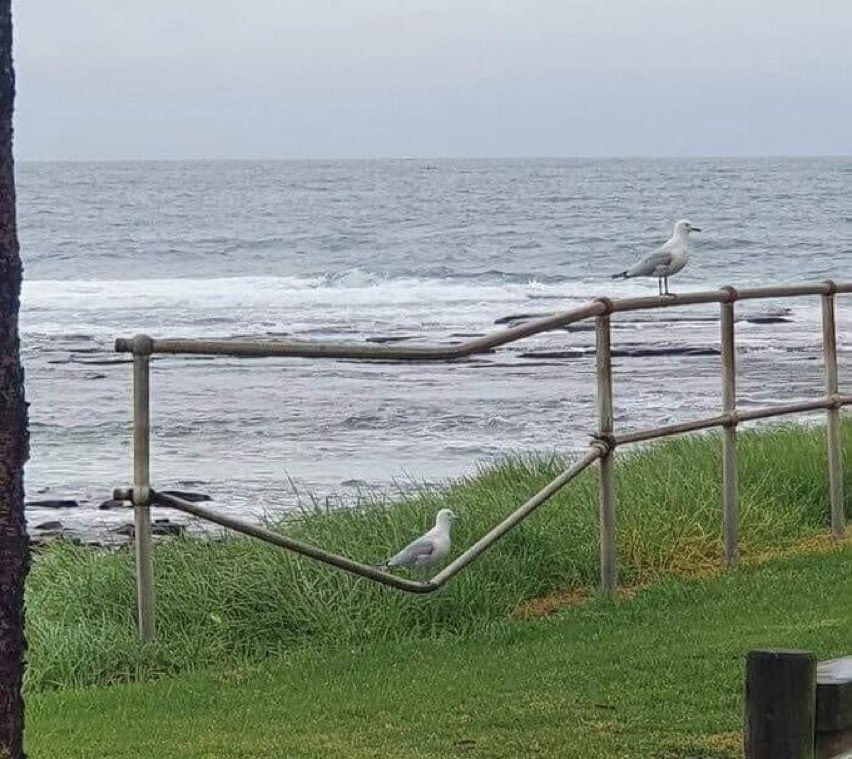
If we’re advertising our ice cream brand in the local paper, how many of our sales from our marketing campaigns vs the good weather? It’s a tricky question with no one right answer. Much of the work marketing scientists do each day is trying to work out the impact of marketing. It’s an important question, because you can’t justify marketing investment without a good answer.
In 2021 the Nobel Prize in Economics was awarded to Joshua Angrist, David Card, and Guido Imben for their work on Causal Inference. They contributed new tools for establishing causality that marketers, academics and even policy makers are using to make decisions. We’ll discuss these techniques in a moment, but first, let’s look at where Causal Inference sits on the hierarchy of evidence in marketing.
Hierarchy of Evidence in Marketing
What constitutes ‘evidence’ that marketing caused higher sales? Some methods are better than others, but aren’t always available. The least reliable (but a step above gut feel) is trusting expert opinions: follow best practice and your company will likely grow, even if you can’t prove why. At the very top of the pyramid are Randomized Controlled Trials (RCTs) which are the most certain you can be that an effect is real (and not just random noise / coincidence).
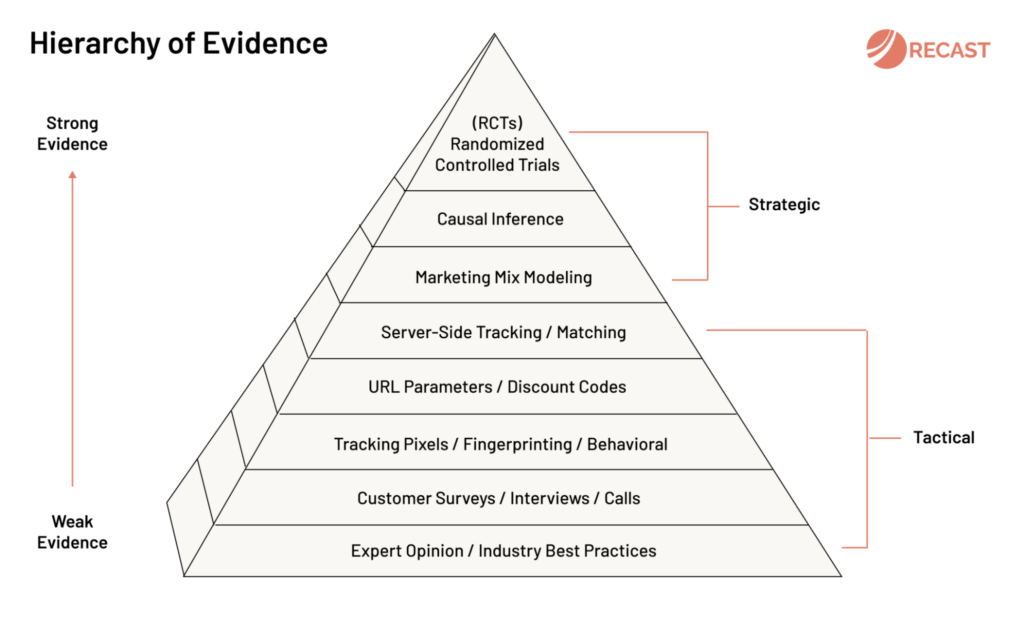
Randomized Controlled Trial (RCT)
The gold standard for proving causality is the Randomized Control Trial (RCT): you may know them as A/B tests. If you can randomly assign potential customers into test and control groups – 50% are shown an ad, and 50% are not – then you can be quite sure the difference between the two can be attributed to the test. Both groups experienced exactly the same conditions other than the test, so all other factors that affect sales (like the weather) are controlled for.
Causal Inference
In practice true RCTs are difficult to set up and run, because in marketing we don’t have control over the ecosystem. For example we can’t control who reads the newspaper, or measure if they saw our ad. So new techniques were developed like Instrumental Variables, Regression Discontinuity, and Natural Experiments, which let scientists emulate the conditions of an experiment, in situations where running a true randomized controlled trial isn’t possible or practical.
- Instrumental Variables: a variable exists that is associated with changes in marketing performance but do not led to change in sales directly (e.g. SuperBowl host cities are chosen as good as randomly assigned before ads are purchased, which will affect ad spend by state but not sales in that state)
- Regression Discontinuity: there’s an abrupt change in policy across a continuous variable, like time or age (e.g. if over 65s are charged a difference price you can isolate the effect of price by comparing 64-and-a-half year-olds with those that are 65)
- Natural Experiments: an historical accident simulates random assignment (e.g. you de-index your website from Google and see a drop in unattributed direct traffic too)
Marketing Mix Modeling (MMM)
Using Econometric modeling it’s possible to associate spikes and dips in sales with changes in marketing activity. This approach is holistic and doesn’t require user-level data, so it works across all marketing channels (even offline) while also incorporating external factors (like the weather). Historically this was time-intensive and required advanced statistical knowledge, but Recast and others are democratizing and modernizing this practice with Bayesian methods.
Other Attribution Methods
The majority of marketing today is digital, where controlling the ecosystem is a little easier (though still not perfect). This means that digital only marketers tend to fall back on methods like Tracking Pixels, URL parameters or Server-Side tracking. These techniques are good for tactical insights and optimization, for example ‘what ad creative is performing best’?
Brands who have harder to track channels like TV, Billboards, PR, Newspapers, Influencers, and Podcasts in their marketing mix can’t rely on digital tracking alone. They tend to pair online data with Customer Surveys and Discount Codes, as well as graduating to the more strategic and sophisticated methods like RCTs, Causal Impact and MMM.
- Server-Side Tracking / Matching: matching behavior of logged in users across devices
- URL Parameters / Discount Codes: a unique URL / unique code for each campaign
- Tracking Pixels / Fingerprinting / Behavioral: record user-level data using cookies / device identifiers
- Customer Surveys / Interviews / Calls: ask your customers how they heard about you
- Expert Opinion / Industry Best Practices: trust that following best practices is working
If you want more information on these techniques and when to use them, check out The Attribution Stack which covers their strengths and weaknesses in more detail.
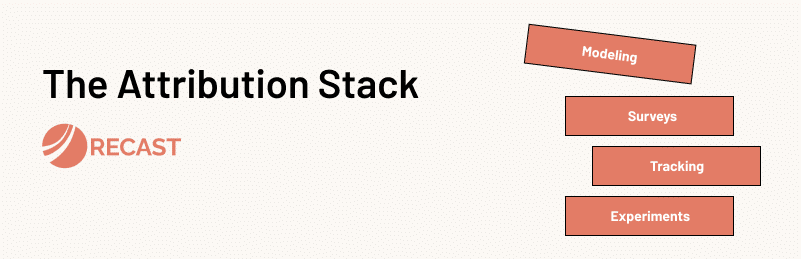
When is Causal Inference appropriate?
It’s easy to get statistics wrong, and Causal Inference is similar to Marketing Mix Modeling, in that you need someone with experience to guide you through the process. Typically these methods are a good fit for brands that already spend several million dollars a year on marketing, have a marketing science team or data scientist, and are using multiple attribution methods spanning from digital tracking to RCTs and MMM.
Causal Inference techniques are all relatively unique in terms of when they can be used. For example you need to devise a good instrumental variable or regression discontinuity, or be lucky enough to stumble upon a natural experiment. Even when you have the results of a Causal Inference quasi-experiment, you will want to calibrate your findings against other attribution methods to be sure. If it departs from what you already know about the business, that’s a sign something went wrong or an opportunity to learn something new.