The Search Advertising Market has been valued at 178 Billion in 2021 and is expected to grow at an 8.5% Compounded Annual Growth Rate from 2021 to 2018. Search is an important part of the Marketing Mix for most companies.
One of the drivers of Search Advertising’s rise is its (alleged) ease of measurement: thanks to digital tracking systems that encompass cookies, pixels, device IDs and other technologies, marketers know how many users saw an advertisement, clicked on it and then proceeded to complete a valuable action for their business such as purchasing a product or completing a lead generation form.
This tracking infrastructure, assisted by reporting tools built by the advertising platforms (Google, Bing) facilitates the attribution of value to these channels. However they often take more credit than they deserve, to the detriment of the less measurable upper funnel (Social Media, Display), and offline channels (TV, Radio, Print).
When a user buys a product after clicking an ad, that’s not enough information to conclude the user bought the product because of the ad. We don’t know what other marketing touchpoints occurred before they searched. If they searched for the company’s brand name, they must have heard about the brand from somewhere, and yet digital tracking would assign full credit to the search campaign they clicked on.
The incrementality question?
To really get an unbiased measurement of the effectiveness of this Marketing Channel, we should start with an Incrementality question: “What would have happened had we not activated Paid Search?”. If we know how much lower our conversions would have been in that scenario, that’s the true amount paid search should be credited for.
Some economists and research scientists have tried to come up with an answer, focusing on Branded Paid Search as the biggest offender: searches for keywords that contain your company or brand name. Their assumption is that many users who click a paid link for a site when they search for a specific brand would have visited the website anyway via the organic link on the search results page.
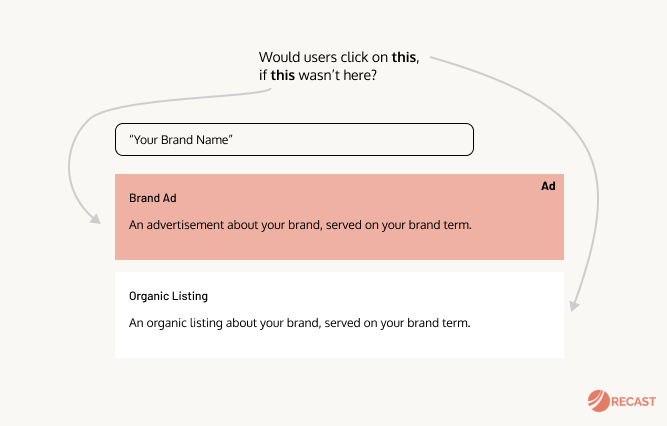
The first big study on the incrementality of Branded Paid Search was run by Blake et al (2015) at Ebay: they did a series of fields experiments coming to the conclusion that, because clicks and purchase intent are highly correlated, the incremental impact of this channel is marginal or, in the worst cases, even absent.
Other researchers, like Coviello et al. (2017), found conflicting evidence by replicating the same experiments in a smaller brand: Edmunds.com. They discovered that only half of the traffic that went to the website through Branded search ads would have still gone there with only organic links.
Given that users were searching for the brand term, it’s surprising that any traffic at all was incremental. However the most likely explanation for this difference lies in the fact that the other half of the users may have clicked sponsored links from competitors who were bidding on the keyword “Edmunds”. There are also stylistic differences between ads and organic listings which may increase overall click through rates.
The useful point that we can take out of these two studies is not whether Branded Paid Search is incremental or not, good or bad, but the methodology that everybody can use to measure its incrementality in our own business.
As we cannot use Randomized Controlled Trials (RCT) – the gold standard of proving causality – in tests comparing Google Ads or Bing Ads to organic listings, we need to fall back on Causal Inference methodologies such as Geo Experiments, and Regression Discontinuity.
Looking for the answer with Causal Inference
Luckily, you don’t need to have PhDs in Economics in your team to run these studies: recent advancements in quasi-experimentation tools are democratizing the opportunity to leverage these methods to everyone who has familiarity with scripting languages such as Python and R, very common in the Data Science community.
The intuition behind a Geo Experiment is relatively straightforward: you take the market and divide it into the geographical unit (i.e. States, DMAs, Zip Codes). Then, you randomise half of the units into a treatment (Paid Search switched on) and a control group (Paid Search switched off).
After observing the two groups for a given period of time (usually a few weeks) we can measure the incrementality of the channel through statistical methodologies called “Difference in Differences” and Synthetic Controls that can be deployed with simple packages like GeoLift (built by Meta Marketing Science team).
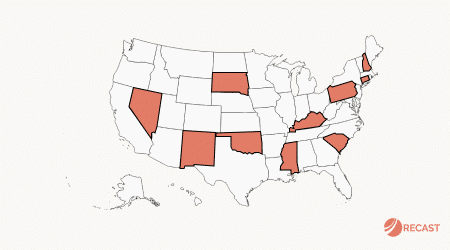
Sometimes, running a Geo-Experiment is not possible or convenient: you might operate in a small market or the volume of your conversions is too small and there is a lot of variability across geographical units.
As an alternative, you can use a method from the Regression Discontinuity family to estimate the causal effect of switching on or off the Paid Search Channel on the business outcome (i.e. Conversions).
This methodology uses historical data up until the launch date to forecast how the trend of conversions would have evolved hadn’t we switched on the campaign (the dotted line in the graph below).
Once estimated the counterfactual, it measures the difference between the estimated trend(dotted line) and the actual data (red line) to calculate the incremental impact: the conversions that would not have happened without the campaign.
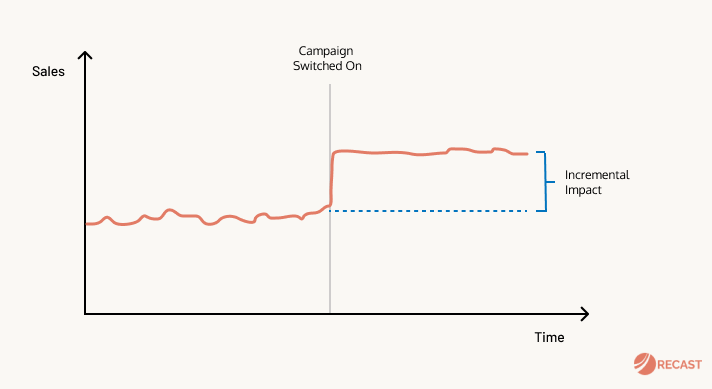
The easiest tool available to conduct such a study is CausalImpact, an R package that constructs a Bayesian Structural Time-Series model that is used to predict the counterfactual.
Actioning the results
Results from these quasi-experimental methods can be actioned directly by deciding to stop the channel if there’s no incrementality, or double down if the quasi-experiments prove a causal impact of the channel on the business.
Moreover, you can validate or calibrate your Marketing Mix Model with this data, for example in using Bayesian priors. This action allows us to improve the model predictions that will guide budget allocation decisions.
Let’s illustrate with a real-world example from my experience. A brand I worked with, planned to allocate a significant part of its media budget to Branded Paid Search in a new market they were launching. The company had already excellent organic rankings and competitors did not target its brand keywords. For these two reasons, I was sceptical about the incrementality of the channel on the primary metric: ‘Customer Acquired’.
To validate my hypothesis, I ran a geo-experiment splitting that market into smaller geographical units. The result: there was no significant incrementality at all. We did not roll out the campaign, and routed the saved budget to other channels.
In my case the campaign hadn’t yet launched, but this process can easily be modified if the channel is already up and running, by testing if switching it off has a negative impact on the business outcome. Either way, the costs of failing to run such a test can be significant.