Business environments are messy; people with different responsibilities need to work together on decisions quickly and without perfect information. That means that sometimes the statistically perfect approach isn’t the best one; faster or less-risky methods that are just good enough can work better than a fully reliable approach that takes several months.
A recent post on Recast looked at why brand search incrementality tests are important and detailed two of what are considered the best in class approaches: geo-testing and regression discontinuity design. In this post I’ll outline a recent brand search incrementality test carried out at shouldweswitchoffbrandads.com that nicely illustrates how business politics and specific limitations can lead to a less statistically perfect approach being the most effective.
The context behind the test
The client who commissioned this test operates globally in the medtech space. The product they sell has a low differentiation and is offered by multiple brands in each market so competition is tough, although it should be noted that this particular company is the market leader in Europe.
In one of their core markets, brand search CPCs had increased by 300% over a 6 month period as a result of competition bidding more aggressively. The business pays for around 250k brand search clicks each month, so even small increases here have a pronounced impact on the efficiency of their marketing. The question of whether this activity was profitable, or whether the campaign could be turned off, was raised several times before we were commissioned to help answer the question.
Limitation #1: Reducing the risk to conversions:
One reason that brand search incrementality tests aren’t carried out more frequently is the risk they pose to revenue; testing usually requires shutting off all or part of the campaign for a period of time. Businesses tend to be concerned that turning off campaigns that report strong last click conversion numbers could lead to significant losses if the conversions aren’t picked up elsewhere.
With this specific test, we were commissioned by the CMO and Head of Paid Search, who were directly responsible for the revenue of the company. Minimising the risk to revenue was a condition of the test being given the green light. We had to design the test in a way that minimised the time the campaigns were off. Initially the testing was limited to low volume days (Saturday + Sunday) and in a low volume market until we could show that there wasn’t a significant loss of conversions. A close monitoring of the site’s revenue was required during the test periods, with the test being halted if losses were too great.
Limitation #2: The company were wary about the competition
Another common concern businesses have with brand search incrementality testing is that by switching the campaigns off not only could they lose sales, but they could be gifting these straight to a competitor. As this client had a lot of aggressive competitors with similar products another condition was minimising the benefit to the competition. This meant avoiding the competition realising that we were running a test and pumping up their coverage of our brand’s name.
Limitation #3: The business model doesn’t fit Geo tests
Lastly, the business model of this client was also different from the usual ecommerce case. Their medtech product sometimes required a physical consultation with a medical specialist and the capacity for these differed greatly depending on the city. This also meant marketing activity was stronger or weaker in some areas. Finding two locations with a statistically similar performance and enough conversion data for the test wasn’t possible.
All of these limitations ruled out both of the two ideal approaches (geo-testing and a regression discontinuity design after a full switch off) so we had to design something more bespoke…
Test design and execution
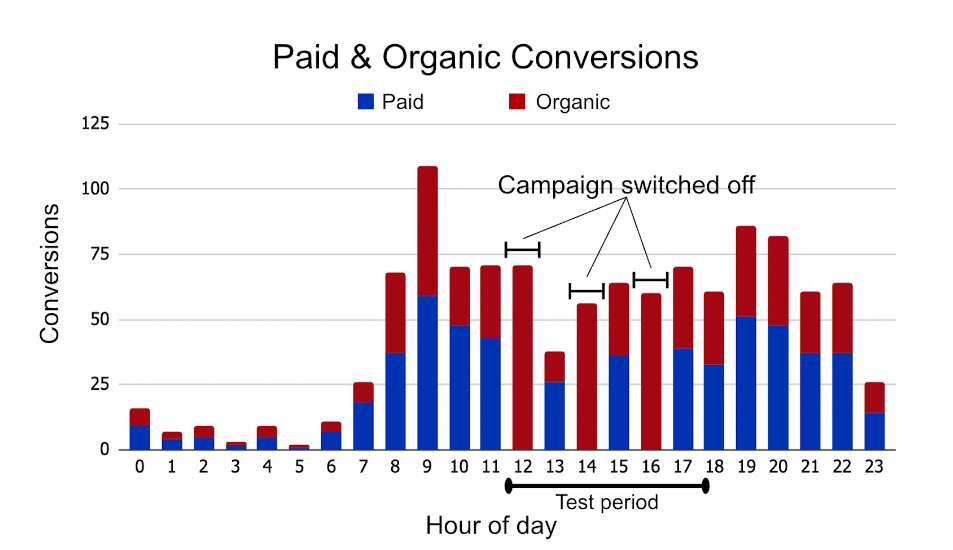
The final design was an on-off test, in which the brand campaigns were switched on and off each hour for a short period through the day. Similar to both the ideal approaches, this allowed us to create counterfactual points for the campaign being off. We started with two 6 hour periods per week, before expanding to five once we could provide the CMO with preliminary results to show there wasn’t any sizable loss to conversions.
We used automations that switched the campaign’s status over the testing period, while also monitoring and assessing the risk to revenue and competitor behaviour. After each test period, and throughout the following days, the automation would check that the CPC was falling within an acceptable range (with the hypothesis that competitor behaviour would change this metric before the auction insights were available). It would also check that there wasn’t a large loss of conversions on the search channel. If either of these metrics fell into predefined danger zones, we would receive a Slack message to nudge us to check out the data, or in extreme cases the test would shut itself off completely (thankfully we didn’t have to deal with the latter in this test).
This minimised the risk to revenue but also meant that the campaigns were only off for up to 3 out of every 24 hours. This led to a ~20% decrease to impression share on test days, a figure that was unlikely to be noticed by competitors during the test to avoid them changing their behaviour.
In total the test ran in 15 x 6 hour periods over a period of 5 weeks.
Analysis of imperfect data
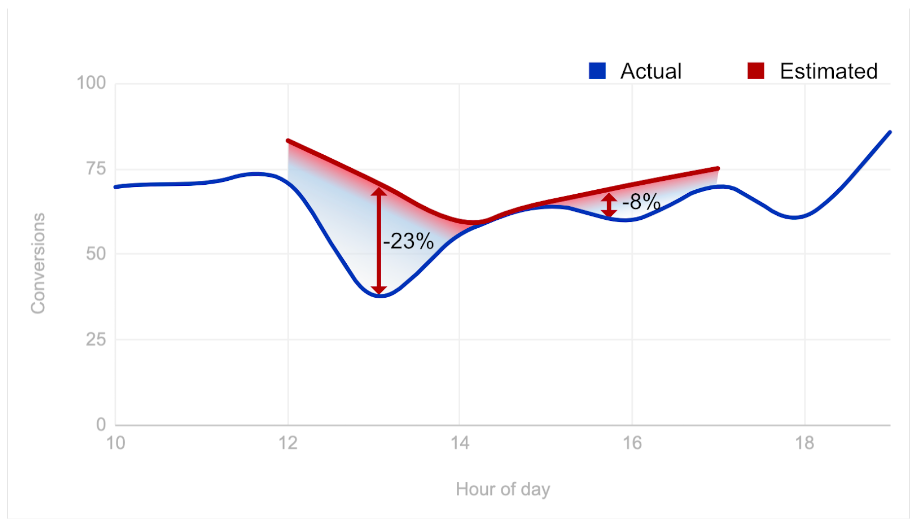
For the analysis we calculated counterfactual points for all the periods where the campaign was off. We did this by using quadratic interpolation to fill in the gaps using data from the hours before each campaign-off point, and historic trends in the campaign. The result was two conversion data sets – one for when the campaign was switched off, and one for what we think would have happened if the campaign was on. These were then compared to estimate the incrementality of the campaigns; we found it to be 11.5% over all test periods. This was used to calculate the cost per incremental conversion (CPiA) as €420 – compared to the €48 CPA reported by Google Ads.
The incrementality was consistently between 8% and 20% throughout the test, however the p-value ended at 0.3, with no improvement even after another week of testing was carried out. While this may have been not ideal statistically, the consistent low incrementality of the campaigns was enough to suggest that the campaign had a low incrementality, even if we could not reliably calculate that exact figure.
Recommendations and next steps
Our recommendation to the client was that they reassess the profitability of their campaign with the CPiA rather than CPA to make a decision on whether this should stay active. We found that some marketing spend could be recovered by simply switching off the campaign on certain days; with an approximately 40% reduction in spend leading to only a 1% decrease in conversions. Extending the test to switch on/off specific audiences or locations could help to find more low incrementality areas to reduce spend as well.
The results allowed the client to look at the campaign like any other prospecting campaign by comparing the CPiA to the LTV of acquired customers (which was pretty high given the cost of their products). Even though the CPiA was much higher than the CPA reported in the Google Ads interface, the figure was still efficient for them given their high LTV – and was relatively low compared to some other prospecting campaigns. This would still be the case at both the upper and lower bounds of incrementality we saw through the test. They decided to keep the brand campaign on, but with the aim of taking advantage of the reducing the budget in the low incrementality days of the week. We’re also looking at further testing to find any other low incrementality cohorts to reduce the spend on.
Outside of the cost saving, having the decision made saves further time for the client because the question won’t be raised as often by the board. To further avoid any potential loss of conversions and to confirm the incrementality over time, the client also requested further tests at a pace of every quarter; this way any change in competition will mean the decision can be remade with the latest information. This also allows for optimisations to be made to the brand search campaigns that could reduce the CPiA.
So while neither of the statistically best test approaches were possible we were able to design a test that answered the problem they had, make a decision and then move on. Being flexible on the approach and allowing results that are good enough for the case at hand means that more decisions can be made. In this case we managed to reduce the client’s marketing spend as well as the time spent asking these questions and the distractions that worrying about the problem caused. Hopefully this is a good illustration of how the most statistically effective approach might not always be the best approach in a business setting.