In the world of website analytics, the concept of “dark social” has emerged as a significant challenge for accurate channel/source reporting. It refers to web traffic that is incorrectly attributed as “direct” in analytics when, in reality, it is driven by social networks and other hidden referral sources. This phenomenon has far-reaching implications for marketers who rely on traditional software-based attribution models to make strategic decisions.
A study by SparkToro of 1000+ visits showed that 100% of all visits from TikTok, Slack, Discord, Mastodon, and WhatsApp, and 75% of visits from Facebook Messenger were marked as “direct”.
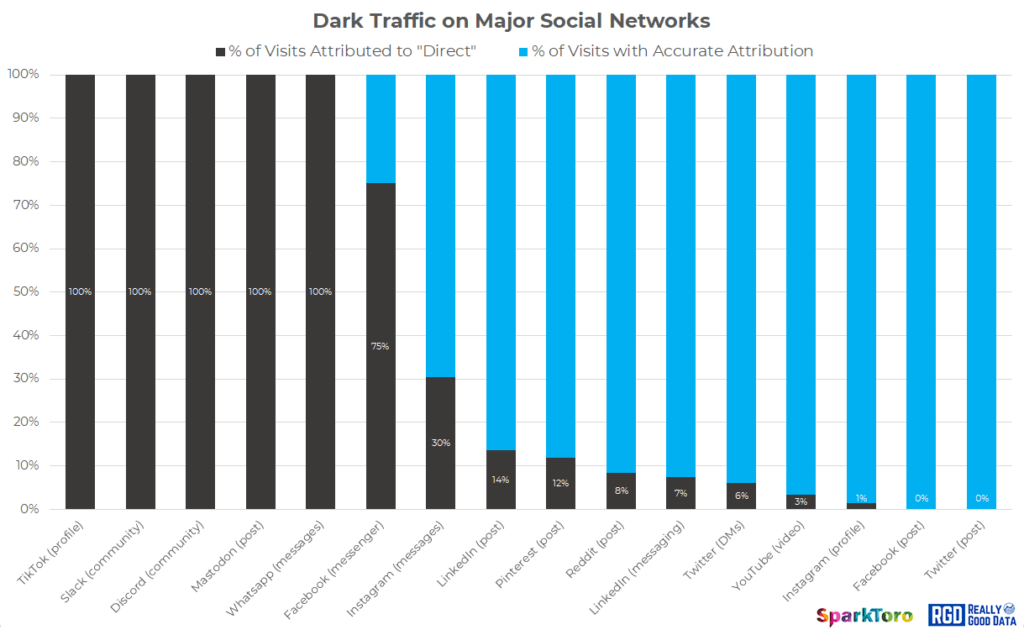
The results have been replicated by another study by Refine Labs. The 12-month study showed a 90% measurement gap between software-based attribution and self-reported customer data, specifically for dark social channels like social media, podcasts, and word of mouth.
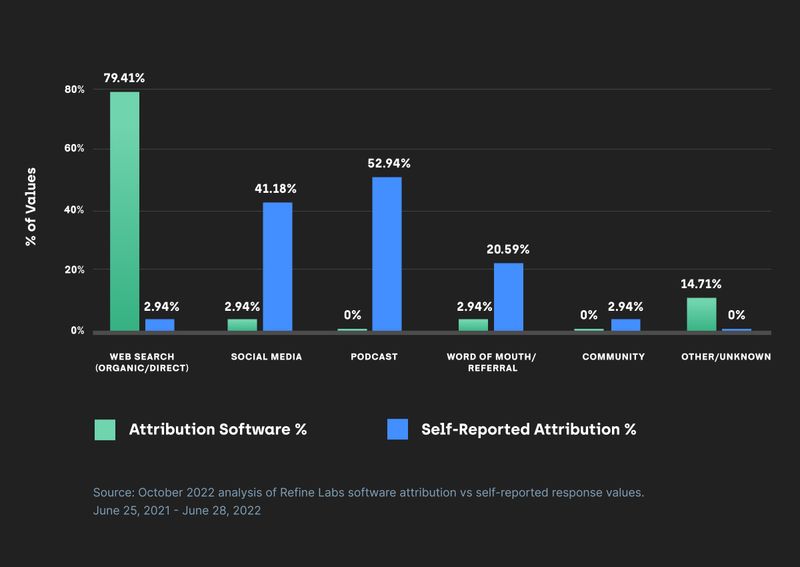
Why you need a “How did you hear about us” survey
It shows that there is a vast disparity between what software reports and what customer journeys actually look like. It is because while a user may go through direct and SEO channels to purchase (last-touch, as is reported by the software), they may have been influenced to purchase in the first place through channels like referral, podcast, and Twitter (which the customer reports). Focusing solely on software-reported attribution leads to prioritising channels that capture demands, but neglecting those that create it.
Hence, to gain a truer picture of what channels actually convert — and make optimal marketing decisions, companies must implement self-reported attribution or checkout surveys in their campaigns.
This is done by having a form question “How did you hear about us” before the Checkout page, or on lead magnet form fill, which can be hard coded by your product team or implemented using a vendor like (friends of Recast) Fairing.
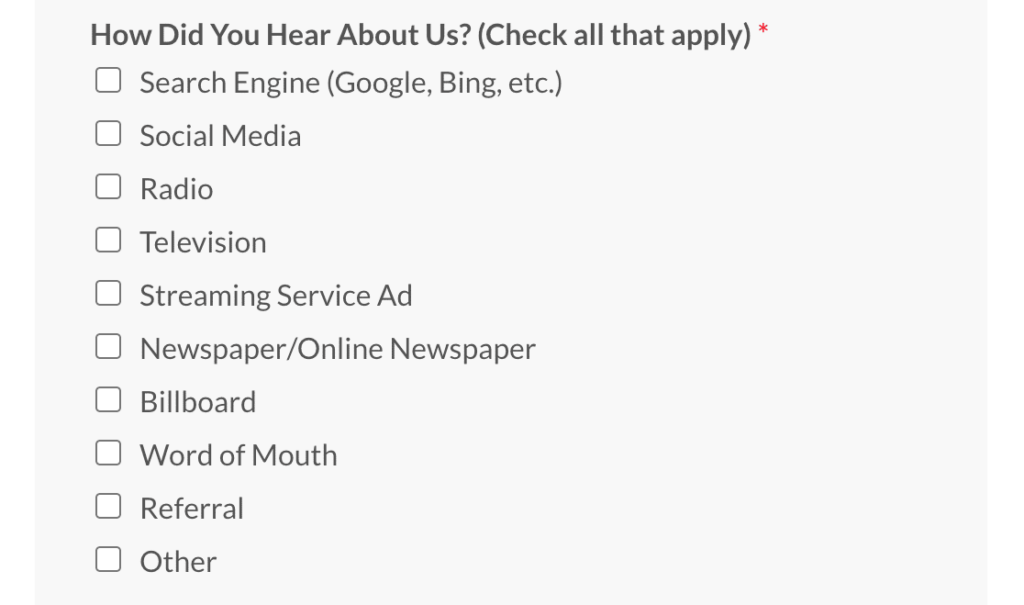
Best practices for the “How did you hear about us” survey
- Make it a required field, not optional You may be tempted to mark the field as optional so as to not create fiction among users to convert. But Chris Walker, CEO of Refine Labs says that it is just an excuse to not do something different — because if you don’t want to analyse the behaviour to purchase, then you are not really concerned about conversion. Moreover, when you make the field optional, nearly 30% of people do not fill out the form. This makes your data not thorough enough to base your marketing decisions on.
- Use a free-text field instead of a drop-down menu Providing predefined options in the form of a drop-down introduces biases and limits the range of responses. It is believed that people are more likely to select the first visible option (also called order bias) or lie on the form if their actual answer doesn’t exist in the list provided. However, most checkout surveys use the drop-down menu or a checkbox list. It is because it helps you categorise and quantify your survey responses. But you can get the best of both worlds by making it a free-text field where users can type in their answers as they want, and then building string-matching automation to automate the categorisation and quantification of the responses. String matching is a process of finding one or more occurrences of a particular pattern or substring within a larger string or text. It involves comparing the characters of the pattern with the characters of the target string to determine if a match exists. Once you set up the survey, you may manually check what kind of responses you are getting for the first few days. You would notice a pattern in how people mention sources or channels. For example, if someone mentions “LI”, then you can automate it to show “LinkedIn” in the backend. If you have a podcast, then a response that mentions the word “podcast” or the name of your show, you automate it to show “Podcast”.
Limitations of ‘How did you hear about us’ survey
However, just like any other attribution method, self-reported attribution also has its limitations.
People often struggle to accurately recall how they initially heard about a business, and their responses may be influenced by recency bias, favouring more recent touchpoints.
Moreover, customer conversions typically occur after multiple touchpoints, making it possible to overlook the impact of certain channels that may not be reflected in form submissions. Expect self-reported attribution to favor more emotional or memorable channels like TV, which actually might be ok, given that most other forms of attribution are biased towards lower funnel channels like PPC (Pay Per Click).
Hence, basing your marketing strategy solely on checkout survey results is as damaging as focusing just on software-reported last-touch attribution. For example, if you stop investing in SEO or paid media after seeing form submission results primarily say organic social or podcast, you will be missing out on retargeting the good-fit traffic and capturing the low-hanging fruit. The effort and resources that went into community building would go to waste.
Embracing a multi-level reporting approach
Different channels serve different purposes. Most marketing requires a healthy marketing mix, with each channel significantly impacting some part of the customer journey. Hence, businesses should embrace a multi-level reporting approach that combines software-generated data with self-reported attribution.
By integrating checkout surveys, you will be able to measure the impact of dark social channels that are difficult to measure in most other ways.
Alternatively, you can also try more sophisticated measuring techniques such as:
- Causal Inference: Replicate a laboratory experiment through techniques such as natural experiments, regression discontinuity, or equivalent methods.
- Marketing Mix Modeling: Employ multi-variable regression techniques or advanced algorithms like Bayesian MMM, to assess the influence of each marketing channel.
- Geographical Test: Randomly assign a portion of geo-regions to undergo changes, and measure the variation in sales between control and test.