There’s a commonly cited Neilsen study that revealed that 92% of consumers trust referrals from family or friends more than any other type of advertising or referral. If you read that report and think “I wonder how much of my traffic comes from word of mouth”, well it’s actually not that easy to find out.
You can see traffic from social media sites in Google Analytics, but the truth is that sharing publicly on social networks is just the tip of the iceberg. Private sharing via Whatsapp, Email or Slack account for as much as 69% of traffic according to Alex Madrigal of the Atlantic. We can’t easily isolate this traffic in analytics, because these platforms don’t pass a marketing source or referrer: they show up as “(direct) / (none)”.
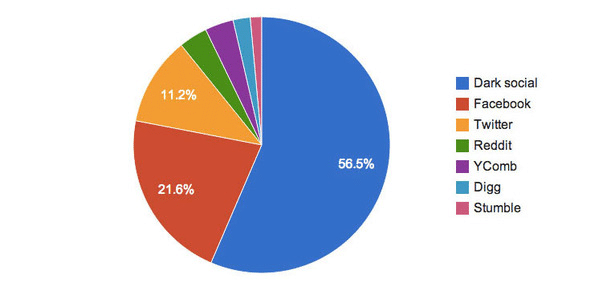
This isn’t really a ‘channel’ as such: more of a bucket to throw any traffic that we don’t know anything about. This is essentially any traffic that you didn’t add UTM parameters to, where the platform they clicked from doesn’t pass a `document.referrer` when requesting the page from your website server. There are lots of places the traffic could have come from that would exhibit this behavior:
- Browser bookmarks
- Click on a URL with broken UTM parameters
- Links in PDFs or other documents
- Click from a locally installed software program
- Clicks from emails without UTM parameters
- Manually typed in the URL
- Copied and pasted the URL from a document
- Traffic from mobile apps
- Fake / spam traffic from bots
- Clicks on shortened URLs
- Clicks from a secure HTTPS page to HTTP
- SEO traffic from logged in users
- Links shared in WhatsApp, Zoom, Slack, etc
Only some of these sources would count as Word of Mouth, so you need to eliminate or account for them if you’re going to truly measure its impact. Some studies found that as much as 80% of all of your sharing happens through dark channels, and with increasing pressure to curtail divisive public posting, even more word of mouth is likely to go dark. Group chats are the new social networks, so it’s important to find a way to separate this traffic out.
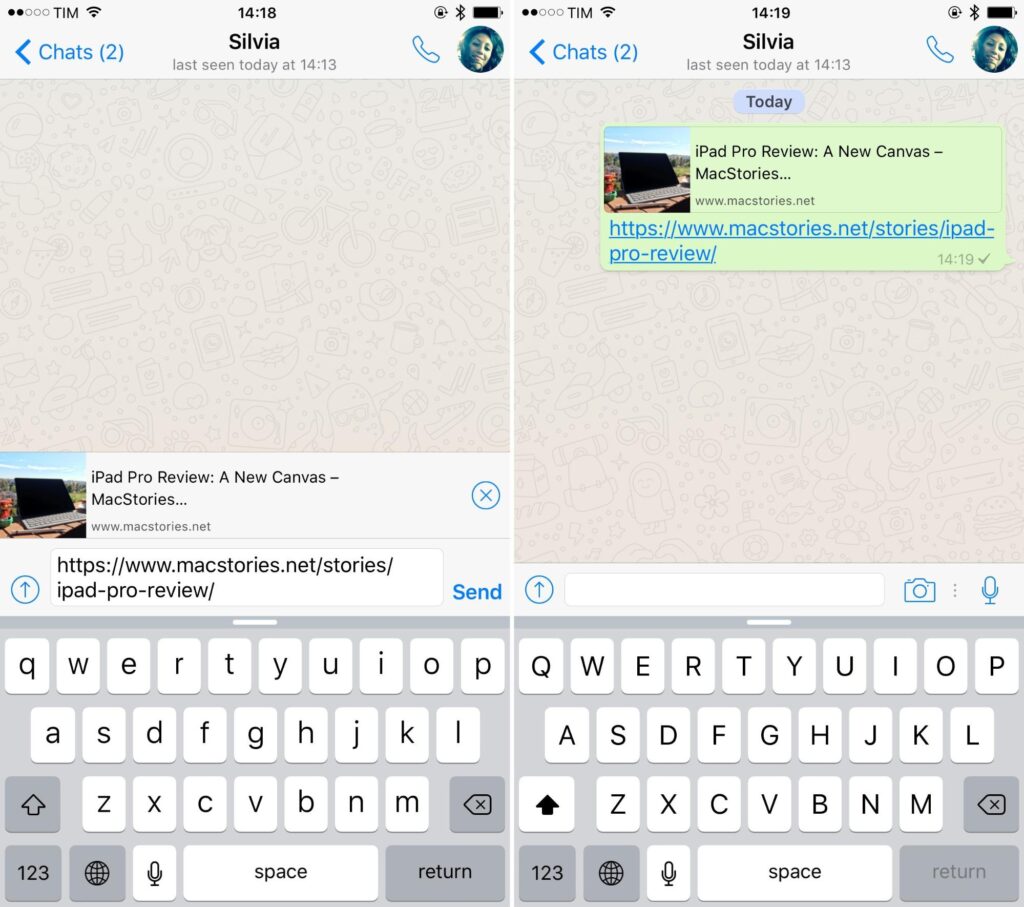
However it’s hard to unpack how much of your direct traffic comes from each of these non social sources (or the countless others not listed). It’s different for every brand, and often requires in some cases quite creative methods of investigation.
Tag Everything
The obvious first thing to do is to make sure all of your marketing resources have UTM parameters correctly tagged. Go through every PDF, PowerPoint, Excel file, and other documents that are public facing, and make sure each has utm_source, utm_medium, and utm_campaign. If you don’t want a long URL, you can always add the tags and then run the URL through a link shortening service. Also go into the settings of your email provider and make sure auto-tagging is turned on, so every email has tags by default. It’s also worth checking your paid advertising campaigns.
Location Data
I once talked to the head of growth at a prominent web browser company, who found out that the big spikes in direct traffic they saw every September turned out to be teachers downloading the browser onto computers in the IT lab, ready for the new semester. They figured it out by looking at the IP addresses and seeing they correlated with the locations of schools.
User Surveys
Sometimes if you want to know where your users are coming from, you just have to ask them. A friend of mine that worked with software used in schools surveyed users and found that much of their direct traffic came from teachers sharing presentations in the staff rooms of schools: they’d click the links in the notes and show up on the website as direct traffic. Once you understand user behavior you can influence it, so for example they gave teachers flyers with unique referral codes so they could track that source separately.
Page Types
Another trick is to take a look at the URLs they’re visiting. If they’re visiting the homepage, well that’s essentially brand traffic: the equivalent to searching for your brand term. If the link is to a blog post or product page that wouldn’t be easy to type in, it’s more likely to be social sharing: someone copying the link and pasting it into an instant messenger app.
Experiments
One way you can tell for sure is to run an experiment, for example Groupon’s wild experiment where they delisted their website from Google! They learned a valuable lesson, which was that at the time around 60% of their direct traffic was actually from SEO. Note how they broke the results of the test down into different page types as well, and found traffic to long URLs was affected far more by this issue.
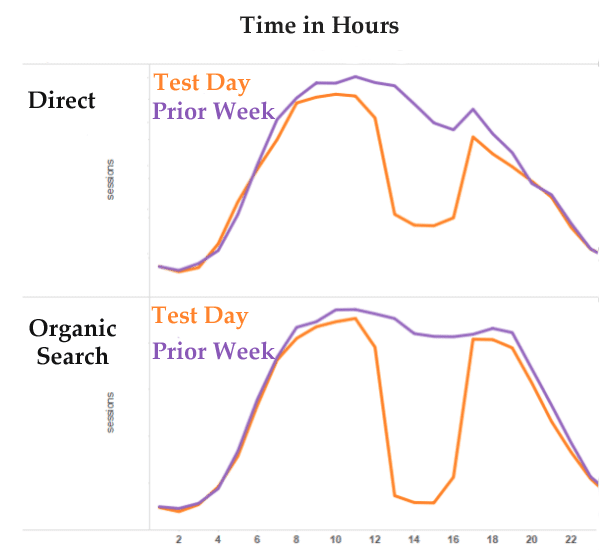
Link Tracing
One interesting way to track link sharing came to me during the COVID pandemic when the media were talking about contact tracing. I figured out if I wrote a custom script to store a User’s ID in local storage, and then update the URL hash (the optional part of a URL that starts with # you see on some websites), then when they share the URL with someone, it would have their ID in the URL hash, and I could tell who it came from.
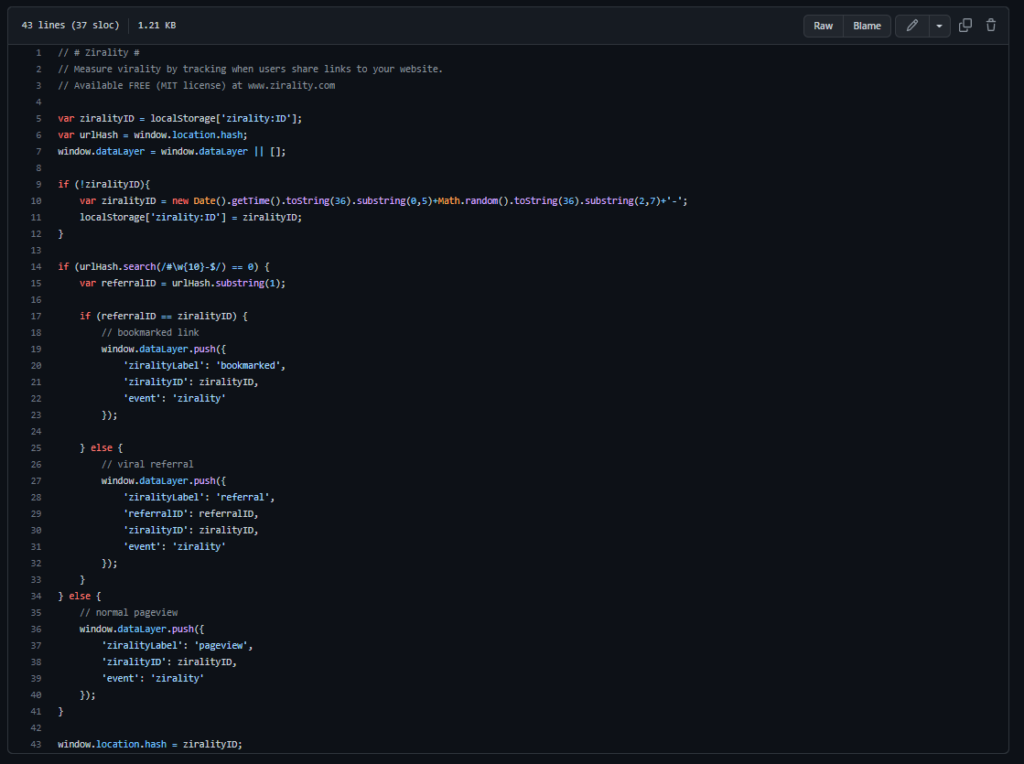
When I tested this out on a few different websites I found that something like 10-20% of direct traffic could be recovered as link sharing. I also was able to measure the average virality of different articles and optimize that, rather than strictly optimizing for SEO. There are some browsers like Safari and Brave where the tracing gets screwy, and it doesn’t work on mobile apps, but it worked well enough to find out a few interesting insights.
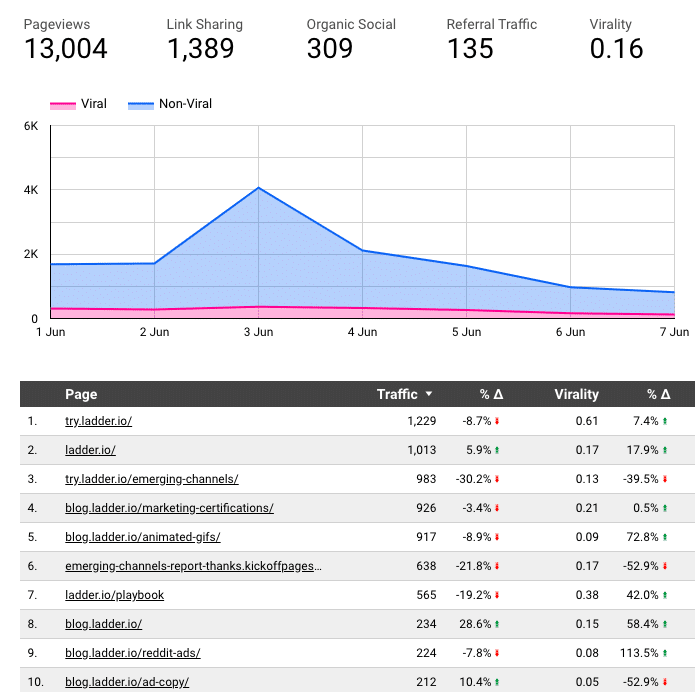
Word of Mouth Coefficient
Another word of mouth measurement project I worked on was the WoMCo, or Word of Mouth Coefficient, a term coined by my co-author Yousuf Bhaijee. Formerly at Zynga, he modeled it off a metric they used internally to measure the virality of a game. It works by taking new direct users and correlating them to active users: the finding being that word of mouth is often a function of retention, or how many users were actively using your product.
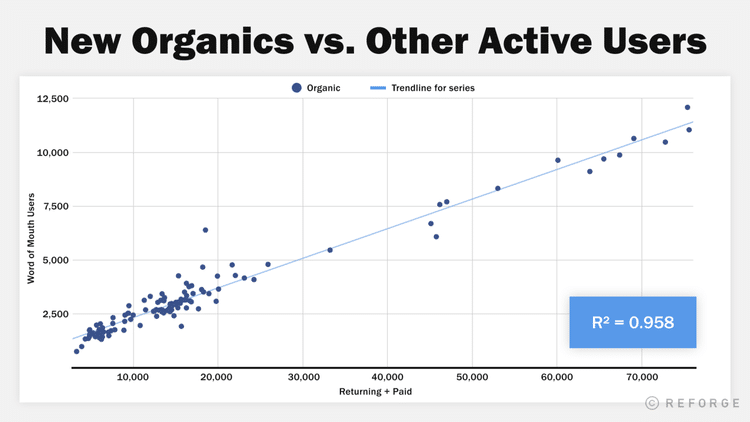
This method should be paired with the methods above, because the correlation between active users and new direct users is definitely stronger once you’ve stripped out any non word of mouth traffic. What we’re doing here is using linear regression to establish the relationship between active users and new direct visitors, and if the relationship is strong, that’s a sign we have successfully identified what are word of mouth users versus users from other sources. If the correlation is weak, it’s worth revisiting some of the other techniques listed.
Marketing Mix Modeling
Word of Mouth Coefficient used linear regression with a single variable, but it’s often impossible to strip out all of the potential causes of direct traffic that aren’t word of mouth. Sometimes your marketing mix is too complex, and you need to account for more than one variable. Linear regression with more than one variable is called econometrics, and when it’s applied to marketing it’s called Marketing Mix Modeling, which is what powers Recast. This method can be less costly than running an experiment, and it can be run more regularly to guide your budget allocation decisions. However it can also give less granularity than these other methods, so it’s worth combining multiple techniques to triangulate the truth about the impact of word of mouth.