Marketing Mix Modeling’s main goal is to find the incremental return of every marketing investment – how many sales did each channel drive that we wouldn’t have gotten otherwise? If you can find true incrementality, you can use it to optimize your budgets, get the right channel mix, and get rid of wasted spend.
The problem is: how can you validate your model? How do you know that the data you’re seeing – from a consultant, an open-sourced model, or a vendor like Recast – is accurate and not misleading you?
There are three main ways to validate your models:
- Conversion lift studies
- Holdout forecasting
- Dynamic budget optimization and evaluation
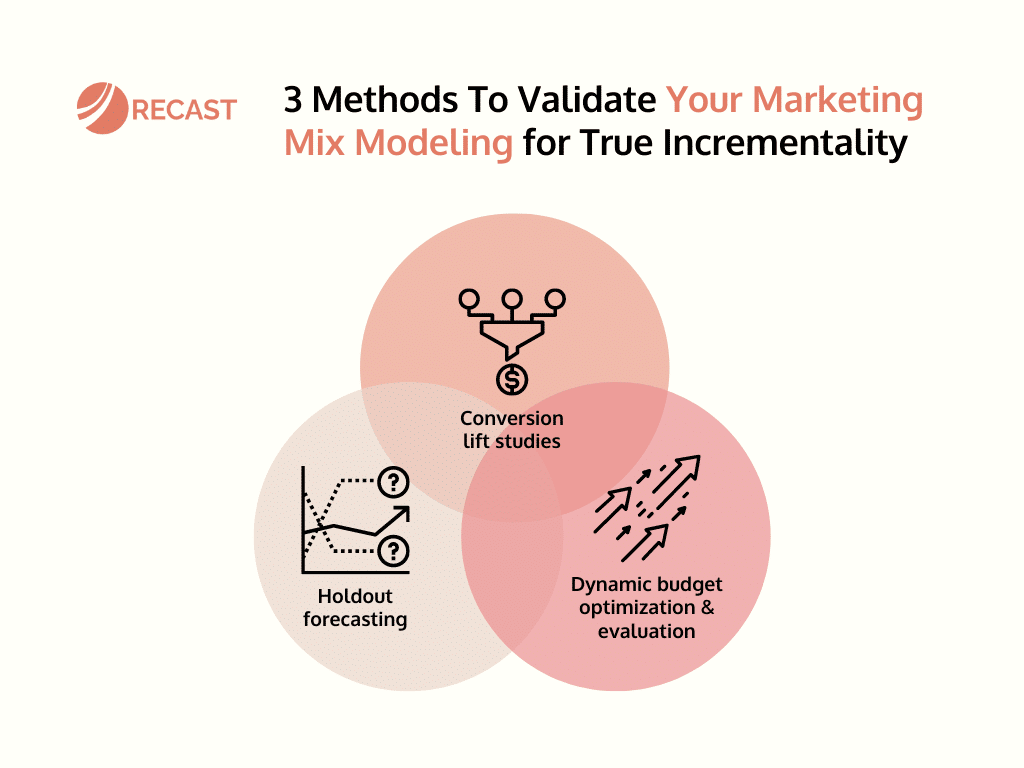
We will cover them in this article in detail, but let’s start with the core challenge of Marketing Mix Modeling that makes this so complicated in the first place.
Causal Inference and MMM:
We don’t just want to know what variables are correlated with sales. We actually want to understand the causal relationship between the two. And causal inference is a much harder problem to solve.
MMM uses statistical methods that are nondeterministic: you can get different results given the same inputs. The actual truth is not known – I know, disappointing.
What we can do is reduce our uncertainty in how our marketing is performing, but it comes with an increasingly complex system with many variables that are beyond our control. There are so many things that can change how effective our marketing is that are completely out of our control, and that truth is always evolving.
Because of that complexity, there are an infinite number of models that fit the data equally well, but that give different conclusions:
Say one model tells you to increase spend on Facebook and decrease spend on paid search. But the other model tells you the exact opposite thing. However, both of those models can fit the data equally well.
How are we supposed to know which model is right?
How to (not) validate your MMM:
Before we cover how to validate your MMM, let’s take a page out of Ghostbusters and dispel some myths around any preconceived notions out there.
- We’re not relying on checks of statistical significance. Although that can be useful when you’re running a hypothesis test, for example, comparing the difference in means between two groups, that’s just not what we’re doing with MMM.
- We’re not looking at in-sample goodness of fit metrics like R squared, mean absolute percentage error, root mean squared error, normalized root mean squared error, etc. They are too easy to manipulate with dummy variables just to increase the accuracy of the model.
- We’re not doing cross-validation. These are typically used with many machine learning methods but we can’t easily do that with MMM because we’re dealing with time series data, and that violates the assumption of IID.
Most importantly, none of these techniques actually differentiate between correlation and causation, which is what we truly want to understand. So, what does work?
How to validate your MMM:
Before we cover the three methods, a quick caveat: they’re not mutually exclusive and, when possible, you should use all of them to triangulate how your model is performing.
1 – Conversion Lift Studies
Conversion lift studies – geolift experiments, holdout tests, randomized control trials— are our ground truth. They are the gold standard for understanding causality, and we can use this to calibrate our models.
Your MMM should be set up to measure incrementality, which means that it should be validated by these other tests that you run outside of the model. If you’re using a legacy MMM, you might have 10-20 models with good fit metrics yielding different results. Then, you can winnow down that set of models to the ones that are consistent with the experiments that you’ve run.
If you’re using a Bayesian framework you can actually include the information from those tests in the priors of your model to calibrate it and push the modeling framework towards the set of models that are actually consistent with the other sources of ground truth you have.
A quick note here: when an MMM and a well-run experiment disagree, the well-run part being very important, it’s normally the MMM that’s wrong. The model is making a lot more assumptions than the experiment so when you have experimental data that conflicts with your MMM, go take a look at why the model might be wrong.
2 – Out-of-sample validation / hold-out forecasting
Out-of-sample validation is something we put a lot of thought into at Recast. The idea is to test the model’s predictions on data that it hasn’t seen before.
In very simple terms, we’re going to “chop off” some amount of our data, train the model until a period of time (say three months ago), and then we’re going to ask that model to make a forecast about what’s going to happen over the next three months. We’ve seen the data, but the model hasn’t so we can compare its results to the actual numbers.
This is similar to cross-validation, but we need to make sure we’re actually doing holdouts at the end of the data because of the time series component. If you just drop out one random week in the middle of your data set, that’s not a good enough check because the model has a bunch of other information about what happened before and after. In order to get confidence in the model, it can’t be overfit to the data that it has already seen before.
Additionally, if the model can do it consistently over and over again in the face of interventions and changes in your marketing budget, that’s going to give you really good evidence that you’ve picked up the true causal signal.
3 – Dynamic budget optimization and evaluation
This rhymes with our final point: changing your budgets to proactively evaluate the model’s ability to predict the results. If we get results from the MMM that says TV is our best channel and Facebook is our worst channel, we should be able to test that the following month. Let’s put some more money into TV and take some more money out of Facebook to see if the model continues to predict accurately and if our overall marketing efficiency actually improves.
MMM has often been considered static: every 3-6 months we get the results and maybe we implement them. But with Recast updating the model weekly allows marketers to use it more dynamically – opening the opportunity to run tests more often and use them to audit the model.
TLDR:
MMM looks to find the true incrementality of your marketing dollars but, because we’re looking for causal inference, we must validate if our model is giving us the right information. There are three main ways to validate your MMM: conversion lift studies, holdout forecasting, and dynamic budget optimization and evaluation. We don’t recommend using them in silo but, instead, in coordination to get a more clear picture.