Marketers are often very focused on the future as their jobs rely on anticipating and meeting the needs of consumers in a rapidly changing market environment. However, to understand the future, it is crucial to look to the past. This post will focus on understanding the history of econometrics to give marketers an insight into the wider context of trends that have shaped the field and influenced how marketing is measured. Having a clear comprehension of the historical evolution of econometrics enables those who use it to critically assess its capabilities and limitations, allowing practitioners to make more informed decisions on how to measure marketing activity in the future.
What is influencing the evolution of econometrics?
Econometrics as applied to marketing has undergone significant changes since its inception, and its evolution has been shaped by various factors. By considering four key areas of influence, we can gain a deeper understanding of how econometrics has changed over time:
- Societal changes. As society has changed, so have the needs and preferences of consumers, and econometrics has had to adapt to meet these changing needs.
- Trends in the advertising industry. As media channels emerge and marketing techniques have evolved, econometrics has had to keep pace, developing new methods for measuring the effectiveness of marketing.
- Technological progress. The increasing availability of data, combined with advances in computing power, has allowed econometricians to analyze large datasets and develop increasingly sophisticated models.
- Advancements in statistical and economic theory. New theories have led to the development of more sophisticated models, and have helped to improve our understanding of the underlying relationships between variables.
The era of traditional econometrics
Informed by statistical and economic theory from as far back as the 1700s, the 1960’s began the era of ‘traditional econometrics’, a period that lasted until the end of the 1990’s. The below timeline gives an overview of the factors that influenced the emergence of econometrics during this period.
Society | Advertising industry trends | Technology | Statistical and economic theory | |
---|---|---|---|---|
1700s to early 1900s | Claude Hopkins Publishes “Scientific Advertising” in 1923, documenting his use of split-testing based on redemption of coupon codes. | Economists theorized that once a certain level of optimal capacity has been achieved, adding another production factor will result in decreasing marginal returns and smaller increases in output, known as the law of diminishing marginal returns. Statisticians establish regression analysis, a method for analyzing the relationship between a dependent variable and one or more independent variables. | ||
1960s to 1980s | TV has become the dominant mass media. By 1960, 45.7m households in the US had television sets. | Thanks to the opportunities created by TV advertising, there was an increase in campaigns with brand building objectives, creating challenges on how to measure effectiveness. | In 1960 E. Jerome McCarthy wrote Basic Marketing: A Managerial Approach, outlining the 4 P’s- product, price, promotion, and place. This framework established a ‘marketing mix’ approach and practitioners needed to understand the impact of each element on marketing outcomes. Statistical Analysis System (SAS) began as a project at North Carolina State University’s agricultural department in 1966, later branching out and becoming widely used for econometrics. Advertising adstock theory from Simon Broadbent in 1979 suggests that the impact of advertising on consumer behavior is proportional to the amount of advertising exposure and decays over time. | |
1990s | The internet grew quickly into a mass medium. By 1995, the popular browser Netscape Navigator had around 10 million global users. | Media buying was widely unbundled from creative agencies into media agencies, creating more focus on media measurement. | Microsoft Excel was launched in 1985 but it was the addition of the Data Analysis Toolpak in 1992 which expanded the pool of individuals who could engage in econometric analysis. Eviews, a Microsoft software package for statistical analysis and econometric forecasting was released in 1994. Fuelled by growth of the internet, coding languages such as Python, SQL, and R become more mainstream. |
Overall this period was characterized by a rapidly changing media and technological environment. Econometrics emerged to measure the impact of marketing efforts on sales and revenue during the ‘Golden Age’ of advertising starting in the 1960’s. The early approach to econometrics during this time relied heavily on linear regression, which for the first time explored the correlation between advertising spend and sales. As new theories emerged, such as the 4P’s and advertising adstocks theory, econometric practitioners were able to include these insights into the framework to improve the overall accuracy of the models.
During the 1990’s technological advancements such as the release of the Analysis ToolPak for Microsoft Excel and the Eviews software package opened up econometrics to a wider base of potential users. The emergence of the internet as a mass medium and the unbundling of media buying into stand alone media agencies put even more focus on measuring marketing effectively.
Whilst the ‘traditional econometrics’ of this era provided insights into marketing effectiveness that had not previously been available, it was a costly endeavor that required the services of highly skilled econometricians and statisticians to create custom models through manual processes. The approach involved meticulous adjustment of inputs and assumptions, which made it so expensive that even large companies would typically only construct a model once a year. As digital marketing became the dominant medium, the needs of marketers changed and this pace became too slow, leading to the next chapter of econometrics, the age of Marketing Mix Modeling.
The modern era: Marketing Mix Modeling (MMM)
The ‘econometrics’ of the 20th century evolved into a more sophisticated approach with the dawn of the new millennium in 2000. For clarity, and to differentiate from the previous era, we’ll refer to this era as the age of Marketing Mix Modeling (MMM), it has replaced the term Econometrics for most modern practitioners. Many of the fundamental concepts of econometrics remain the same, but driven by huge change across society, the advertising industry, technology and theory, MMM has emerged to better fit the needs of modern marketers who rose through the ranks during the rise of digital advertising, which eclipsed all other forms of media in terms of advertising budget share.
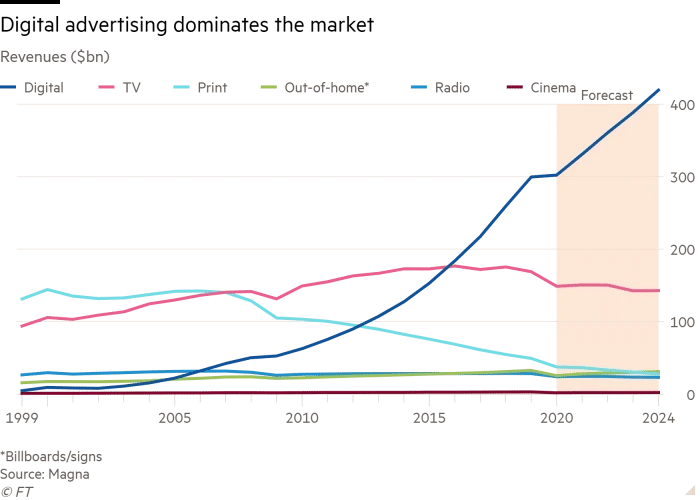
Society | Advertising industry trends | Technology | Statistical and economic theory | |
---|---|---|---|---|
2000s | Google Adwords launches, popularizing the auction model for online advertising in late 2000. The release of Facebook Ads’ release in 2007 leads to increased availability in hyper targeted / measurable ads online. | Fuelled by growth of the internet, coding languages such as Python, SQL, and R become more mainstream. SaaS (Software As A Service) trend begins, increasing accessibility to advanced tools used via the web browser The first ad blocking software was released in 2006. | ||
2010s | Emergence of DTC brands such as Warby Parker in 2010, Harry’s in 2012 and Glossier in 2014, who pioneered data-driven strategies. The EU’s new data protection law, GDPR, came into effect in 2018. In 2018 the Cambridge Analytica Scandal broke, leading to an increased consumer awareness on digital privacy. | Digital becomes the largest medium by adspend, overtaking TV in 2015. Marketers question the reliability of platform data, thanks to announcements on errors being found in data reported from companies such as Facebook | Stan, an open-source software library for Bayseian statistical modeling, was released in 2012. PyMC, a Python library for probabilistic programming that allows users to easily create, run, and analyze Bayesian models, was released in 2013. Recast was founded in 2019 by Michael Kaminsky and Thomas Vladeck. | Google released papers exploring Bayesian methods for MMM in 2017. Binet & Field published research in 2018 focussed on the importance of brand building. |
2020s | In 2023, California’s data privacy rights act, CCPA, came into effect. | Some large advertisers such as AirBnB and Adidas announced a shift back towards brand building objectives vs performance. The emergence of new, harder to measure media channels such as influencer marketing with $16.4bn in 2022 and podcasts with $2bn in 2022. Digital adspend is forecasted to be worth 69% of total media adspend in 2023, making it significantly larger than all the other media combined. | Major changes in data availability occurred in the market, thanks to Apple’s 2020 iOS 14 announcement and Google’s 2021 3rd-party cookie depreciation announcements. Facebook Robyn, an open-sourced and semi-automated automated MMM package, was launched in 2021. Uber Orbit, a Python package for Bayesian time series forecasting and inference, was launched in 2021. Google Lightweight MMM, a Bayesian library that allowed users to easily train models, was released in 2022. | In 2021 causal inference, which focuses on understanding of when causal relationships can be established, won a Nobel prize. Rise in the use of cross-validation and new error metrics such as NRMSE in data science practices, helping practitioners build accurate and reliable machine learning models. |
This period has been heavily influenced by the growth of the internet as the dominant media channel across the world. As eyeballs went online, new ways of targeting users online initially created a trend towards performance advertising and a need to closely measure and attribute. The emergence of DTC brands accelerated this approach as they pushed the envelope of what could be done with more data-driven strategies. Interestingly, the focus on performance objectives has in more recent years shown signs of reversing as some large brands pivot back to brand building objectives, creating a new set of measurement challenges.
Privacy became a central issue thanks to GDPR, CCPA and the Cambridge Analytica scandal. During this privacy-first era there was an almost overnight decline in the availability of data such as third-party data and device IDs that marketers had previously relied upon to measure effectiveness, creating a need to find new approaches to measurement.
Fortunately, great strides have been made in MMM to help fill some of these gaps for advertisers. The release of libraries such as Stan, PyMC and Google Lightweight MMM make it easier to create and use complex statistical models by allowing users to perform Bayesian inference. Recently this has further evolved thanks to the power of automation, where we see MMM moving towards more advanced algorithms such as those used by Meta Robyn and Recast. Crucially, marketers have also automated their data pipelines, increasing data availability and decreasing the resource needed to collect and clean this data. Overall these changes make it easier, quicker, and cheaper to run MMM than ever before, with less human error.
The Future of Marketing Measurement
“The more you know about the past, the better prepared you are for the future” – Theodore Roosevelt
Undoubtedly, the evolution of MMM is ongoing, just as the wider context of society, technology, advertising, and economic and statistical theories will continue to change. As a flexible framework, MMM is capable of adapting to these changes and providing answers to crucial marketing effectiveness questions. With the increasing automation in the industry, MMM will become more accessible to marketers of all sizes, even those who previously may not have been able to make the necessary investment. This will democratize access to measuring marketing effectiveness, enabling advertisers of all sizes to make informed decisions about their marketing spend. Overall, the ability of MMM to adapt and evolve along with the changing landscape is what makes it a valuable tool for marketers in the long term.
If you’d like to chat about how Recast can help your brand eliminate wasted marketing spend and find true incrementality, let’s talk.