If you’re considering working with a marketing mix modeling vendor, you’ve probably heard of Google’s open-source MMM tool, Lightweight MMM, Meta’s Robyn MMM, and our very own MMM platform here at Recast.
Now, we know that choosing an MMM is a complex decision that has many variables you have to look at before you decide which vendor is best for your company.
We also know that we’re obviously biased towards Recast, but we will try to be as objective as possible in this article comparing all three potential MMM vendors: Lightweight MMM, Robyn MMM, and Recast.
Let’s get started:
Operationalizing MMM
Building a great MMM model is hard enough, but we’ve seen that sometimes the hardest part is to make sure your marketers can take action off the results that the model outputs.
The most critical part of a successful MMM implementation is getting marketers to actually use the results to drive improved performance in marketing efficiency.
Unfortunately, most MMM tools are designed for data scientists and not marketers, so an MMM implementation falls flat when it comes time to actually take action. Below, we discuss some of the differences between Recast and Lightweight MMM and how Recast focuses on making the results actually actionable.
Validation
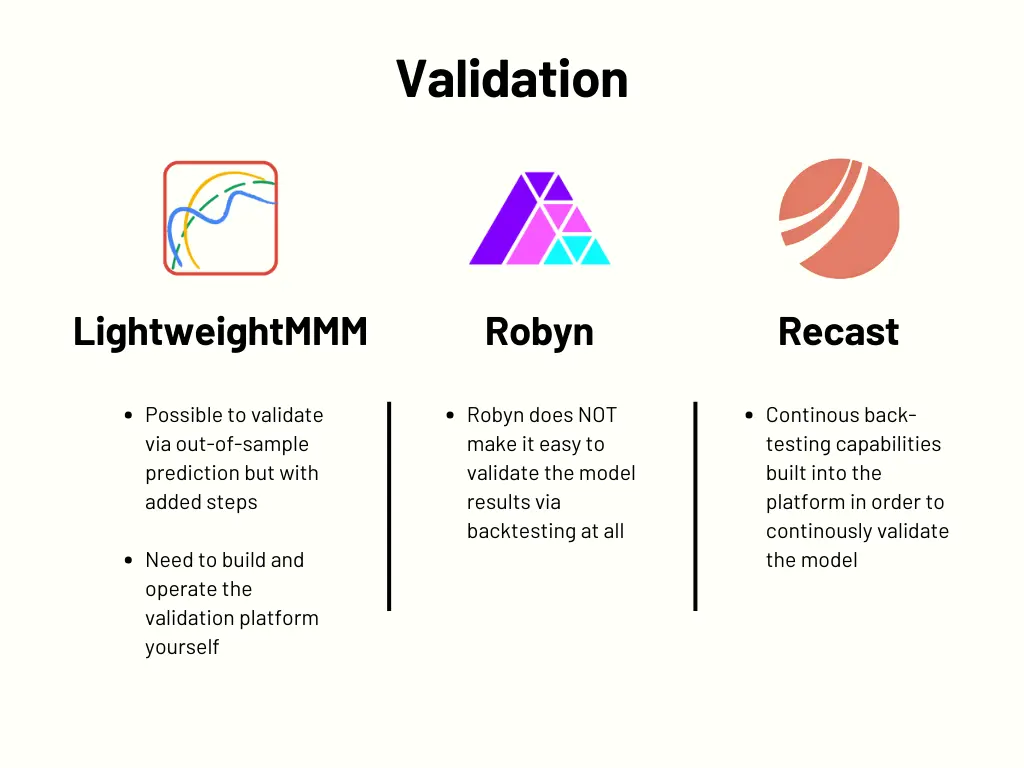
Validation is critically important to a successful MMM project. Marketers need to trust the results of the model and know that when they are following the MMM recommendations it will lead to improved performance for the business.
At Recast, we believe that the best practice for validating an MMM model is through continuous back-testing. That is, we use a model run through a certain date (say, June 1st) to make predictions about data that the model hasn’t seen yet (say, from June 2nd to August 31). If the MMM has correctly estimated the true incrementality of the different marketing channels, then the MMM should be able to make accurate predictions about data it hasn’t seen yet.
While it is possible to validate the Lightweight MMM model via out-of-sample prediction, you need to build and operate the validation platform yourself instead of relying on Recast’s built-in validation tooling.
Robyn does not make it easy to validate the model results via backtesting at all. This makes it very difficult for marketers to truly trust the results and feel confident taking action on them.
Data Science Resources
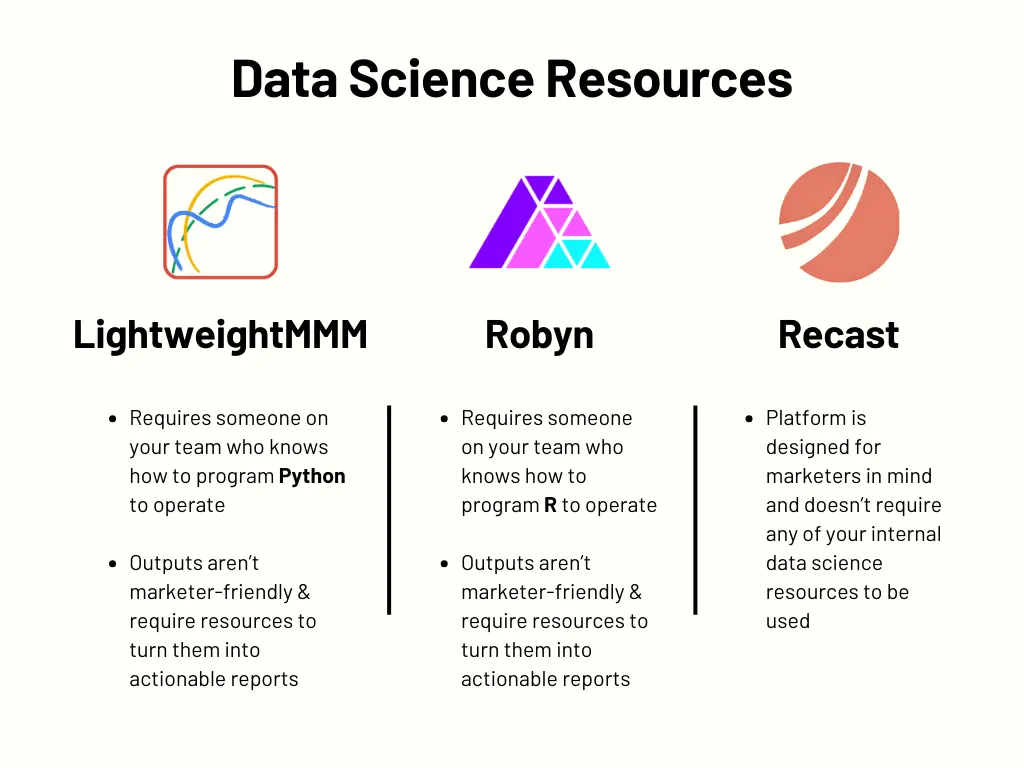
Lightweight MMM is developed as a Python package and requires someone on your team who knows how to program Python to operate it. Additionally, Lightweight MMM’s outputs are not marketer-friendly so you will need to invest substantial resources in taking the output from Lightweight MMM and turning that into reports that marketers are familiar with.
Robyn is developed as an R package and requires someone on your team who knows how to program R to operate it. Additionally, Robyn’s outputs are not marketer-friendly so you will need to invest substantial resources in taking the output from Robyn and turning that into reports that marketers are familiar with.
Recast is a full-service MMM platform designed for marketers. Our data science team is in charge of the initial model build and ongoing monitoring of data quality and model performance without needing any of your internal data science resources.
Tools for Marketers
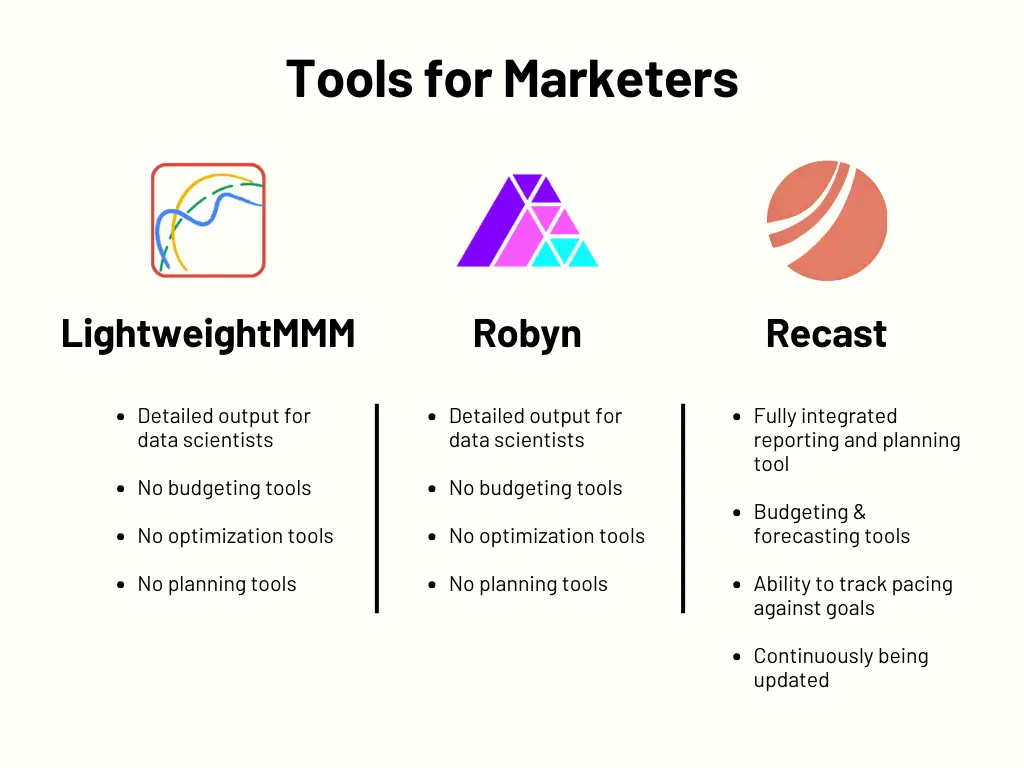
Marketers have specific requirements around how they want to use an MMM to build budgets, do forecasting, and do reporting, and often building those interactive tools is beyond what a data science team wants to maintain.
Recast provides a best-in-class fully integrated reporting and planning tool that not only exposes the results of the MMM but also allows marketers to build budgets, run their own forecasts, and track pacing against goals. Additionally, Recast is continuously updating and improving the tool based on feedback from customers and continuously rolling out those improvements to all users.
Lightweight MMM generates detailed output for data scientists but does not provide tools for budgeting, optimization, and planning for marketers.
Robyn generates detailed output for data scientists but does not provide tools for budgeting, optimization, and planning for marketers.
Modeling
To be useful, MMM models must calculate the incrementality of marketing channels. To actually be able to estimate true incrementality the model’s assumptions must match the reality of how marketing actually works as closely as possible. Of course, no model can capture all of the details of the real world, but when you’re evaluating tools you should think hard about the assumptions the model is making and how that may bias results.
Time Series vs Static Performance
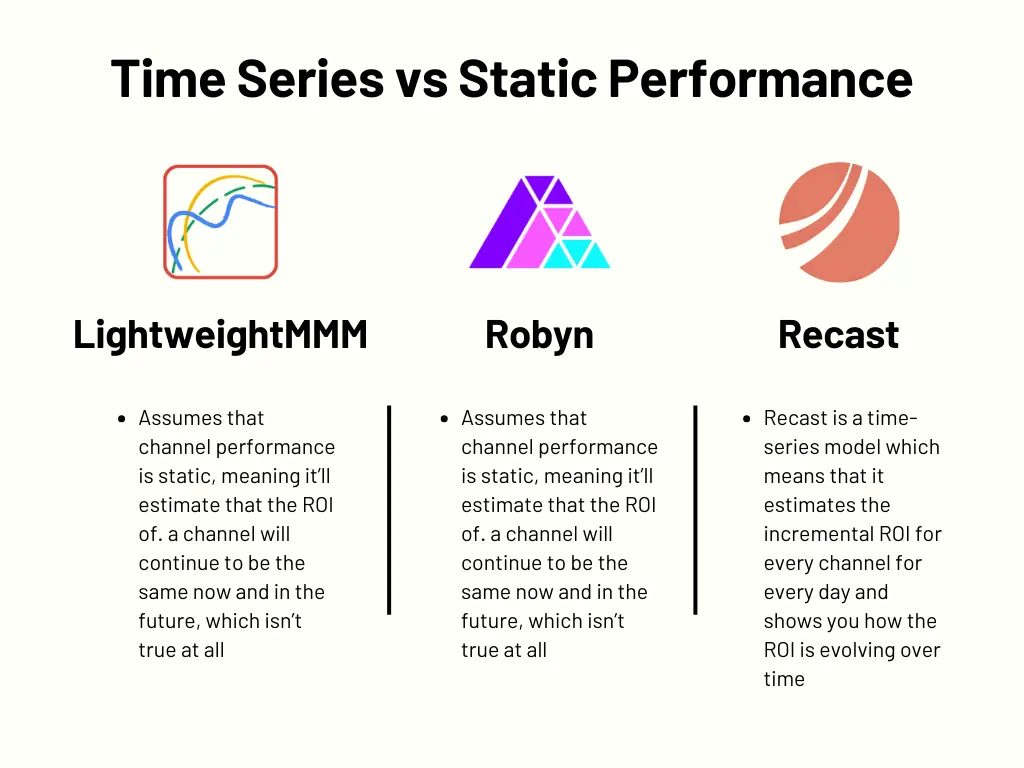
Lightweight MMM assumes that channel performance is static which is to say that if a channel like Facebook has an ROI of 3.5x today it means that it has always had and always will have an ROI of 3.5x. Robyn makes this assumption too.
The problem is that no marketer believes that could possibly be true. Changes like the release of iOS14.5 and ATT can (and did!) change the performance of Facebook marketing. Similarly, seasonality can impact the performance of Facebook’s spend. So can changes in creative or marketing strategy. Recast is a time-series model which means that Recast estimates the incremental ROI for every channel for every day.
As channel performance changes, Recast shows you how the ROI is evolving over time with creative and intrinsic channel changes.
Seasonality
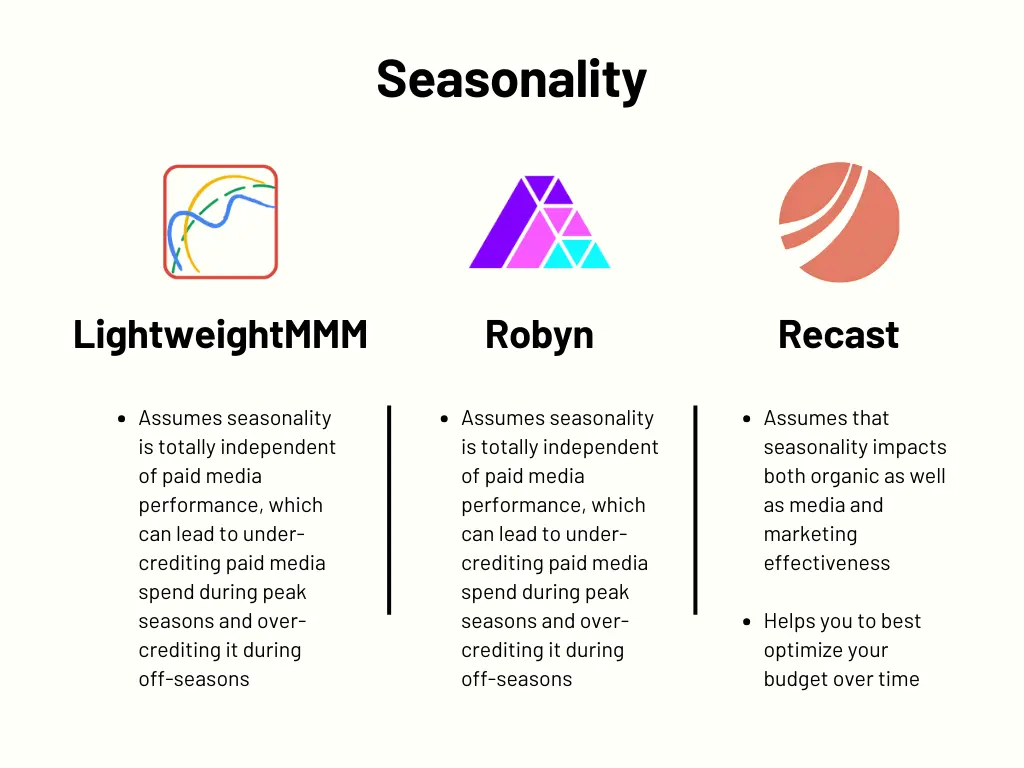
Both Lightweight MMM and Robyn make the assumption that seasonality is totally independent of paid media performance. This means that, for businesses that are highly seasonal (maybe they have a peak sales period around Christmas), they will assume that all of the increase in sales around that time is due to seasonality, and not paid media performance. The result of this is that both models will under-credit paid media spend during peak seasons and over-credit media spend during off-seasons.
Recast assumes that seasonality impacts both the organic part of the business as well as media and marketing effectiveness. If Facebook spend tends to be more performant in the summer than the winter, Recast will capture that underlying pattern and help you understand how you should optimize your marketing budget over time to get the best returns.
Promotional Activities
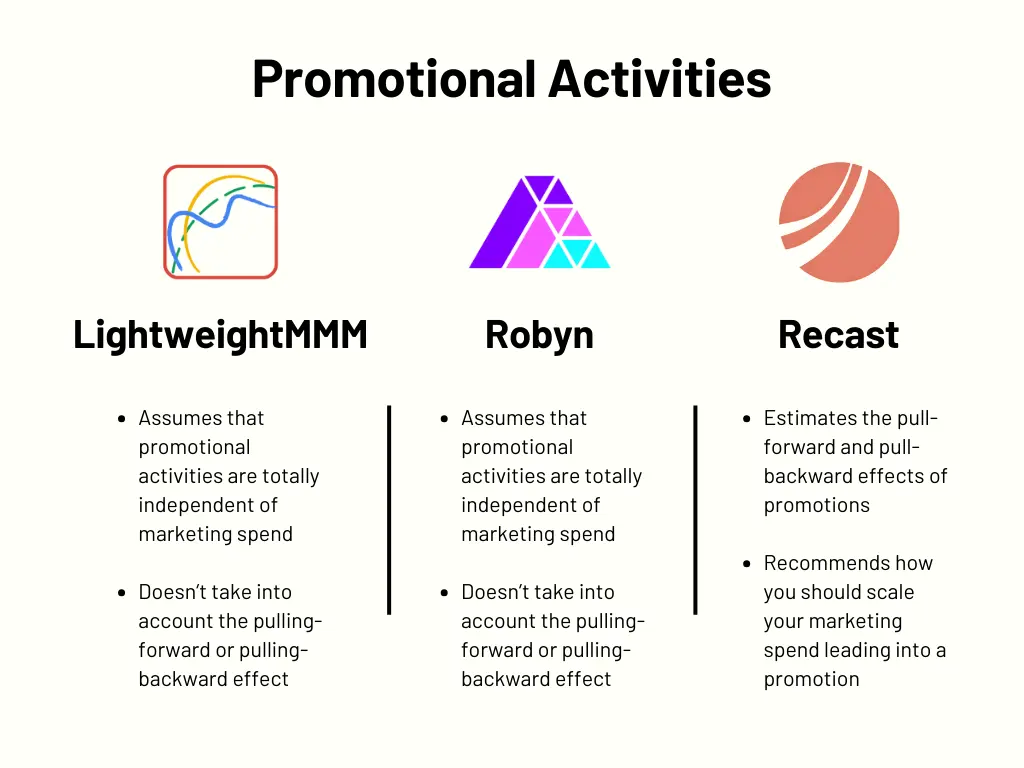
Again, both Lightweight MMM and Robyn assume that promotional activities are totally independent of marketing spend. So if you run a 20% off sale for Memorial Day and have a spike in sales, they will attribute all of that spike to the Memorial Day promotion and none of it to your marketing spend leading up to the promotion. Additionally, they will assume that all of the sales during that “spike” of sales are purely incremental instead of considering the case where the promotion is pulling forward or pulling backward sales that you might have gotten anyway.
Recast actually estimates both the pull-forward and pull-backward effects of promotions (leading to the post-promotion hangover many are familiar with) as well as how those promotional events interact with marketing spend. Recast can recommend how you should scale your marketing spend leading into a promotion in order to maximize returns.
Demand Capture Channels
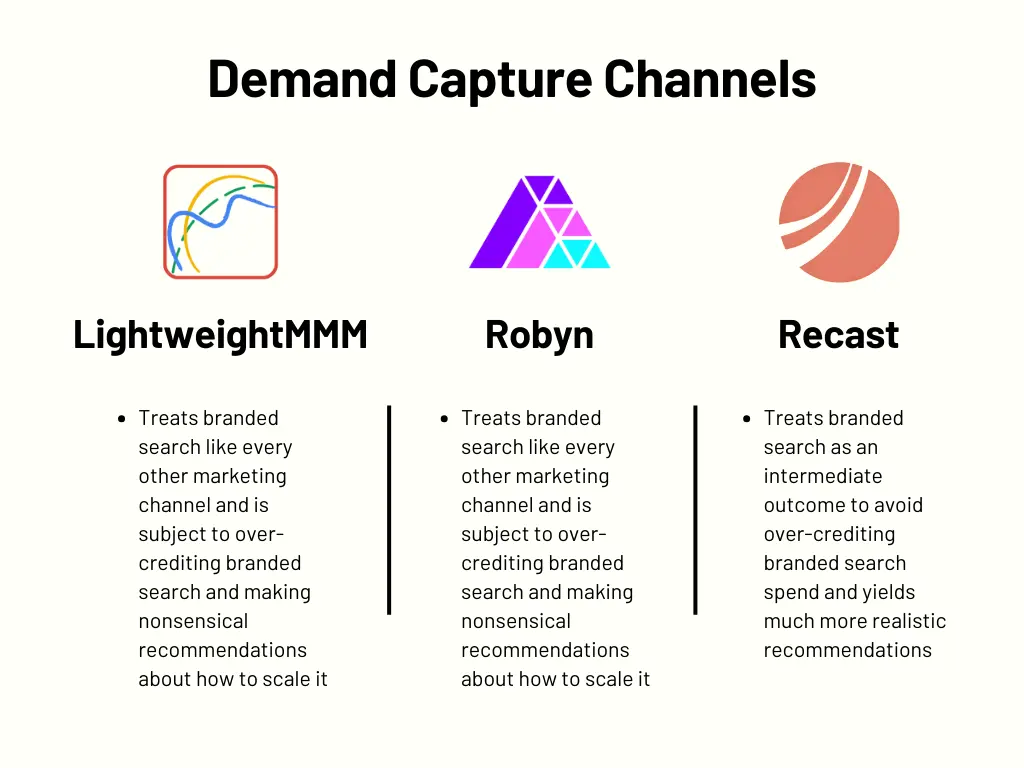
Certain channels like “branded search” do not work the same way as other marketing channels. Most brands cannot choose to simply scale up their branded search spend but instead are reliant on first generating more branded searches that they can then capture. Additionally, branded search activity tends to be highly correlated with sales so it’s easy for less sophisticated models to over-credit branded search returns.
Lightweight MMM and Robyn treat branded search just like every other marketing channel and are subject to over-crediting branded search and making nonsensical recommendations about how to scale into that channel.
Recast treats branded search as an intermediate outcome which is to say that Recast simultaneously models how other marketing activity drives branded search as well as how branded search subsequently drives incremental revenue. This avoids over-crediting branded search spend and yields much more realistic recommendations.
Incorporating Experimental / Lift Test Results
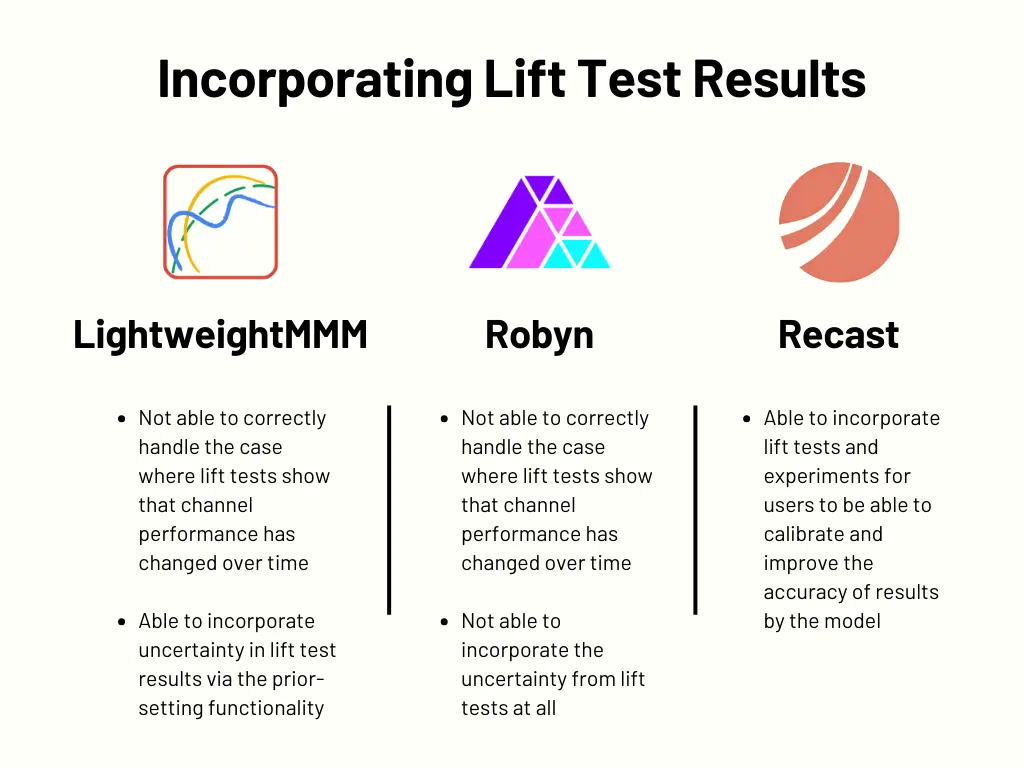
Lift tests and experiments are critically important for calibrating and improving the accuracy of results from an MMM model. However, there are two important features of lift tests that are important to keep in mind when including them in an MMM model:
Lift test results are a snapshot in time. If you run a lift test from January 4 to January 28 then those lift test results apply to that period of time. By the time June rolls around, it’s unclear to what extent those lift test results should still influence our understanding of channel performance.
Lift test results include uncertainty. Depending on the size of the experiment and how well-powered it is, we might have smaller or larger confidence bounds around our estimate of incrementality. A good lift test will have results that look something like “We estimated the channel to have an incremental ROI of 2.4x with a standard error of 0.4”. This means that really incremental ROI is estimated to be somewhere between 1.6x and 3.2x (95% confidence interval).
Correctly incorporating lift test results into an MMM means incorporating both the time-bounded nature of the test as well as the uncertainty into the modeling framework.
Since Lightweight MMM does not allow marketing performance to change over time, it is not able to correctly handle the case where lift tests show that channel performance has changed over time. A lift test from 9 months ago impacts the results just as much as a lift test last week. However, Lightweight MMM is able to incorporate uncertainty in lift test results via the prior-setting functionality.
Since Robyn does not allow marketing performance to change over time either, so it is not able to correctly handle the case where lift tests show that channel performance has changed over time. Additionally, Robyn is only able to incorporate lift tests as point estimates and so is not able to incorporate the uncertainty from lift tests at all.
The Bigger Picture: Recast vs Robyn vs Lightweight MMM – What’s Best?
This blog post is pretty clearly slanted towards Recast. In part, that’s because we’ve invested a lot of time and resources into building a product that we’re proud of, and in part that’s because we truly honestly believe that an open-source DIY MMM is not the right solution for the vast majority of brands.
One reason is that it’s just too easy to get wrong and therefore be led astray. MMM is a case where something is not better than nothing. In fact, nothing is way better than something that’s actually wrong.
Recast is powered by a proprietary statistical model composed of thousands of lines of Stan code and, while much less expensive than legacy MMM solutions, is certainly more expensive than free. We, of course, think that most people should buy instead of build, but understand that might not be realistic for smaller companies. So while this might look heavily biased, a lot of that is coming from the fact that this is a comparison between an advanced proprietary solution and two open-source tools — which will be more bare-bones
Also, most, if not all of the open-source tools required for MMM generate results that might be consumable for data scientists, but are not necessarily ready to be delivered to marketers. Just exporting a CSV file or the default Matplotlib plots that come out of the open-source library is not going to cut it for less technical users.
Getting the results is an important part of the process, but it’s actually just the first step. There are a lot more steps beyond that when it comes to taking those results and making them actually actionable for a marketing team to go use.
In general, if you have the budget to invest in making one of the open-source tools work for you, you have the budget to afford a better off-the-shelf product. And if you don’t have the budget to invest, then using an open-source tool that’s misleading is actually worse than investing that effort in doing things like running better and more focused experiments.