Disclaimer: This blog post was written by an external contributor about approaches for measuring new marketing channels with marketing mix modeling. The approach laid out in this document is not reflective of how Recast approaches modeling new channels. The actual model specification can be found in the technical model specification.
If you’re in the marketing world, you’re probably familiar with marketing mix modeling (MMM), one of the attribution methods popularized since Apple limited tracking in iOS14. MMM is a statistical technique that helps marketers allocate resources across different channels based on past performance. However MMM works based on historical data, which you don’t have when launching a new channel. What this means in practice is that new channels tend to be statistically insignificant. In the case of Meta’s Robyn, the Ridge Regression algorithm ‘shrinks’ the coefficient for a new channel to zero: essentially proclaiming the new channel as worthless. For example take this example from Robyn’s documentation, showing the ‘events’ channel as driving 0% of revenue most of the time: something your events team is likely to disagree with!
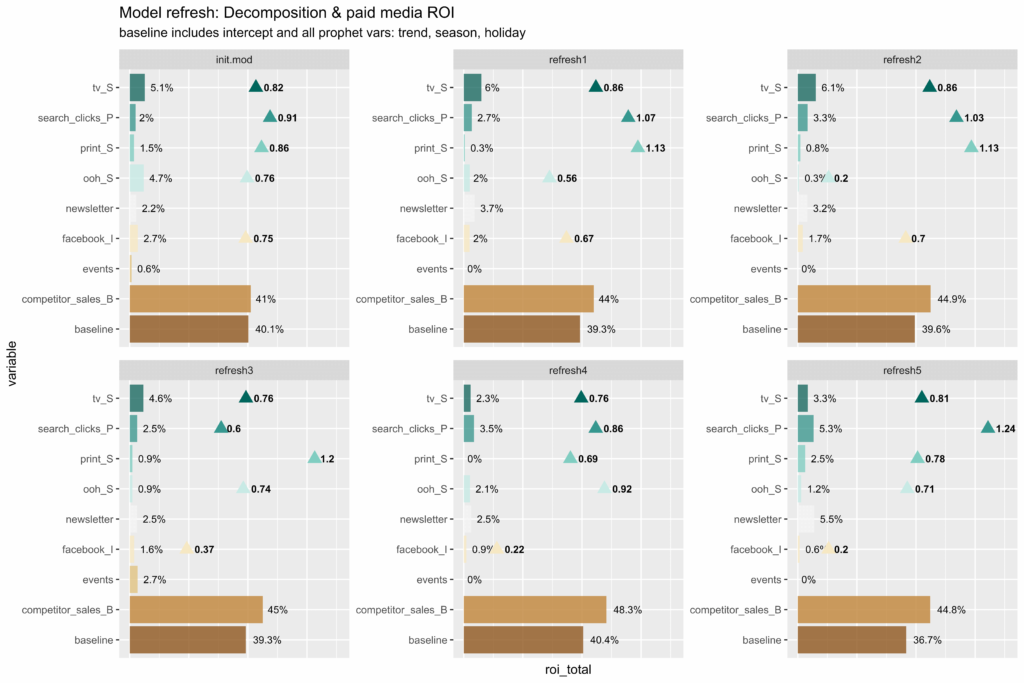
If your attribution model treats new channels as automatically bad, that can lead to sub-optimal resource allocation and over-saturation of existing channels. The ‘new channel problem’ hasn’t been as large an issue historically, as MMM was typically used by large businesses to make budget allocation decisions spanning the course of years. For these businesses the channel mix didn’t change all that often, and when it did, it would be several months to a year before the next modeling exercise, allowing ample time for data collection. Modern consumer brands diving into MMM have a much more dynamic marketing mix, and make budget allocation decisions in real-time with a ‘test-and-learn’ approach, rather than adhering to an annual planning cycle. That has necessitated the development of more modern methods, like Bayesian priors, so that new channels are given a chance to prove themselves.
There are several reasons why measuring new channels is difficult in MMM, from lack of data, to the complexity of modeling, and a lack of understanding of how a new channel works. We’ll cover each of these issues, highlighting how we solve them with our automated MMM solution at Recast.
1. Lack of data
The main reason why MMM may not be effective for new channels is that there is often a lack of data available when these channels are first launched. Typically marketers will “test in” to a new channel, starting with only a few percentage points of their mix. But this may be much less than the day-to-day percentage variation in sales. It also takes time for any spend to realize its full effect (due to adstocking). These two facts together mean that it can take some time to gather enough signal. If the day you launch a new channel there happens to be a spike in sales for some other reason, the model may mistakenly assign too much contribution to the new channel. Or vice versa, if you launch in a period of low demand, the model might blame that on the new channel, and claim it somehow lowered sales!
At Recast we use a Bayesian model, which allows us to set ‘priors’ for each variable in the model based on domain expertise. For example you could make certain assumptions about how media channels perform – they positively impact sales, their impact is lagged over time, and they saturate at high spend levels – that give us a reasonable basis for modeling any channel accurately. From this starting point, the model increases in accuracy over time as we gather more data on the new channel. This decreases the surface area of possible values the parameters in the model could take on, which greatly decreases the risk of the model giving you an implausible result.
2. Lack of understanding
The people who build marketing mix models are usually not the same people who actually run campaigns day to day. They’re usually experts in statistics or data science, who work across a broad range of tasks, potentially not even exclusive to marketing. Therefore they tend not to have the domain expertise to know that TikTok ads are likely to perform similar to Instagram Reels, being both short form video channels with lots of fast-growing under monetized inventory. Lack of domain expertise can lead to obvious avoidable mistakes, which is why MMM vendors insist on so many meetings with various stakeholders in the team. However this can also be a source of bias, with modelers eager to show the results they know their clients want to see.
The way we think about this problem at Recast is to empower the actual marketers running the campaign to use their domain expertise. In our system it’s possible to set the Bayesian priors for a new channel based on an existing one, so the model has the best possible starting point. For example if you launch TikTok ads today, we can start it off with a similar model to your Instagram Reels campaigns, allowing us to make reasonable predictions about performance from the outset. Over time the model will deviate from the initial priors as more data helps it decrease uncertainty about how this new channel actually works. Even if the initial assumptions were biased, it started us off in the right neighborhood, and from there we let the data guide us.
3. Accounting for Optimization
Even if you successfully model your new channel, how quickly do the results go ‘stale’? For older more established channels your results are relatively stable over time, but for new channels that assumption rarely holds. It’s common to see an order of magnitude increase in performance as you learn the channel, testing new targeting options and creative concepts until you find what works. Most marketing mix models handle this poorly, because they make the indefensible assumption that channel performance is static over time! Meaning when it calculates the ROI of a channel it assumes the channel had that ROI over the entire modeling period. Making that assumption dismisses all of the hard work your team has done over the past few months or years to optimize channel performance.
The way to solve this is a relatively new contribution to MMM, called Time-Varying Coefficients. The key to this technique is again a Bayesian model, which allows you more flexibility on the assumptions your model makes. In a Time-Varying model, each channel doesn’t have just one coefficient for the whole modeling period, it has one for every day or week! This is made possible by assuming today’s performance will look similar to yesterday’s performance, but might deviate if we have enough certainty from the data. Over time this allows the channel’s ROI to drift up or down dynamically as the channel is optimized or deteriorates.
4. Expensive & Time-Consuming
One of the drawbacks of marketing mix modeling is that it can be quite time-consuming. It takes weeks to compile all of the data and do the necessary data cleaning to get it into the right format for modeling. In addition stakeholders need to be consulted across the business, to absorb domain knowledge and understand how the model will be used to make decisions. Finally the model has to be built and then tested, before making changes to improve accuracy and explain implausible results. This process can take a significant amount of time and resources and usually needs to involve one or many technical people like statisticians, data scientists, and software engineers. The costs can add up, which is why traditionally most companies only did MMM once per year.
We’ve found that the best way to make MMM more economically viable is to amortize the cost of building a model over time, by automating each stage of the process as much as possible. That way when the model is built, you don’t need to redo all the work again next year: you can have it automatically running in the background, updating every week or two with fresh data so you can make better budget allocation decisions in real-time. We have slowly found ways to standardize and automate the data collection process, connecting into marketing platforms and data warehouses via API in order to pull the data automatically. In addition, the work we’ve done on our modeling algorithm leaves us reasonably confident that once it’s running, it can continue to deliver actionable insights with limited human intervention.
5. Interpreting Results
One limitation of marketing mix modeling is that it can be quite complex. This complexity can make it difficult to understand and interpret the results of the analysis. This is the primary reason why marketing mix modeling is not more widely used. Though the release of open source libraries by Meta, Google, and Uber have helped make the marketing community aware of MMM, there’s still a lot of work to be done to educate marketers on the technique, and abstract away a lot of that complexity behind software like Recast, which empowers marketers to make budget allocation decisions without needing a data science team or statistics training.
We’ve found the key to making MMMs actionable is in the end-game, after the model is built. Most vendors ignore this stage, as they are often just relieved to have actually delivered a working model. At Recast the key tool in our arsenal is our budget optimizer, which lets marketers simulate different budget allocation scenarios and see what marketing mix would give them the best chance of hitting their targets. Most practitioners enjoy solving statistics problems, but it’s easy to forget that math is just a means to an end. Giving marketers the ability to make accurate forecasts about the future is what MMM is for, and it must empower them to make better decisions or the entire exercise was a waste.
Conclusion
Marketing mix modeling doesn’t handle new channels well out of the box. This is because new channels don’t have enough data, MMM practitioners don’t know much about how they work, and the standard MMM algorithms don’t account for changing performance over time. Compounding these issues are the facts that MMM is complex and can be time-consuming and expensive work. Even if you make it to the end of the process, a model is useless if it doesn’t inspire marketers to take action.
Thankfully MMM is being upgraded by new vendors like Recast, and modern consumer brands who demand more insight into new channel performance. Using Bayesian methods to set priors is key to getting the flexibility needed to account for new channels in your model. As well as modeling new channels based on the performance of existing channels, priors can also be utilized to allow channel performance to vary over time. Once you have a system like this in production, with data pulled in and cleaned automatically, MMM can be a viable option for automatically reporting on the effectiveness of any new channels you plan to launch.