In July 2022, the American Data Privacy and Protection Act (ADPPA) became the first bicameral and bipartisan online privacy bill to be cleared by a House committee. The act seeks to pre-empt the patchwork of state-level privacy laws currently in place in the US, such as the California Consumer Privacy Act (CCPA).
While the bill is still in its infancy — it still has to be cleared by the House and the Senate before ending up on President Biden’s desk — this is the farthest that any such bill on data privacy has come.
The fact that the bill has bipartisan support, and that it cleared the committee by a near unanimous vote of 53-2 means that it is almost certain to be made into law in 2023. The only thing that could change between now and then are the exact provisions of the act.
However, if you’re a business that relies in any way on user-level data to shape your marketing strategy, that’s not much to be hanging on to. We know data privacy legislation has been firming up the world over — GDPR, CCPA, COPPA, and now ADPPA. If this one doesn’t get you, the next one will.
Understanding ADPPA
At the heart of the American Data Privacy and Protection Act are four principles:
1. Ownership — The user is the owner of their personal data, and businesses must treat user data the same way they would treat an individual’s personal property.
2. Data Minimization — Businesses must commit to collecting as little user-level data as is needed.
3. Transparency — Businesses must clearly communicate to users when their data is being collected, and offer them the option of opting out of such data collection.
4. Responsibility — Once user data has been collected, businesses must use it with the highest levels of safety, security, and responsibility.
In a nutshell, the ADPPA prohibits businesses from collecting “covered data”, unless it is for one of the 17 purposes that the act lists down. These include data collected for the purpose of:
1. Allowing the completion of a transaction or order
2. Developing, maintaining, improving, or repairing a product or service
3. Authenticating users before allowing them access to a product or service
4. Fulfilling a warranty
5. Preventing, detecting, or responding to a security incident
6. Prevention or detection of fraud, harassment, or any illegal activity
7. Complying with legal obligations
8. Preventing death or bodily harm to oneself or to another person
9. Conducting certain kinds of research
10. Recalling a product
11. Delivering communications to the subject which is not advertorial in nature, and which the subject anticipates
12. Facilitating communications
13.Transferring of assets in the event of a merger or acquisition
14. Protecting the security of covered personal data
15. Preventing or responding to certain public safety hazards
16. First party advertising to adults
17. Targeted advertising, except for purposes prohibited under the act
It may seem at first sight, that targeted advertising, and first-party advertising appear to have sneaked in through the backdoor at number 16 and 17. Not so fast. Covered data is just the first layer of data protection the act enables. There are more restrictions on certain kinds of data which the act defines as “sensitive covered data”, which we’ll come to in a moment.
Even when businesses do collect covered data for any of the 17 purposes allowed under this act, they may use this data for targeted advertising only if they provide targeted individuals with “clear and conspicuous” means of opting out.
Covered Data, Sensitive Covered Data, and Covered Algorithms in the ADPPA
The ADPPA defines two categories of data which it covers, and to which it applies.These are covered data and sensitive covered data.
Covered Data — This includes any data that can be used to identify an individual, or a device linked to an individual. Not included under covered data are deidentified data, publicly available information, and employee data such as the business contact information of an employee, or data collected from job applicants by entities.
Sensitive Covered Data — Sensitive covered data under ADPPA is any of the following 16 types of data:
1. Government issued IDs such as social security numbers
2. Medical history or healthcare information, future, present, or past
3. Financial information such as bank account numbers, debit or credit cards, bank balance etc.
4. Biometric information
5. Genetic information
6. Precise geolocation
7. Private communication such as emails, texts, etc. or any information that identifies the parties involved in such communication
8. Log-in credentials
9.Information identifying an individual’s sexual behavior
10. Calendar information
11. Intimate photographs or videos
12. Any information about video content selected by an individual collected by an entity that is not a provider of video content services such as a cable network or a streaming video service provider
13. Information about race, color, religion, sexual orientation, or union membership
14. Any information collected while knowing that the individual is a minor
15. Information identifying an individual’s online activities across third-party websites
16. Covered data collected for the purpose of identifying any of the above types of data
Of particular interest to digital marketers here is item number 15, which classifies any information identifying an individual’s online activities over time, and across third-party websites, as sensitive covered data.
What sets sensitive covered data apart from covered data is that businesses are not allowed to use it for first-party marketing, and targeted advertising, nor can they transfer this data in case of mergers, acquisitions, or bankruptcy proceedings. These correspond to permitted uses number 13, 16, and 17 in the list discussed in the previous section. The only cases where the collection and processing of sensitive covered data may be permissible is when it is needed for making available a product or service specifically requested by the user.
Finally, the ADPPA introduces a third term called a “covered algorithm”. This includes any computational process that makes a decision related to covered data. The act requires any large data holder using such an algorithm to conduct an impact assessment of their actions and submit it to the concerned enforcement authority. This means more compliance headaches for firms collecting large amounts of user data.
ADPPA and Digital Marketing
So what does all this mean for marketers? Three things, essentially:
1. Less data
2. More compliance
3. Broken tracking
Studies show that at present, only 31% of the users in the US consent to cookies. This is the scenario when most businesses in the US are not bound by any federal law to conspicuously display options to opt-out. What would happen to cookie consent rates if websites were required to explicitly display an opt out option?
According to a study on 70,000 users from Chile, the cookie consent rate drops down to a mere 5% when options to opt out of targeted advertising are conspicuously displayed.
That then, is the scenario most marketers in the US would be faced with once the ADPPA comes into effect.
That’s not all. The ADPPA specifically prohibits targeted advertising aimed at any individual under the age of 17. This means that the ADPPA casts a wider safety net than existing legislation aimed at protecting minors such as COPPA, which only covers children under the age of 13.
Nor is the ADPPA meant to be a toothless tiger. Among its provisions is a requirement that the Federal Trade Commission (FTC) create a dedicated bureau of privacy (BoP) to oversee compliance.
Users have a right to not only access their data, but also demand the entities collecting such data to delete it. This in turn, creates a whole new set of challenges for businesses — that of data mapping, classification, and discovery.
Organizations can only delete user data if they know where it is stored in the first place. With the maze of data clouds, data lakes, CRMs, and CDPs in which customer data is stored, and through which it is shared with third-parties, servicing a multitude of customer requests to delete their data is a potential nightmare.
The ADPPA uses the term “service provider“, which is analogous to the GDPR’s data processor, and “covered entities“, the equivalent to GDPR’s data controllers. The covered entities under ADPPA are not responsible for any violation by the service providers so long as the entity itself adhered to the ADPPA rules. So you need to make sure that your house is in order.
The solution — decreased reliance on user-level data.
Marketing Mix Modeling for a Post-ADPPA World
Marketing Mix Modeling (MMM) uses econometric techniques such as multivariate regression to factor in the impact of non-market forces on campaign performance, in addition to market forces. This results in richer, more complete, and more privacy-friendly models.
User-level data is just one variable in MMM — great if you have it, but you can do just as well without it.
Attribution models on the other hand are built on very limited data sets that fail to account for the complexity of human decision-making.
For instance, using cookies to reconstruct a consumer journey is based only on the data collected from a customer’s interaction with each touchpoint in the journey. It fails to take into account the fact that there are a large number of unknown variables beyond our control that shape purchase decisions. Even if it could take these into account, attribution modeling simply lacks the raw power to process and make sense of a large number of random events.
MMM not only accounts for a far greater number of variables, it is also capable of assigning probabilistic weights to the several possible outcomes. For instance, suppose that The Umbrella Man Company ran a pay-per-click (PPC) campaign in June and saw a spike in sales. Attribution models will tell the marketing team at The Umbrella Man Company which of the touchpoints in the consumer’s journey — from the point at which they searched online for an umbrella till the point they landed on www.theumbrellamancompany.com — contributed to the eventual sale.
Of course, the reconstruction of this journey would depend on data collected using third-party cookies and app tracking across devices, websites, surfaces, and platforms, all of which the ADPPA seeks to restrict under its data minimization principle. And any purchases by consumers under the age of 17 wouldn’t even show up in the model, as the ADPPA explicitly forbids ad targeting for this demographic.
MMM, on the other hand, will tell the marketing team that June is the wettest month in the United States, and that sales of umbrellas go through the roof each year in June anyway. It rained a lot so people bought more umbrellas.
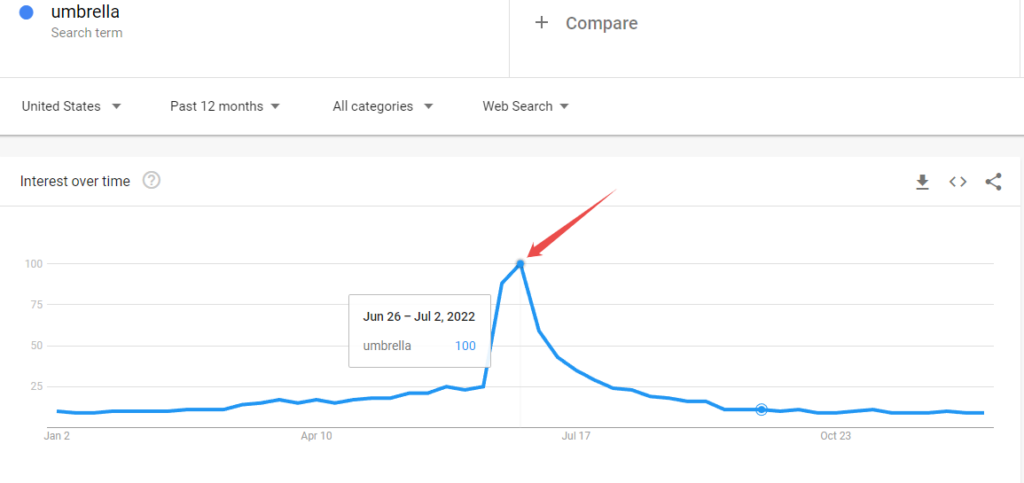
Source: Google Trends trendline for the search term “umbrella” over a 12 month period in the US.
If there are spikes in other times of the year, when you spend a lot on ads, and dips at other times when you cut spend, the differences can be modeled versus seasonality. What you’re left with is a breakdown by channel of what they contributed to topline sales, and an estimate for what will happen if you continue to spend at higher or lower levels.
The best part? You don’t need to collect user-level data for any of this. Of course, this is a simple example, and marketing mix models can run into tens or even hundreds of variables, if you’re using more advanced techniques like Bayesian MMM.
Conclusion
The American Data Privacy and Protection Act is only the latest development in the unfolding of a new data privacy regime that is already setting the norm for how users, states, and the law view data. The European Union’s GDPR, California’s CCPA, Google’s third-party cookie deprecation, and iOS 14’s app tracking transparency features were all precursors to the ADPPA.
While the act may or may not become law in its present form, marketers would do well to assume that the days of unrestricted access to user-level data are as good as over. Which is a blessing in disguise, as there are more efficient econometric models that can deliver richer analytic insights while being future-proof from any data privacy legislation. If you don’t need a user’s data, you don’t have to lose sleep over locating and deleting it.
Get in touch with us to find out how Recast can help you future proof your data modeling. Or if you’re only interested in learning more about Bayesian Marketing Mix Modeling, check out our tutorial here.