In today’s rapidly evolving retail environment, it can be a real challenge for omnichannel brands to measure the incremental impact of their marketing efforts. The modern customer journey spans multiple touchpoints — a consumer might browse for a product category online, see a TV ad, get driven to an owned/D2C experience, do more research in-store, and then ultimately buy on a 3P marketplace like Amazon. Journeys like this make it incredibly difficult to tie marketing spend in a given channel to incremental sales.
In the face of this complexity, there are a few important problems brands need to solve for:
- Understanding how paid marketing activities drive total omnichannel performance.
- Optimizing marketing spend across distribution endpoints, from Retail/Wholesale, to D2C, to 3P Ecomm, and beyond.
This article focuses on how marketing mix modeling (MMM) can provide a holistic approach for omnichannel retailers to navigate these challenges.
The Challenge of Measurement in Omnichannel Retail
Many omnichannel brands rely on traditional, digitally native measurement techniques to optimize budgets across their marketing portfolio. These techniques, such as digital tracking (sometimes just called “attribution”), often fall short in providing complex brands a complete picture of marketing effectiveness.
Pain points primarily arise from the inability to attribute sales across online and offline channels. This can look like:
- Disjointed Data Silos: Digital and offline media data are often stored and analyzed separately. This fragmentation makes it difficult to determine which channels are truly driving results.
- Overlap in Reporting: Marketing teams frequently report on metrics specific to individual channels, such as digital ads or in-store promotions, without a clear way to measure the overlap between online and offline efforts. This often leads to “double counting” across different platforms.
- Incomplete View of the Customer Journey: With digital-tracking-based attribution, many interactions between channels are missed, such as when a customer views a product on their phone after seeing a TV ad, but ultimately makes their purchase in a physical store.
- Attributable ≠ Incremental: With most digital tracking systems, incrementality — the notion that not all marketing touchpoints are driving net new behaviors beyond an organic demand baseline — is a distant afterthought. So, while a channel manager may care about their CPA and ROAS, the metrics that more meaningfully drive business results are Cost per Incremental Action (iCPA) and Incremental Return on Ad Spend (iROAS).
The Role of Marketing Mix Modeling (MMM) for Omnichannel Brands
Marketing mix modeling is a powerful measurement solution for omnichannel brands. It can provide a comprehensive view of how marketing efforts influence incremental sales in total and across all of a brand’s distribution channels.
Rather than focusing on digital interactions, MMMs use top-down statistical modeling techniques on aggregate data to identify the incremental return on investment of every channel in a media mix — whether digital, offline, or hybrid media.
These techniques provide a few key benefits for omnichannel brands.
Apples-to-Apples Performance Comparison Across Distribution Channels: Digital tracking can only provide attribution for owned distribution channels, but media mix models do not have these same limitations. Because they use top-down modeling techniques on aggregate data, MMMs provide apples-to-apples performance comparisons across owned and un-owned distribution channels. This helps fill in significant attribution gaps that many omnichannel brands struggle with.
Holistic Budget Allocation Across Media Channels: Without a complete understanding of how different media channels influence overall sales, brands risk overspending in low-impact areas or missing opportunities to invest in channels that drive real incremental gains.
Marketing mix models can isolate the incremental contribution of each channel on total sales — in addition to halo effects these channels have on sales in each distribution channel — providing clear insights into where marketing budgets should be allocated for maximum impact.
Maximizing ROI: By understanding the incremental return on ad spend (iROAS) from each media channel, MMMs empower retailers to reallocate their marketing spend toward channels delivering the strongest incremental returns. This data-driven approach allows for smarter investment decisions, ensuring spend is not being wasted and is concentrated in the most performant areas.
Accounting for Time Delays: Not all marketing activities have immediate effects. Marketing mix models can estimate time-lagged effects — often referred to as “shift curves” or “adstock” — which ensures brands can accurately measure both the immediate and long-term impact of their marketing efforts. This allows omnichannel brands to understand the ongoing returns of past marketing efforts and the contributions they’re making to today’s sales performance.
Optimizing for Incremental Sales: The true power of MMM lies in its ability to isolate the incremental impact of each marketing activity. By understanding the incremental effect of their marketing activities, brands can optimize towards media channels that are truly driving new customer actions in their priority distribution channels.
Case Study: Using MMM for Omnichannel Decision-Making
Imagine you’re the VP Marketing at a leading perfume brand. You sell in Target, your D2C website, Amazon, and Sephora. You’re tasked with increasing revenue across not one… not two… but all of these touch points in the upcoming quarter. How can an MMM platform help?
You might use a similar workflow to what’s described below to help set realistic marketing goals, get executive buy-in and start making meaningful optimizations across your marketing mix.
Channel-Level Analysis: You start by digging deep into the channel-level performance insights provided by your MMM, particularly drilling into how ROIs and marginal ROIs (the efficiency of the last dollar spent) are trending over time.
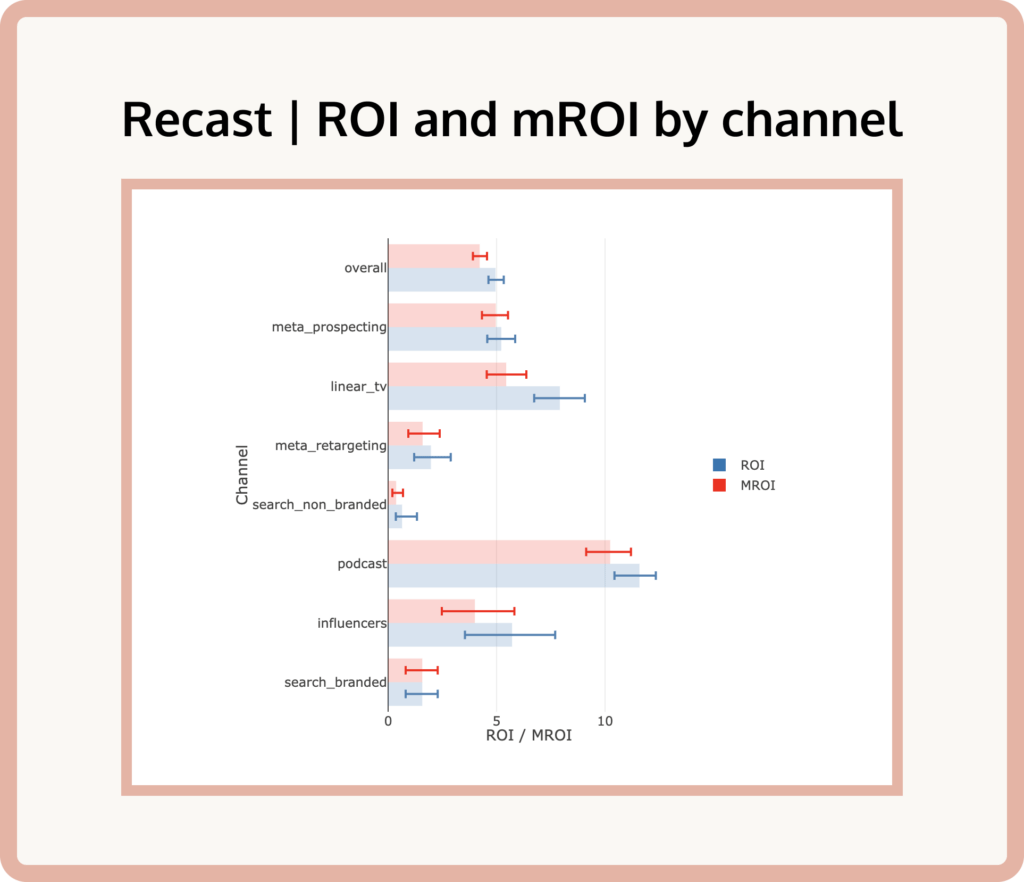
This helps you understand the overall scalability of your current mix as well as the incremental impact of your marketing channels on revenue (in total, and for each of D2C, Amazon, Target and Sephora).
A few points jump out at you:
- Meta campaigns, particularly Retargeting, look great in-platform but their ROI in your D2C MMM model is actually below the average of other channels. Meta Retargeting also isn’t having much impact on Sephora and Target sales.
- By contrast, your D2C MMM model says TikTok and YouTube are highly incremental channels, despite showing fairly low ROIs on in-platform reporting. They’re also driving strong halo effects on Sephora and Target sales according to those models, which are not captured at all by digital tracking.
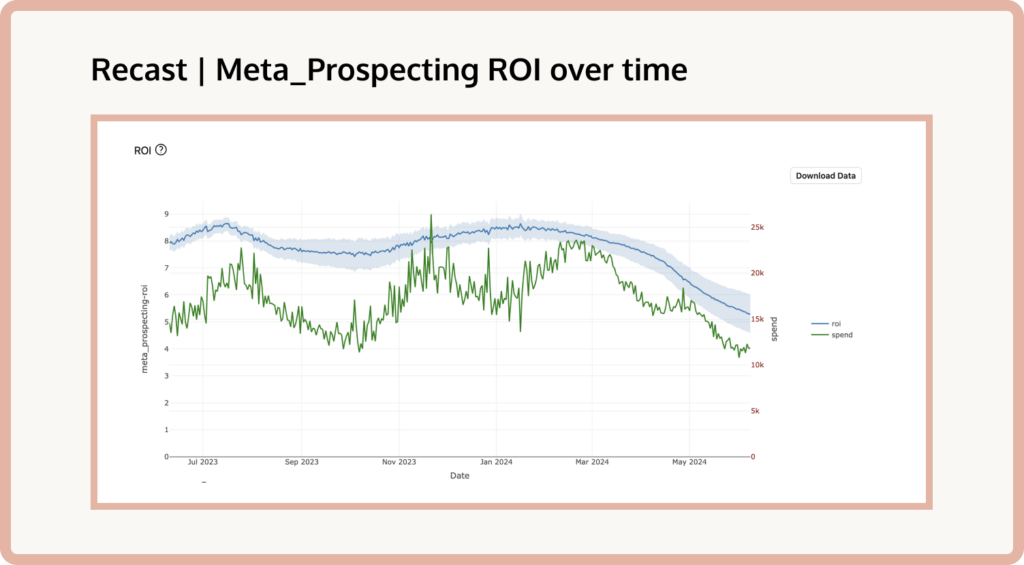
You come away from your analysis with a strong understanding of incrementality across your media mix, as well as the impact that each channel is having across your distribution touch points. You feel confident scaling towards a more ambitious marketing goal, especially given the strong marginal ROIs in YouTube and TikTok.
But the performance of Meta – one of your core marketing channels – gives you some pause. To address this, you decide to find some new sources of incremental revenue. You chart out a testing roadmap that includes:
- Decreasing budget on Meta Retargeting
- Increased budget on TikTok and YouTube
- Two tests of net-new marketing channels: Podcasts and Pinterest – we’ll check on these later in our goal tracking workflow
You move ahead with your recommendation, data in hand, to get executive buy-in for these new tests before the quarter starts.
Setting Goals and Scenario Analyses: Now, you need to update specific revenue forecasts for the upcoming quarter – in total and across each of your distribution nodes (Target, D2C, Amazon, and Sephora).
You also want to understand some of the revenue trade-offs you’ll be making among those distribution nodes as you shift channel budgets.
To do this, you jump from your MMM’s insights tools into its forecasting tools. These let you simulate different budget scenarios:
- What happens if you increase or decrease total marketing budgets?
- What happens if you keep the total budget flat but shift spend between channels?
- What happens if you change total budgets and shift it between channels?
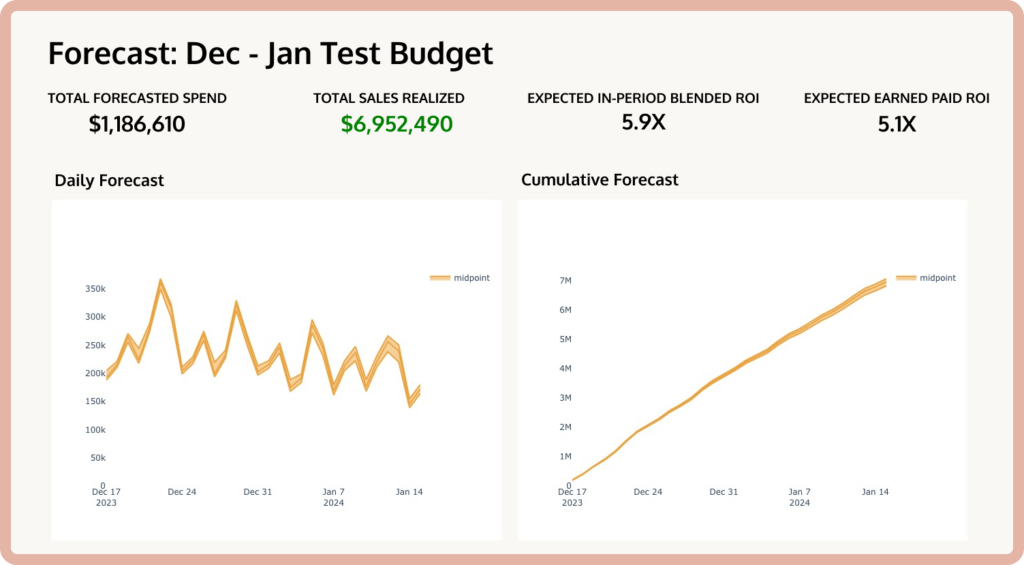
As you run these forecasts, you start to home in on ambitious, but achievable revenue targets. You decide to prioritize an initial channel mix that will have the greatest impact on Sephora revenue – at the cost of some D2C revenue – as this distribution line is most impactful to your brand’s total performance from a profit perspective, and has future strategic upside to get you into additional doors over time.
Ongoing Goal Tracking: Throughout the quarter, you keep track of pacing against your goals and use your MMM to make budget shifts that improve the likelihood of meeting them.
Your MMM receives new data as the quarter progresses, which allows you to assess the impacts that your revised channel mix is having:
- You look at how marginal ROIs on TikTok and YouTube are trending to see if they were as scalable as the MMM first suggested
- You see the incremental impacts of budget shifts on Sephora revenue, your most important distribution line
- You get early reads – albeit with wide confidence intervals – on the incrementality of new Podcast and Pinterest tests
Early in the quarter, if you sense a low likelihood of hitting your revenue goals based on the MMM’s forecasts, you can proactively flag this to leadership. You can also use the platform’s recommendations to make ongoing shifts to channel budgets that improve the likelihood of meeting your most important marketing goals.
Conclusion: Leveraging MMM for Performance and Clarity
For omnichannel brands, traditional marketing measurement techniques often leave gaps in the full picture of marketing performance. By leveraging marketing mix modeling, brands gain the clarity needed to optimize their marketing budgets, focusing on channels that drive the most meaningful and incremental results.
With the ability to account for incremental sales, time delays, and cross-channel effects, MMMs enable smarter marketing investments and improved business performance in an increasingly complex retail landscape.